Foveated Vision For Deepface Recognition
PROGRESS IN PATTERN RECOGNITION, IMAGE ANALYSIS, COMPUTER VISION, AND APPLICATIONS (CIARP 2019)(2019)
摘要
In the last decade deep learning techniques have strongly influenced many aspects of computational vision. Many difficult vision tasks can now be performed by deploying a properly tailored and trained deep network. The enthusiasm for deep learning is unfortunately paired by the present lack of a clear understanding of how they work and why they provide such brilliant performance. The same applies to biometric systems. Deep learning has been successfully applied to several biometric recognition tasks, including face recognition. VGG-face is possibly the first deep convolutional network designed to perform face recognition, obtaining unsurpassed performance at the time it was firstly proposed. Over the last years, several and more complex deep convolutional networks, trained on very large, mainly private, datasets, have been proposed still elevating the performance bar also on quite challenging public databases, such as the Janus IJB-A and IJB-B. Despite of the progress in the development of such networks, and the advance in the learning algorithms, the insight on these networks is still very limited. For this reason, in this paper we analyse a biologically-inspired network based on the HMAX model, not with the aim of pushing the recognition performance further, but to better understand the representation space produced by including the retino-cortical mapping performed by the log-polar image resampling.
更多查看译文
关键词
Face representation space, Hierarchical model 'HMAX', Biological foveated vision
AI 理解论文
溯源树
样例
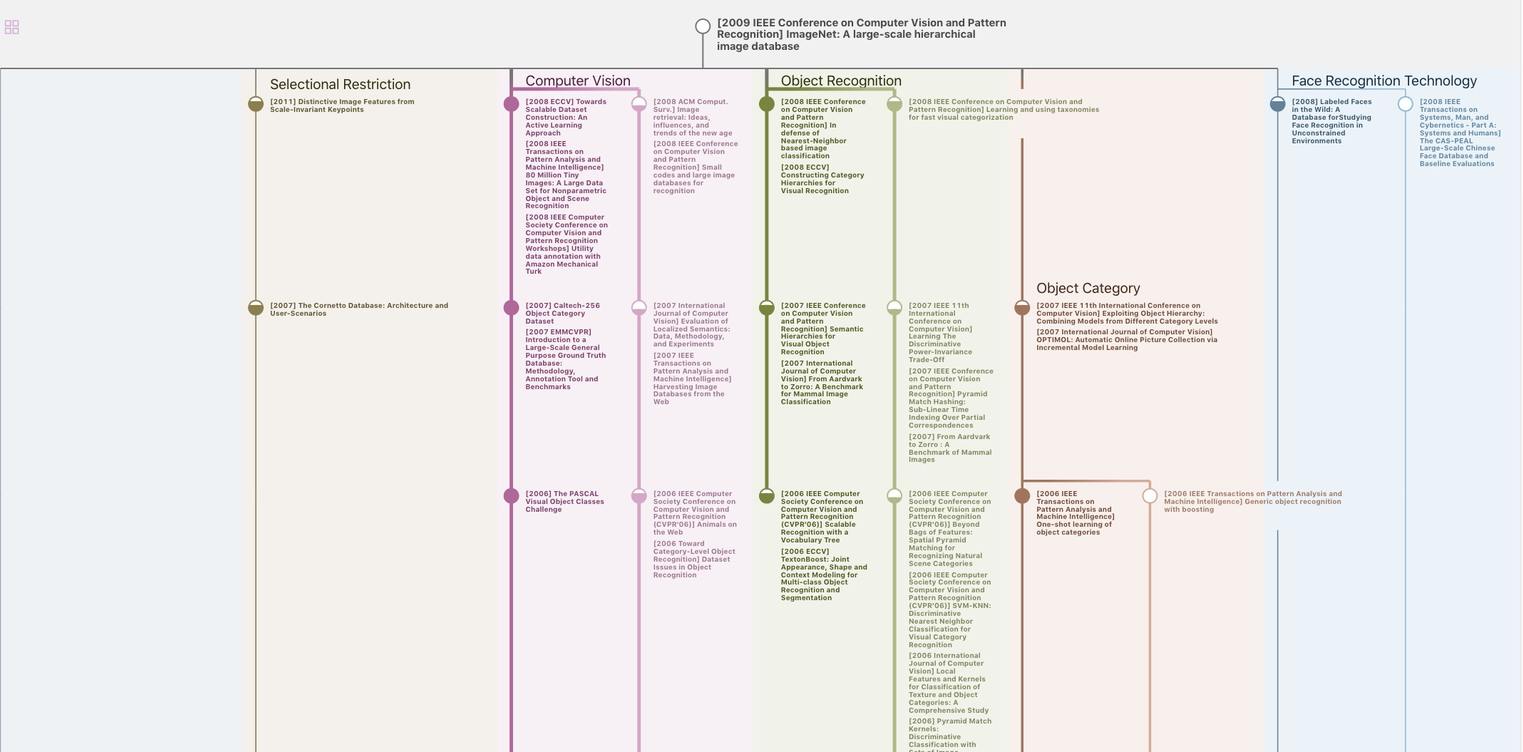
生成溯源树,研究论文发展脉络
Chat Paper
正在生成论文摘要