A Method to Model Conditional Distributions with Normalizing Flows
arxiv(2019)
摘要
In this work, we investigate the use of normalizing flows to model conditional distributions. In particular, we use our proposed method to analyze inverse problems with invertible neural networks by maximizing the posterior likelihood. Our method uses only a single loss and is easy to train. This is an improvement on the previous method that solves similar inverse problems with invertible neural networks but which involves a combination of several loss terms with ad-hoc weighting. In addition, our method provides a natural framework to incorporate conditioning in normalizing flows, and therefore, we can train an invertible network to perform conditional generation. We analyze our method and perform a careful comparison with previous approaches. Simple experiments show the effectiveness of our method, and more comprehensive experimental evaluations are undergoing.
更多查看译文
AI 理解论文
溯源树
样例
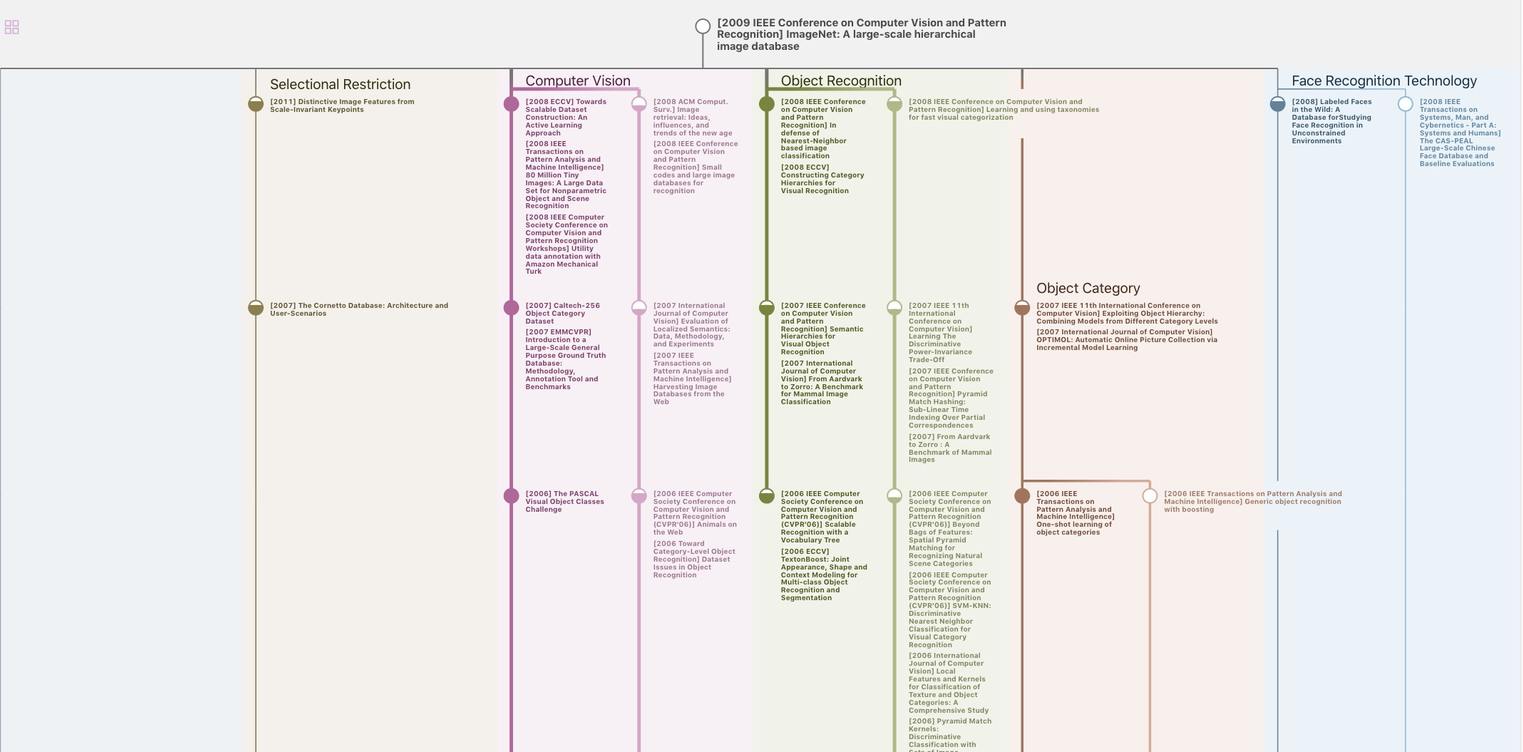
生成溯源树,研究论文发展脉络
Chat Paper
正在生成论文摘要