SID4VAM: A Benchmark Dataset With Synthetic Images for Visual Attention Modeling
2019 IEEE/CVF INTERNATIONAL CONFERENCE ON COMPUTER VISION (ICCV 2019)(2019)
摘要
A benchmark of saliency models performance with a synthetic image dataset is provided. Model performance is evaluated through saliency metrics as well as the influence of model inspiration and consistency with human psychophysics. SID4VAM is composed of 230 synthetic images, with known salient regions. Images were generated with 15 distinct types of low-level features (e.g. orientation, brightness, color, size...) with a target-distractor pop-out type of synthetic patterns. We have used Free-Viewing and Visual Search task instructions and 7 feature contrasts for each feature category. Our study reveals that state-of-the-art Deep Learning saliency models do not perform well with synthetic pattern images, instead, models with Spectral/Fourier inspiration outperform others in saliency metrics and are more consistent with human psychophysical experimentation. This study proposes a new way to evaluate saliency models in the forthcoming literature, accounting for synthetic images with uniquely low-level feature contexts, distinct from previous eye tracking image datasets.
更多查看译文
关键词
SID4VAM,visual attention modeling,saliency metrics,target-distractor pop-out type,synthetic patterns,synthetic pattern images,eye tracking image datasets,visual search task instructions,human psychophysics,free-viewing,deep learning saliency models
AI 理解论文
溯源树
样例
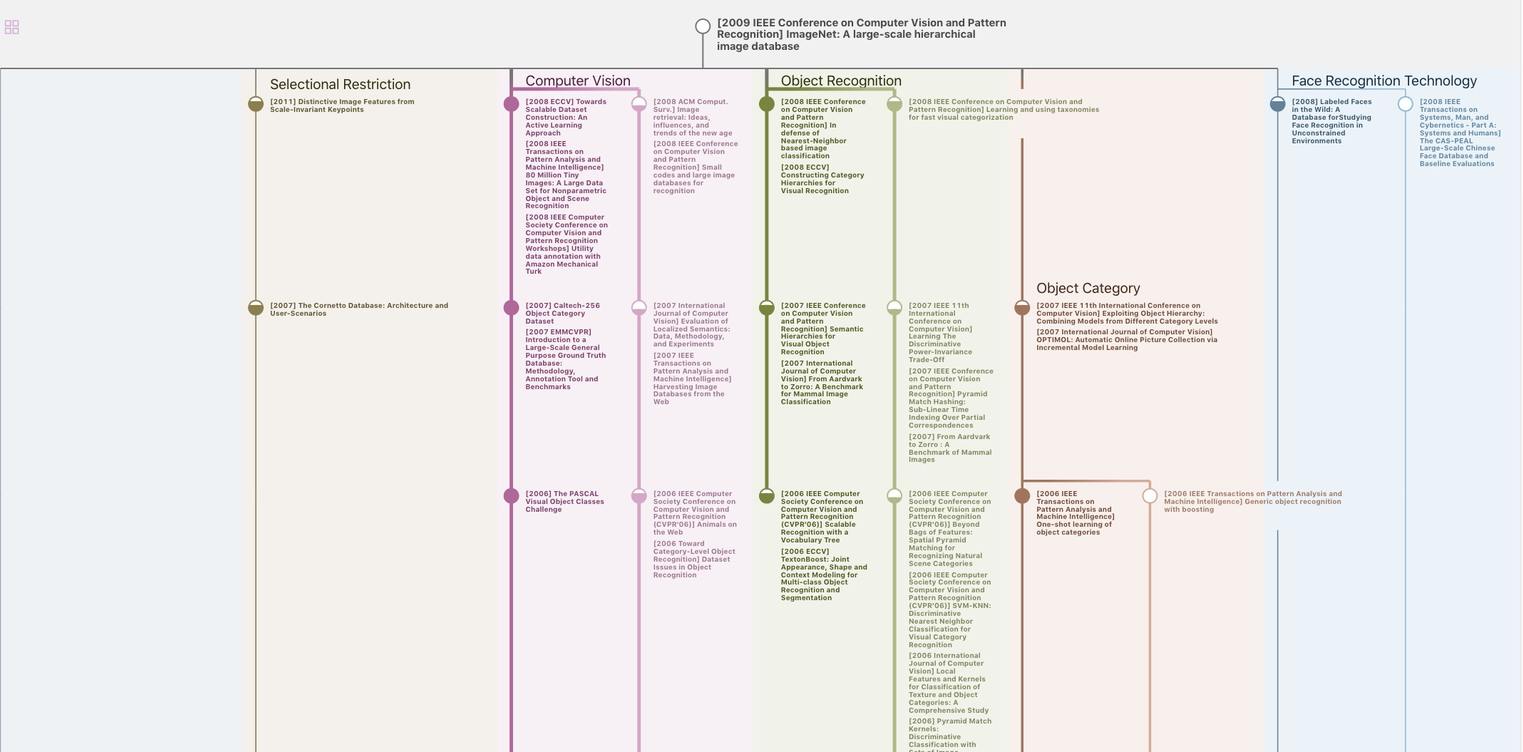
生成溯源树,研究论文发展脉络
Chat Paper
正在生成论文摘要