Nonreversible Jump Algorithms For Bayesian Nested Model Selection
JOURNAL OF COMPUTATIONAL AND GRAPHICAL STATISTICS(2020)
摘要
Nonreversible Markov chain Monte Carlo methods often outperform their reversible counterparts in terms of asymptotic variance of ergodic averages and mixing properties. Lifting the state-space is a generic technique for constructing such samplers. The idea is to think of the random variables we want to generate as position variables and to associate to them direction variables so as to design Markov chains which do not have the diffusive behavior often exhibited by reversible schemes. In this article, we explore the benefits of using such ideas in the context of Bayesian model choice for nested models, a class of models for which the model indicator variable is an ordinal random variable. By lifting this model indicator variable, we obtain nonreversible jump algorithms, a nonreversible version of the popular reversible jump algorithms. This simple algorithmic modification provides samplers which can empirically outperform their reversible counterparts at no extra computational cost. The code to reproduce all experiments is available online (1) .
更多查看译文
关键词
Bayesian statistics, Markov chain Monte Carlo methods, Nonreversible Markov chains, Peskun–, Tierney ordering, Weak convergence
AI 理解论文
溯源树
样例
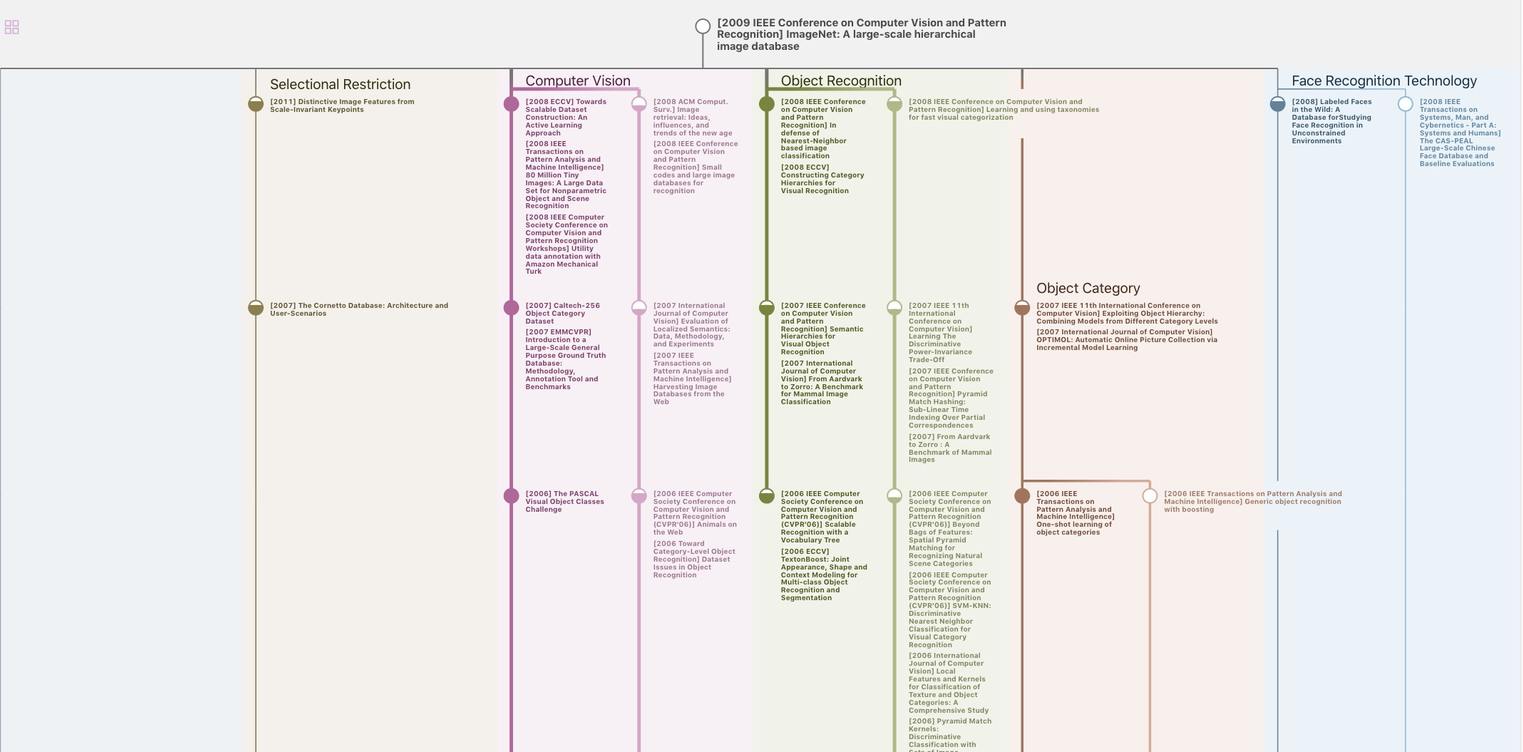
生成溯源树,研究论文发展脉络
Chat Paper
正在生成论文摘要