Deep Dynamic Fusion Network for Traffic Accident Forecasting
Proceedings of the 28th ACM International Conference on Information and Knowledge Management(2019)
摘要
Traffic accident forecasting is a vital part of intelligent transportation systems in urban sensing. However, predicting traffic accidents is not trivial because of two key challenges: i) the complexities of external factors which are presented with heterogeneous data structures; ii) the complex sequential transition regularities exhibited with time-dependent and high-order inter-correlations. To address these challenges, we develop a deep Dynamic Fusion Network framework (DFN), to explore the central theme of improving the ability of deep neural network on modeling heterogeneous external factors in a fully dynamic manner for traffic accident forecasting. Specifically, DFN first develops an integrative architecture, i.e., with the cooperation of a context-aware embedding module and a hierarchical fusion network, to effectively transferring knowledge from different external units for spatial-temporal pattern learning across space and time. After that, we further develop a temporal aggregation neural network layer to automatically capture relevance scores from the temporal dimension. Through extensive experiments on real-world data collected from New York City, we validate the effectiveness of our framework against various competitive methods. Besides, we also provide a qualitative analysis on prediction results to show the model interpretability.
更多查看译文
关键词
deep learning, intelligent transportation, spatial-temporal prediction, traffic accident forecasting
AI 理解论文
溯源树
样例
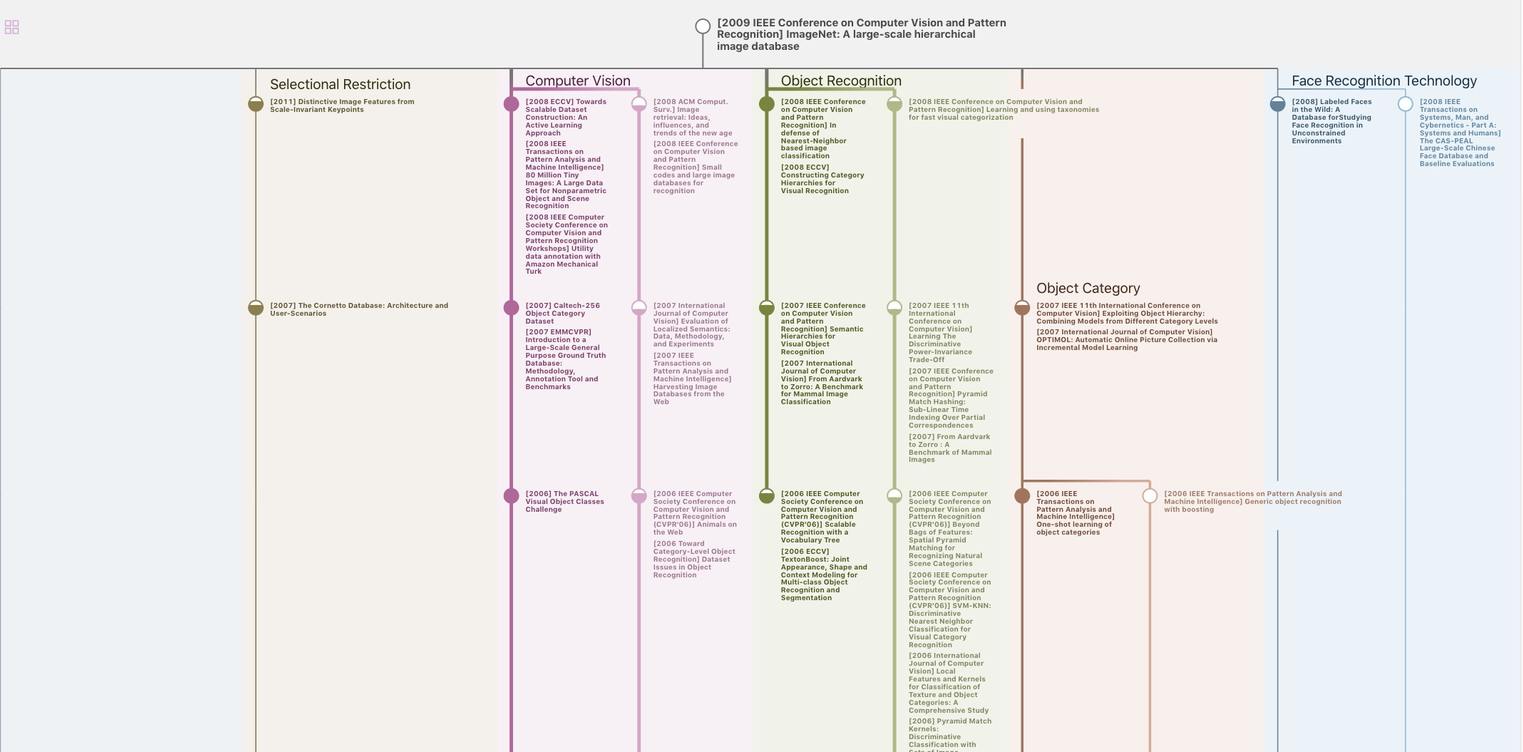
生成溯源树,研究论文发展脉络
Chat Paper
正在生成论文摘要