Prediction of p K a Using Machine Learning Methods with Rooted Topological Torsion Fingerprints: Application to Aliphatic Amines.
JOURNAL OF CHEMICAL INFORMATION AND MODELING(2019)
摘要
The acid-base dissociation constant, pK(a), is a key parameter to define the ionization state of a compound and directly affects its biopharmaceutical profile. In this study, we developed a novel approach for pK(a) prediction using rooted topological torsion fingerprints in combination with five machine learning (ML) methods: random forest, partial least squares, extreme gradient boosting, lasso regression, and support vector regression. With a large and diverse set of 14 499 experimental pK(a) values, pK(a) models were developed for aliphatic amines. The models demonstrated consistently good prediction statistics and were able to generate accurate prospective predictions as validated with an external test set of 726 pK(a) values (RMSE 0.45, MAE 0.33, and R-2 0.84 by the top model). The factors that may affect prediction accuracy and model applicability were carefully assessed. The results demonstrated that rooted topological torsion fingerprints coupled with ML methods provide a promising approach for developing accurate pK(a) prediction models.
更多查看译文
AI 理解论文
溯源树
样例
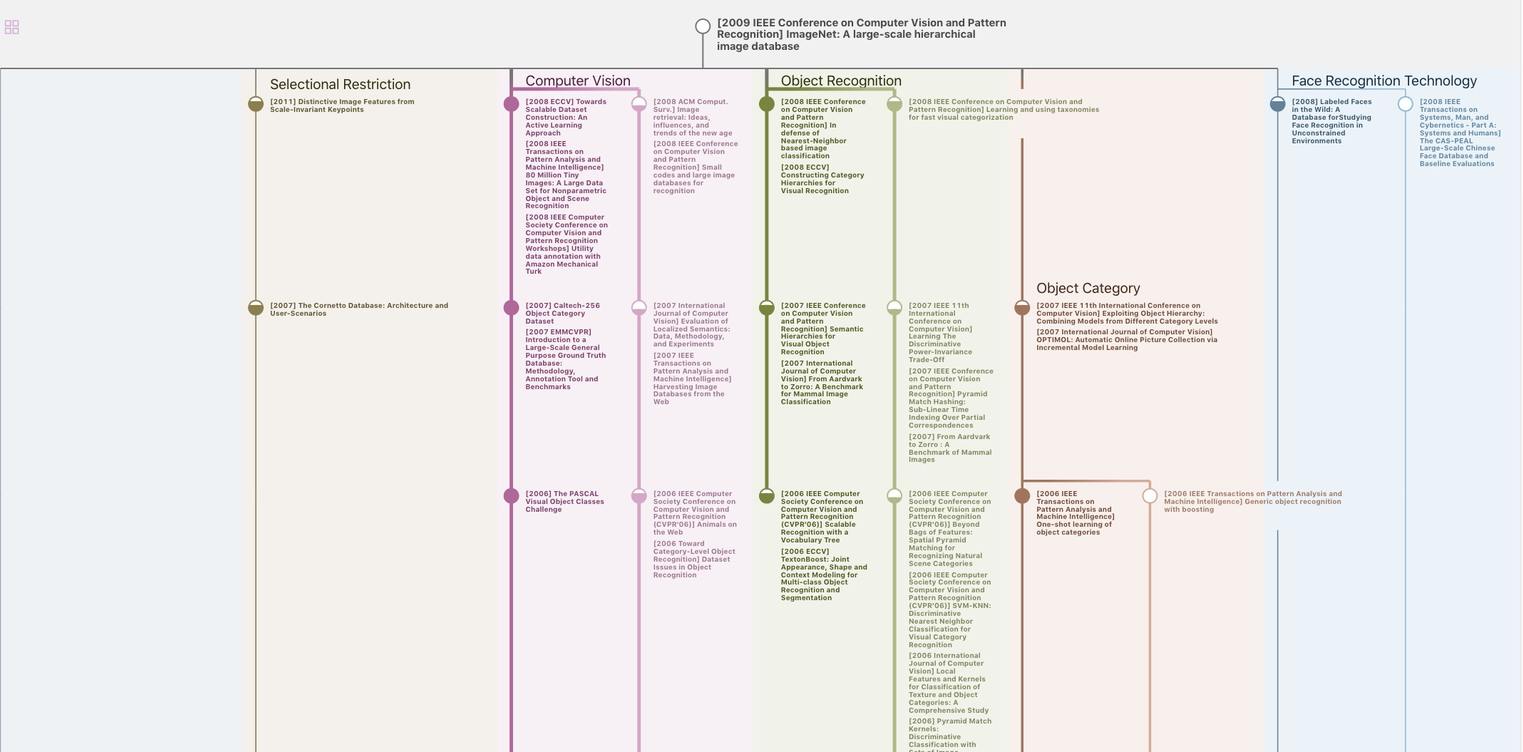
生成溯源树,研究论文发展脉络
Chat Paper
正在生成论文摘要