Learning short-text semantic similarity with word embeddings and external knowledge sources.
Knowledge-Based Systems(2019)
摘要
We present a novel method based on interdependent representations of short texts for determining their degree of semantic similarity. The method represents each short text as two dense vectors: the former is built using the word-to-word similarity based on pre-trained word vectors, the latter is built using the word-to-word similarity based on external sources of knowledge. We also developed a preprocessing algorithm that chains coreferential named entities together and performs word segmentation to preserve the meaning of phrasal verbs and idioms. We evaluated the proposed method on three popular datasets, namely Microsoft Research Paraphrase Corpus, STS2015 and P4PIN, and obtained state-of-the-art results on all three without using prior knowledge of natural language, e.g., part-of-speech tags or parse tree, which indicates the interdependent representations of short text pairs are effective and efficient for semantic textual similarity tasks.
更多查看译文
关键词
Paraphrase identification,Sentence similarity,Short text similarity,Semantic textual similarity
AI 理解论文
溯源树
样例
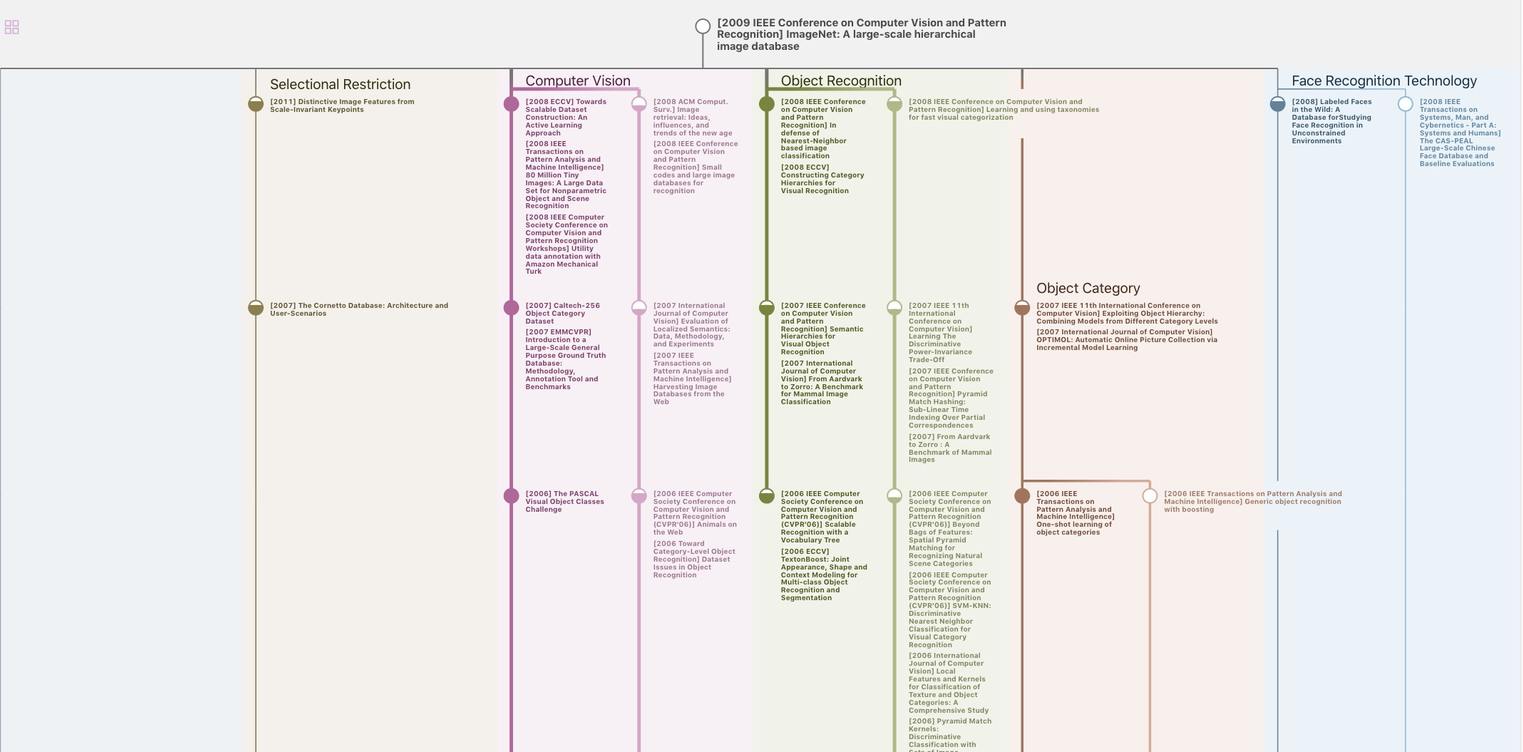
生成溯源树,研究论文发展脉络
Chat Paper
正在生成论文摘要