PRNet: Outdoor Position Recovery for Heterogenous Telco Data by Deep Neural Network
Proceedings of the 28th ACM International Conference on Information and Knowledge Management(2019)
摘要
Recent years have witnessed unprecedented amounts of telecommunication (Telco) data generated by Telco networks. For example, measurement records (MRs) are generated to report the connection states, e.g., received signal strength, between mobile devices and Telco networks. MR data have been widely used to precisely recover outdoor locations of mobile devices for the applications e.g., human mobility, urban planning and traffic forecasting. Existing works using first-order sequence models such as the Hidden Markov Model (HMM) attempt to capture the spatio-temporal locality in underlying mobility patterns for lower localization errors. Such HMM approaches typically assume stable mobility pattern of underlying mobile devices. Yet real MR datasets frequently exhibit heterogeneous mobility patterns due to mixed transportation modes of underlying mobile devices and uneven distribution of the positions associated with MR samples. To address this issue, we propose a deep neural network (DNN)-based position recovery framework, namely PRNet, which can ensemble the power of CNN, sequence model LSTM, and two attention mechanisms to learn local, short- and long-term spatio-temporal dependencies from input MR samples. Extensive evaluation on six datasets collected at three representative areas (core, urban, and suburban areas in Shanghai, China) indicates that PRNet greatly outperforms seven counterparts.
更多查看译文
关键词
deep neurual network, outdoor position recovery, telecommunication big data
AI 理解论文
溯源树
样例
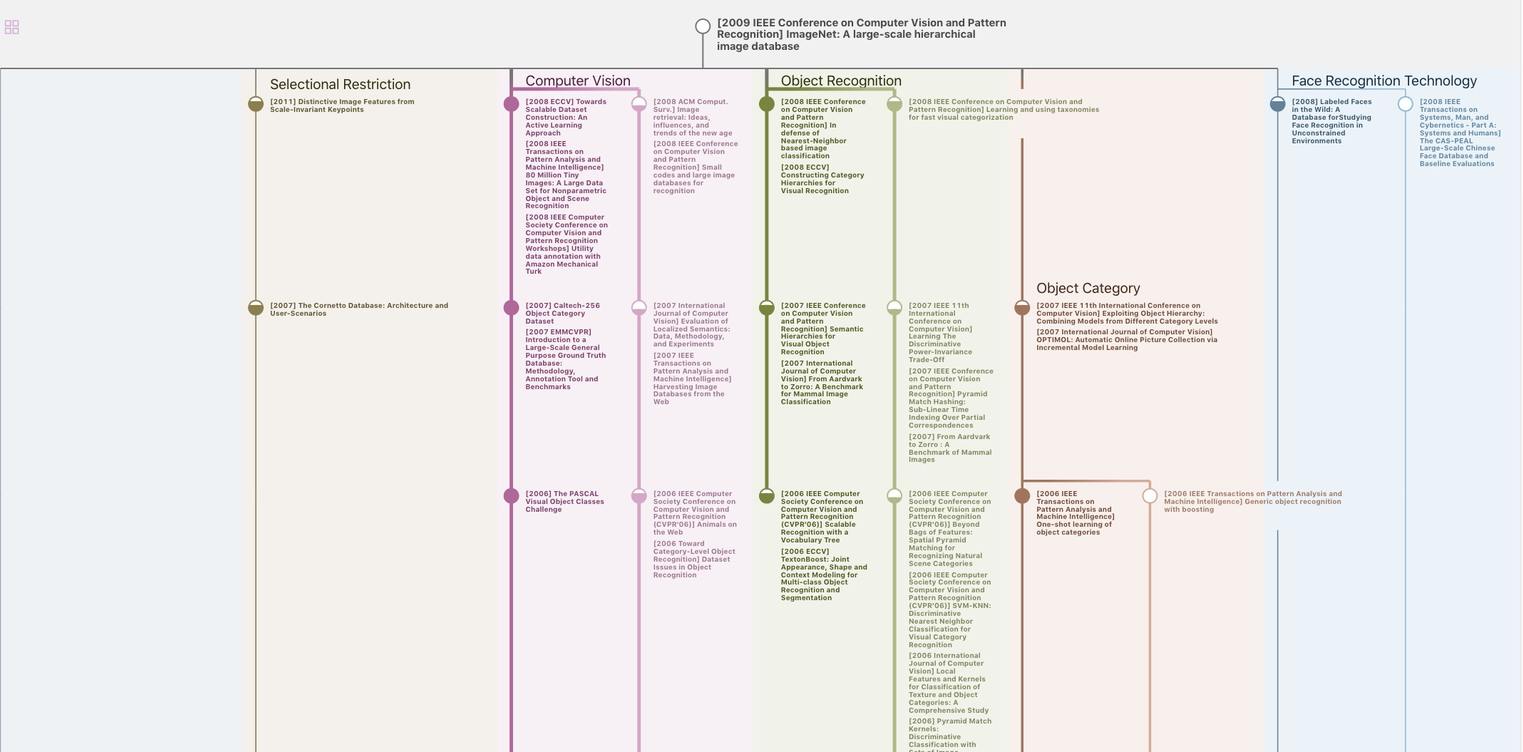
生成溯源树,研究论文发展脉络
Chat Paper
正在生成论文摘要