Weibull Racing Survival Analysis with Competing Events, Left Truncation, and Time-Varying Covariates
JOURNAL OF MACHINE LEARNING RESEARCH(2023)
摘要
We propose Bayesian nonparametric Weibull delegate racing (WDR) to fill the gap in interpretable nonlinear survival analysis with competing events, left truncation, and time -varying covariates. We set a two-phase race among a potentially infinite number of sub -events to model nonlinear covariate effects, which does not rely on transformations or complex functions of the covariates. Using gamma processes, the nonlinear capacity of WDR is parsimonious and data-adaptive. In prediction accuracy, WDR dominates cause -specific Cox and Fine-Gray models and is comparable to random survival forests in the presence of time-invariant covariates. More importantly, WDR can cope with different types of censoring, missing outcomes, left truncation, and time-varying covariates, on which other nonlinear models, such as the random survival forests, Gaussian processes, and deep learning approaches, are largely silent. We develop an efficient MCMC algorithm based on Gibbs sampling. We analyze biomedical data, interpret disease progression affected by covariates, and show the potential of WDR in discovering and diagnosing new diseases.
更多查看译文
关键词
Bayesian nonparametrics,censoring and missing outcomes,interpretable nonlinearity,MCMC
AI 理解论文
溯源树
样例
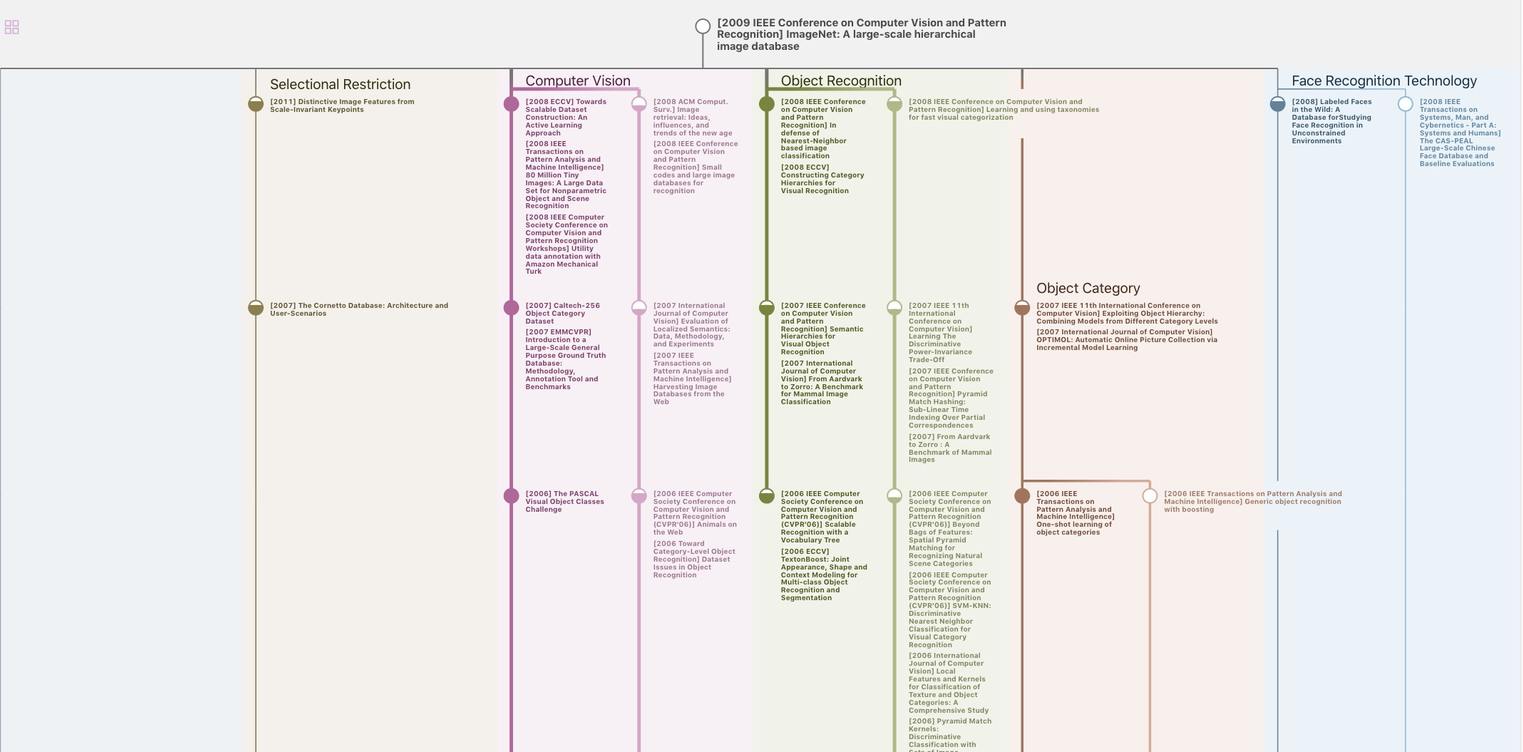
生成溯源树,研究论文发展脉络
Chat Paper
正在生成论文摘要