Guiding mineralization co-culture discovery using Bayesian optimization.
ENVIRONMENTAL SCIENCE & TECHNOLOGY(2019)
摘要
Many disciplines rely on testing combinations of compounds, materials, proteins, or bacterial species to drive scientific discovery. It is time-consuming and expensive to determine experimentally, via trial-and-error or random selection approaches, which of the many possible combinations will lead to desirable outcomes. Hence, there is a pressing need for more rational and efficient experimental design approaches to reduce experimental effort. In this work, we demonstrate the potential of machine learning methods for the in silico selection of promising co-culture combinations in the application of bioaugmentation. We use the example of pollutant removal in drinking water treatment plants, which can be achieved using co-cultures of a specialized pollutant degrader with combinations of bacterial isolates. To reduce the experimental effort needed to discover high-performing combinations, we propose a data-driven experimental design. Based on a dataset of mineralization performance for all pairs of 13 bacterial species co-cultured with MSH1, we built a Gaussian process regression model to predict the Gompertz mineralization parameters of the co-cultures of two and three species, based on the single-strain parameters. We subsequently used this model in a Bayesian optimization scheme to suggest potentially high-performing combinations of bacteria. We achieved good performance with this approach, both for predicting mineralization parameters and for selecting effective co-cultures, despite the limited dataset. As a novel application of Bayesian optimization in bioremediation, this experimental design approach has promising applications for highlighting co-culture combinations for in vitro testing in various settings, to lessen the experimental burden and perform more targeted screenings.
更多查看译文
AI 理解论文
溯源树
样例
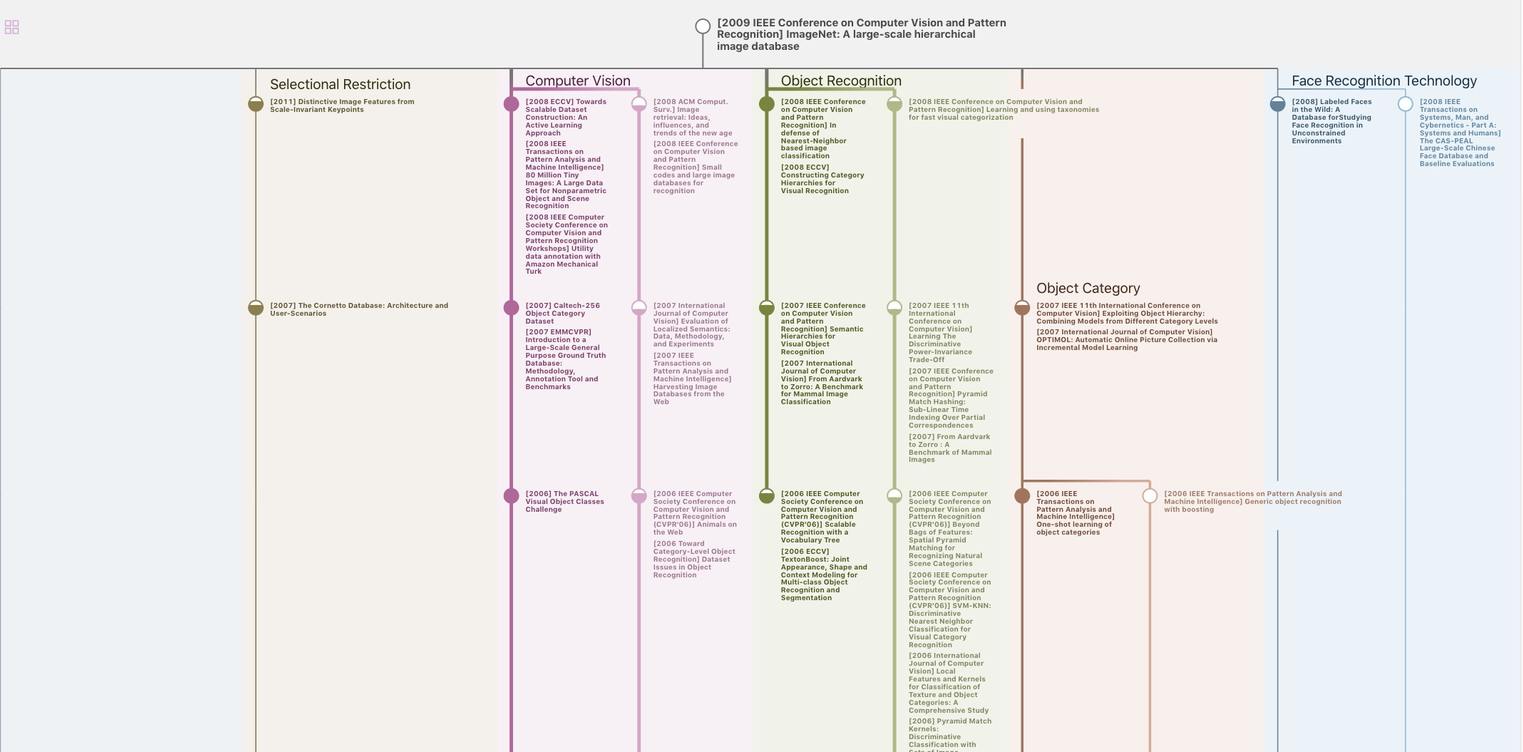
生成溯源树,研究论文发展脉络
Chat Paper
正在生成论文摘要