A Semantics Aware Random Forest for Text Classification
Proceedings of the 28th ACM International Conference on Information and Knowledge Management(2019)
摘要
The Random Forest (RF) classifiers are suitable for dealing with the high dimensional noisy data in text classification. An RF model comprises a set of decision trees each of which is trained using random subsets of features. Given an instance, the prediction by the RF is obtained via majority voting of the predictions of all the trees in the forest. However, different test instances would have different values for the features used in the trees and the trees should contribute differently to the predictions. This diverse contribution of the trees is not considered in traditional RFs. Many approaches have been proposed to model the diverse contributions by selecting a subset of trees for each instance. This paper is among these approaches. It proposes a Semantics Aware Random Forest (SARF) classifier. SARF extracts the features used by trees to generate the predictions and selects a subset of the predictions for which the features are relevant to the predicted classes. We evaluated SARF's classification performance on $30$ real-world text datasets and assessed its competitiveness with state-of-the-art ensemble selection methods. The results demonstrate the superior performance of the proposed approach in textual information retrieval and initiate a new direction of research to utilise interpretability of classifiers.
更多查看译文
关键词
ensemble selection, random forest, semantic explanations
AI 理解论文
溯源树
样例
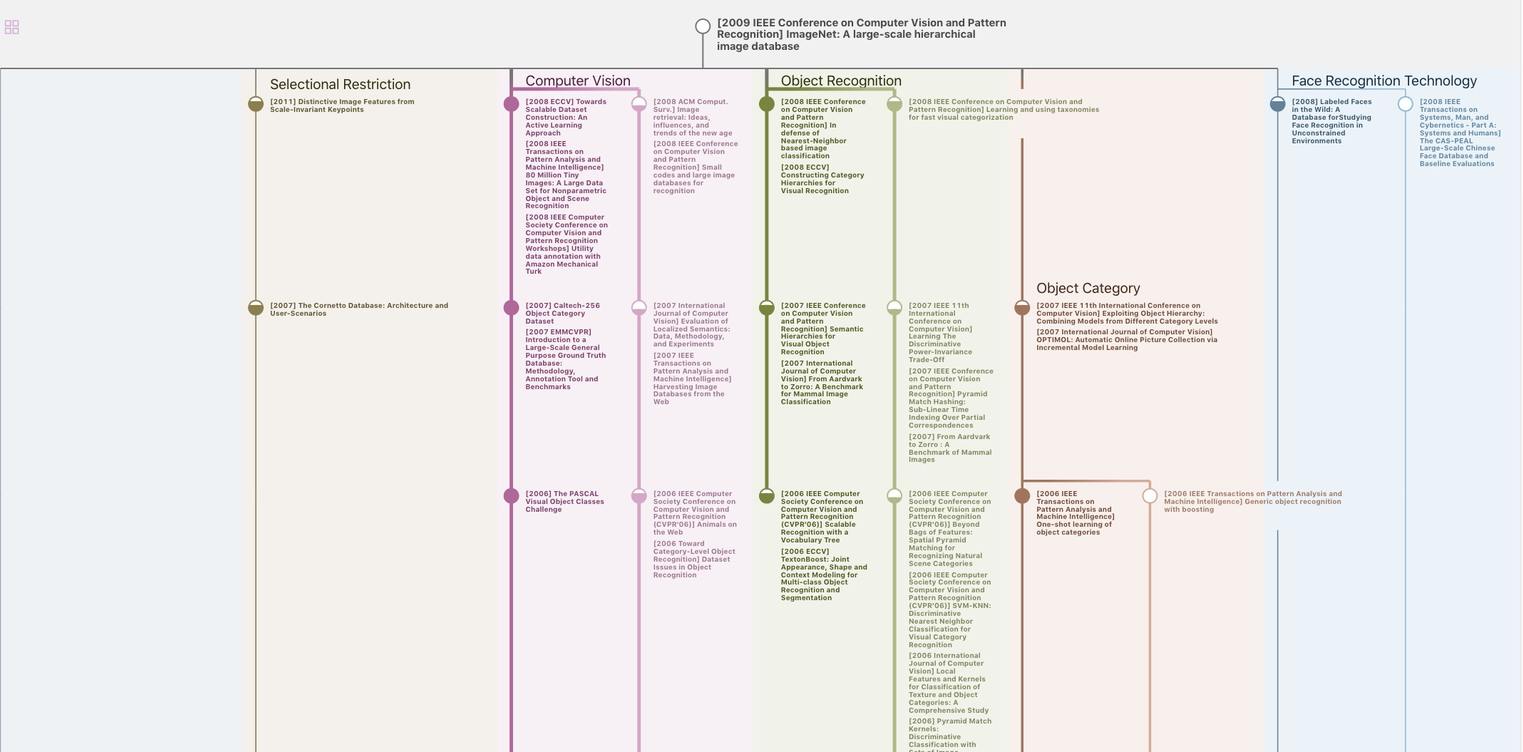
生成溯源树,研究论文发展脉络
Chat Paper
正在生成论文摘要