CRUX: Adaptive Querying for Efficient Crowdsourced Data Extraction
Proceedings of the 28th ACM International Conference on Information and Knowledge Management(2019)
摘要
Crowdsourcing is essential for collecting information about real-world entities. Existing crowdsourced data extraction solutions use fixed, non-adaptive querying strategies that repeatedly ask workers to provide entities from a fixed domain until a desired level of coverage is reached. Unfortunately, such solutions are highly impractical as they yield many duplicate extractions. We design an adaptive querying framework, CRUX, that maximizes the number of extracted entities for a given budget. We show that the problem of budgeted crowdsourced entity extraction is NP-Hard. We leverage two insights to focus our extraction efforts: \em exploiting the structure of the domain of interest, and \em using exclude lists to limit repeated extractions. We develop new statistical tools to reason about the number of new distinct extracted entities of \em additional queries under the presence of little information, and embed them within adaptive algorithms that maximize the distinct extracted entities under budget constraints. We evaluate our techniques on synthetic and real-world datasets, demonstrating an improvement of up to 300% over competing approaches for the same budget.
更多查看译文
关键词
crowdsourcing, extraction, structured domains
AI 理解论文
溯源树
样例
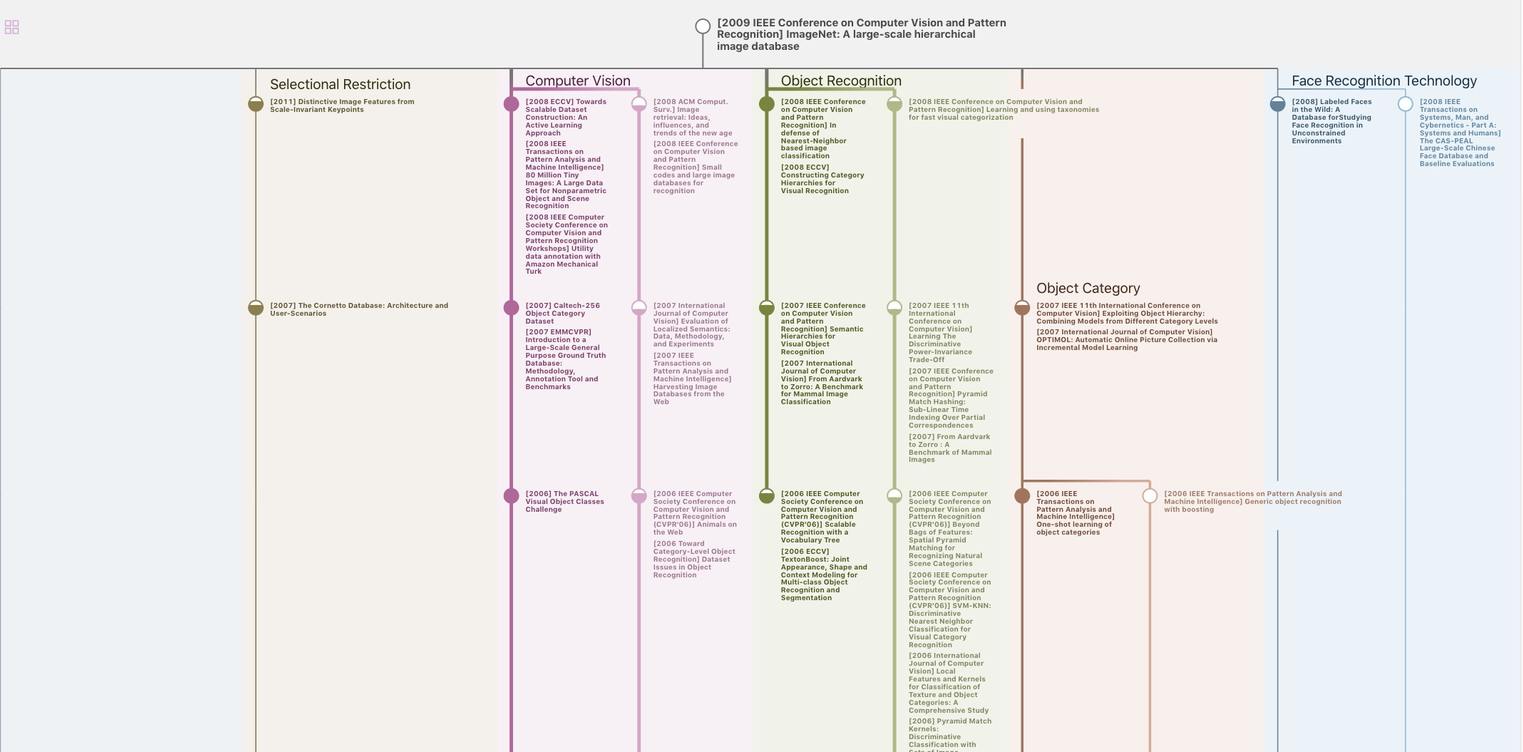
生成溯源树,研究论文发展脉络
Chat Paper
正在生成论文摘要