Question Difficulty Prediction for Multiple Choice Problems in Medical Exams
Proceedings of the 28th ACM International Conference on Information and Knowledge Management(2019)
摘要
In the ITS (Intelligent Tutoring System) services, personalized question recommendation is a critical function in which the key challenge is to predict the difficulty of each question. Given the difficulty of each question, ITS can allocate suitable questions for students with varied knowledge proficiency. Existing approaches mainly relied on expert labeling, which is both subjective and labor intensive. In this paper, we propose a Document enhanced Attention based neural Network(DAN) framework to predict the difficulty of multiple choice problems in medical exams. DAN consists of three major steps: (1) In addition to stem and options, DAN retrieves relevant medical documents to enrich the content of each question; (2) DAN breaks down the question's difficulty into two parts: the hardness for recalling the knowledge assessed by the question and the confusion degree to exclude distractors. For each part, DAN introduces corresponding attention layers to model it; (3) DAN combines two parts of difficulties together to predict the overall difficulty. We collect a real-world data set from one of the largest medical online education websites in China. And the experimental results demonstrate the effectiveness of the proposed framework.
更多查看译文
关键词
attention, document retrieval, educational mining, question difficulty prediction
AI 理解论文
溯源树
样例
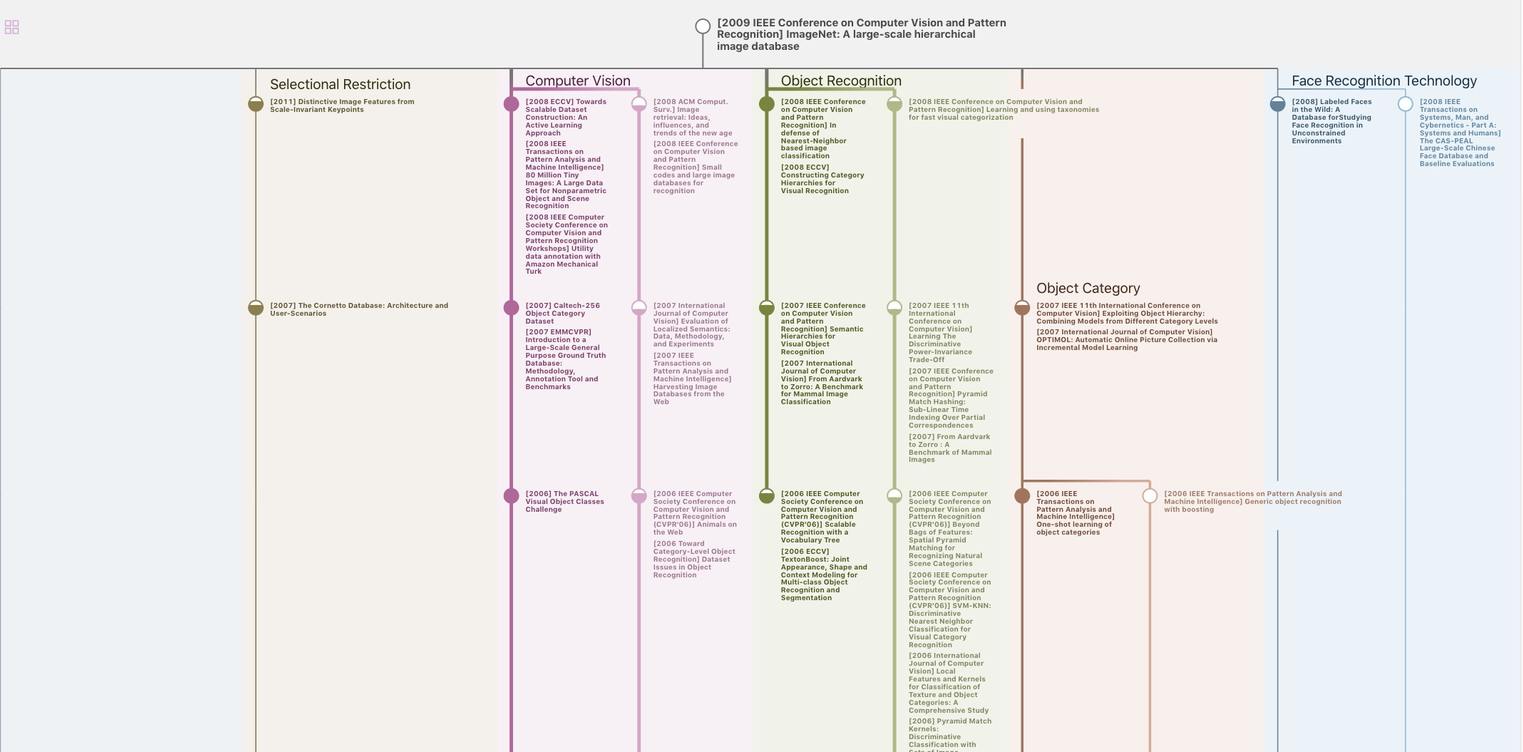
生成溯源树,研究论文发展脉络
Chat Paper
正在生成论文摘要