Multi-Task Deep Learning for Pedestrian Detection, Action Recognition and Time to Cross Prediction
IEEE Access(2019)
摘要
A pedestrian detection system is a crucial component of advanced driver assistance systems since it contributes to road flow safety. The safety of traffic participants could be significantly improved if these systems could also predict and recognize pedestrian's actions, or even estimate the time, for each pedestrian, to cross the street. In this paper, we focus not only on pedestrian detection and pedestrian action recognition but also on estimating if the pedestrian's action presents a risky situation according to time to cross the street. We propose 1) a pedestrian detection and action recognition component based, on RetinaNet; 2) an estimation of the time to cross the street for multiple pedestrians using a recurrent neural network. For each pedestrian, the recurrent network estimates the pedestrian's action intention in order to predict the time to cross the street. We based our experiments on the JAAD dataset, and show that integrating multiple pedestrian action tags for the detection part when merge with a recurrent neural network (LSTM) allows a significant performance improvement.
更多查看译文
关键词
Estimation,Roads,Deep learning,Safety,Recurrent neural networks,Automobiles,Trajectory,Action recognition,deep learning,pedestrian detection,time-to-cross estimation
AI 理解论文
溯源树
样例
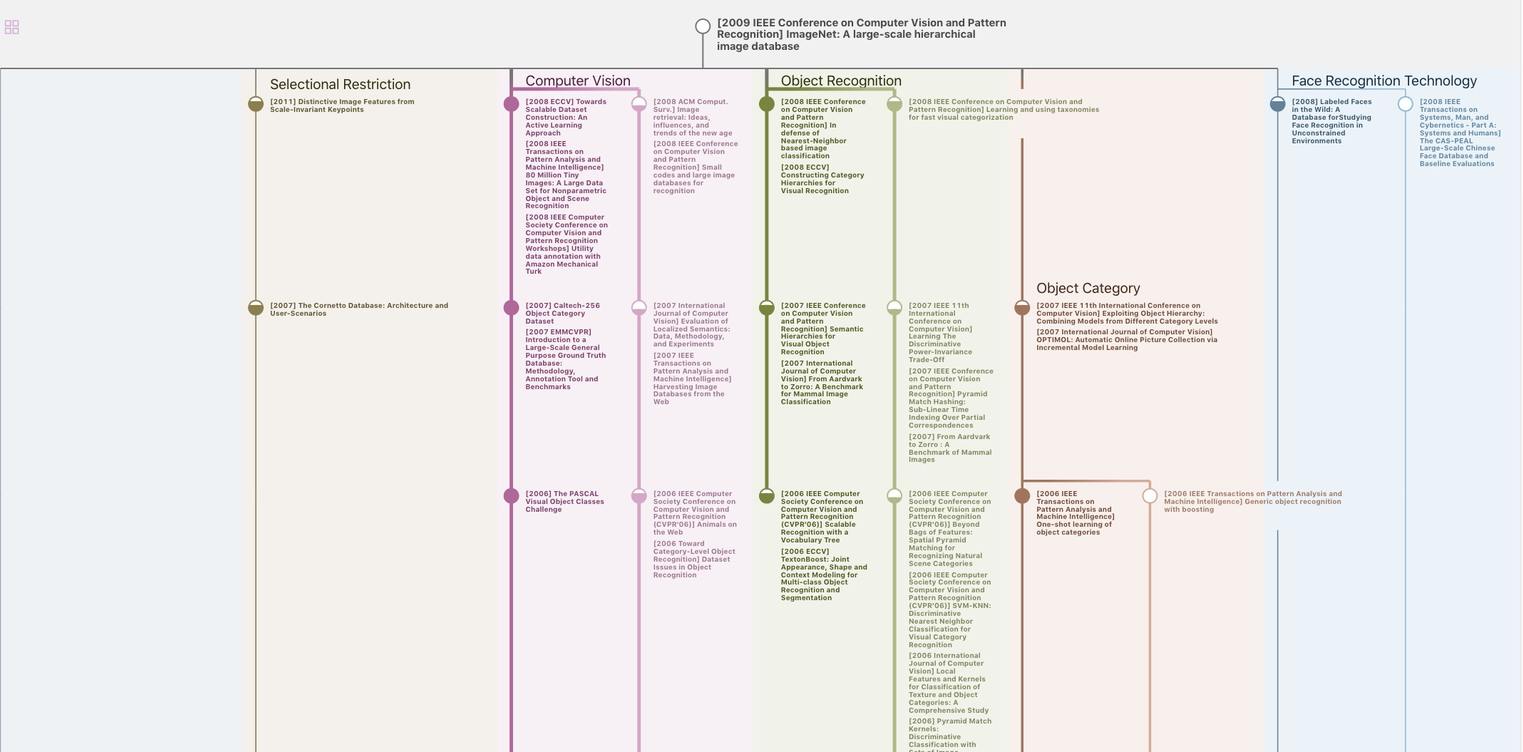
生成溯源树,研究论文发展脉络
Chat Paper
正在生成论文摘要