The Future Strikes Back: Using Future Treatments to Detect and Reduce Hidden Bias.
SOCIOLOGICAL METHODS & RESEARCH(2019)
摘要
Conventional advice discourages controlling for postoutcome variables in regression analysis. By contrast, we show that controlling for commonly available postoutcome (i.e., future) values of the treatment variable can help detect, reduce, and even remove omitted variable bias (unobserved confounding). The premise is that the same unobserved confounder that affects treatment also affects the future value of the treatment. Future treatments thus proxy for the unmeasured confounder, and researchers can exploit these proxy measures productively. We establish several new results: Regarding a commonly assumed data-generating process involving future treatments, we (1) introduce a simple new approach and show that it strictly reduces bias, (2) elaborate on existing approaches and show that they can increase bias, (3) assess the relative merits of alternative approaches, and (4) analyze true state dependence and selection as key challenges. (5) Importantly, we also introduce a new nonparametric test that uses future treatments to detect hidden bias even when future-treatment estimation fails to reduce bias. We illustrate these results empirically with an analysis of the effect of parental income on children's educational attainment.
更多查看译文
关键词
causal inference, confounding, bias, directed acyclic graphs, future treatments
AI 理解论文
溯源树
样例
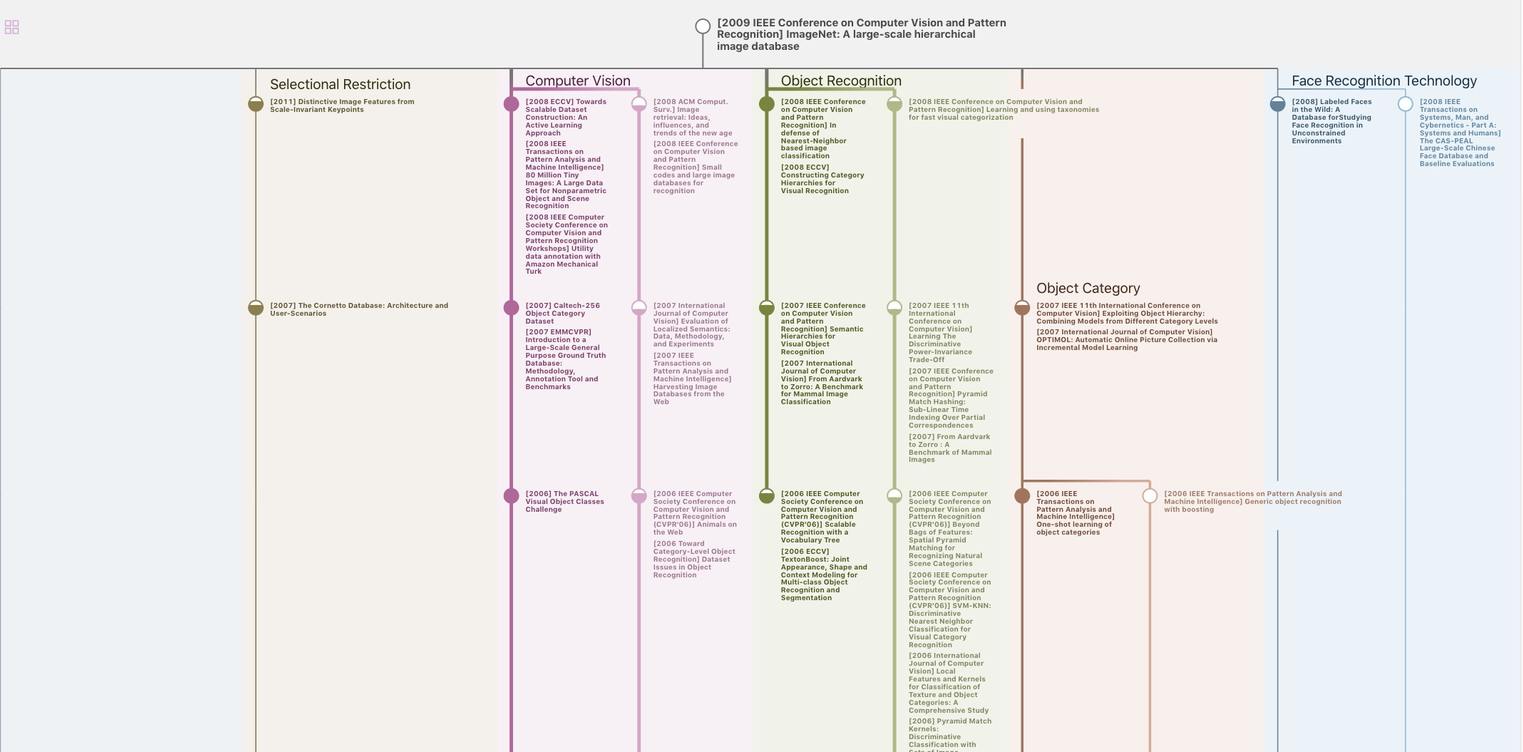
生成溯源树,研究论文发展脉络
Chat Paper
正在生成论文摘要