Cross-Scale Residual Network for Multiple Tasks:Image Super-resolution, Denoising, and Deblocking
arxiv(2019)
摘要
In general, image restoration involves mapping from low quality images to their high-quality counterparts. Such optimal mapping is usually non-linear and learnable by machine learning. Recently, deep convolutional neural networks have proven promising for such learning processing. It is desirable for an image processing network to support well with three vital tasks, namely, super-resolution, denoising, and deblocking. It is commonly recognized that these tasks have strong correlations. Therefore, it is imperative to harness the inter-task correlations. To this end, we propose the cross-scale residual network to exploit scale-related features and the inter-task correlations among the three tasks. The proposed network can extract multiple spatial scale features and establish multiple temporal feature reusage. Our experiments show that the proposed approach outperforms state-of-the-art methods in both quantitative and qualitative evaluations for multiple image restoration tasks.
更多查看译文
AI 理解论文
溯源树
样例
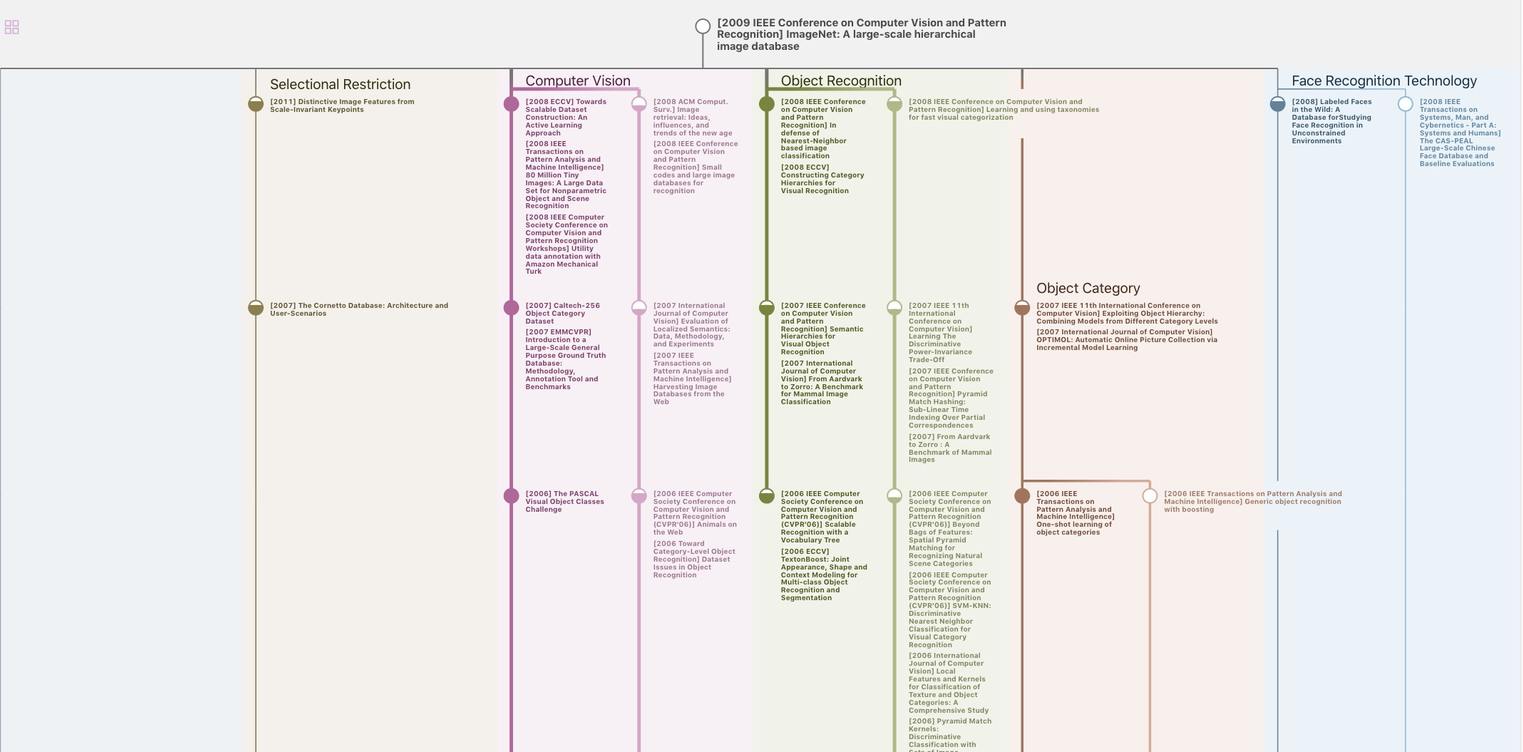
生成溯源树,研究论文发展脉络
Chat Paper
正在生成论文摘要