Query Embedding Learning for Context-based Social Search
Proceedings of the 28th ACM International Conference on Information and Knowledge Management(2019)
摘要
Recommending individuals through keywords is an essential and common search task in online social platforms such as Facebook and LinkedIn. However, it is often that one has only the impression about the desired targets, depicted by labels of social contexts (e.g. gender, interests, skills, visited locations, employment, etc). Assume each user is associated a set of labels, we propose a novel task, Search by Social Contexts (SSC), in online social networks. SSC is a kind of query-based people recommendation, recommending the desired target based on a set of user-specified query labels. We develop the method Social Query Embedding Learning (SQEL) to deal with SSC. SQEL aims to learn the feature representation (i.e., embedding vector) of the query, along with user feature vectors derived from graph embedding, and use the learned query vectors to find the targets via similarity. Experiments conducted on Facebook and Twitter datasets exhibit satisfying accuracy and encourage more advanced efforts on search by social contexts.
更多查看译文
关键词
context query, query embedding, social network, social search
AI 理解论文
溯源树
样例
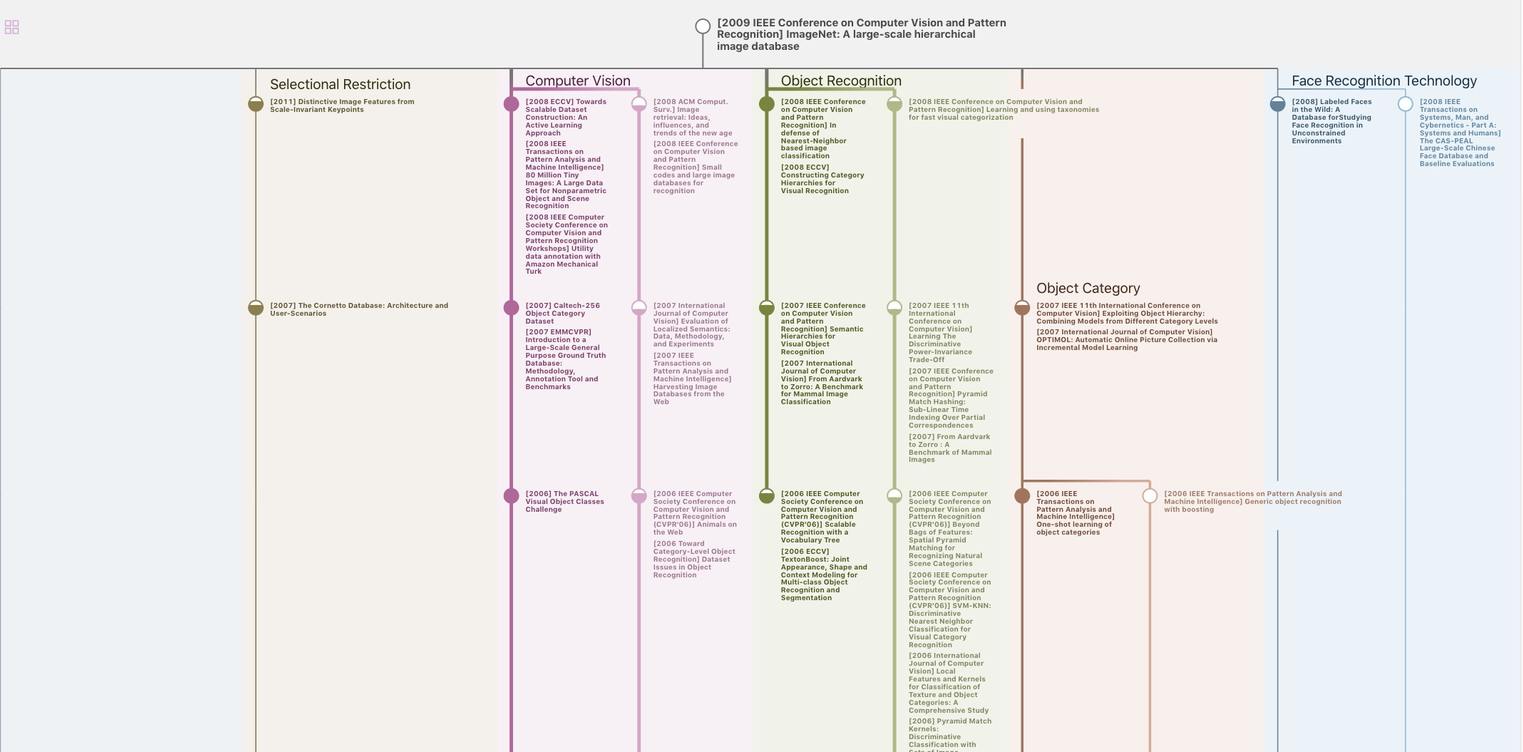
生成溯源树,研究论文发展脉络
Chat Paper
正在生成论文摘要