Method For Probabilistic Flood Forecasting Considering Rainfall And Model Parameter Uncertainties
JOURNAL OF HYDROLOGIC ENGINEERING(2019)
摘要
Hydrologic basin models are generalizations of natural hydrologic processes and inevitably contain multiple uncertainties in the simulation of rainfall-runoff events. These uncertainties typically include input uncertainty, model structure uncertainty, and model parameter uncertainty. The input uncertainty is divided into rainfall calculation uncertainty (RCU) and precipitation forecasting uncertainty. For model parameter uncertainty, there are only a few parameters in a hydrologic basin model that make significant contributions to flood forecasting so that only the probability distribution functions of those sensitive parameters need to be evaluated. In this study, a new method of RCU assessment is derived using an inverse sampling gauge (ISG) approach, in which the influencing factors of the RCU are addressed in the calculated area precipitation and standard deviation. Additionally, the coefficient of variation-Nash-Sutcliffe efficiency measure (CV-NS) method is introduced to identify the sensitive parameters of a hydrologic model. The methods of ISG and CV-NS are tested in Huangnizhuang Basin, China, in the evaluation of the RCU and the parameters uncertainty of the Xinanjiang model. It is indicated that the ISG method is useful in RCU estimation by deriving the conditional probabilistic distribution of areal precipitation, and the CV-NS method is effective and simply in the operation of sensitive parameters' identification. In addition, the continuous ranked probability skill score (CRPSS) is employed as an indicator to show the relative performance of predictions. Three probabilistic predictions considering different sources of uncertainties are conducted and compared with the deterministic prediction. The results of the comparisons suggest that predictions considering one or more uncertainties have higher predictive performance than the deterministic one. Moreover, main source of uncertainty can be identified by the results of CRPSS among different probabilistic predictions. In this study area, the model parameters' uncertainty is the main uncertainty. (C) 2019 American Society of Civil Engineers.
更多查看译文
关键词
Calculated areal precipitation (CAP), Sensitive parameter identification, Continuous ranked probability skill score (CRPSS), Xinanjiang hydrologic basin model, Huangnizhuang Basin
AI 理解论文
溯源树
样例
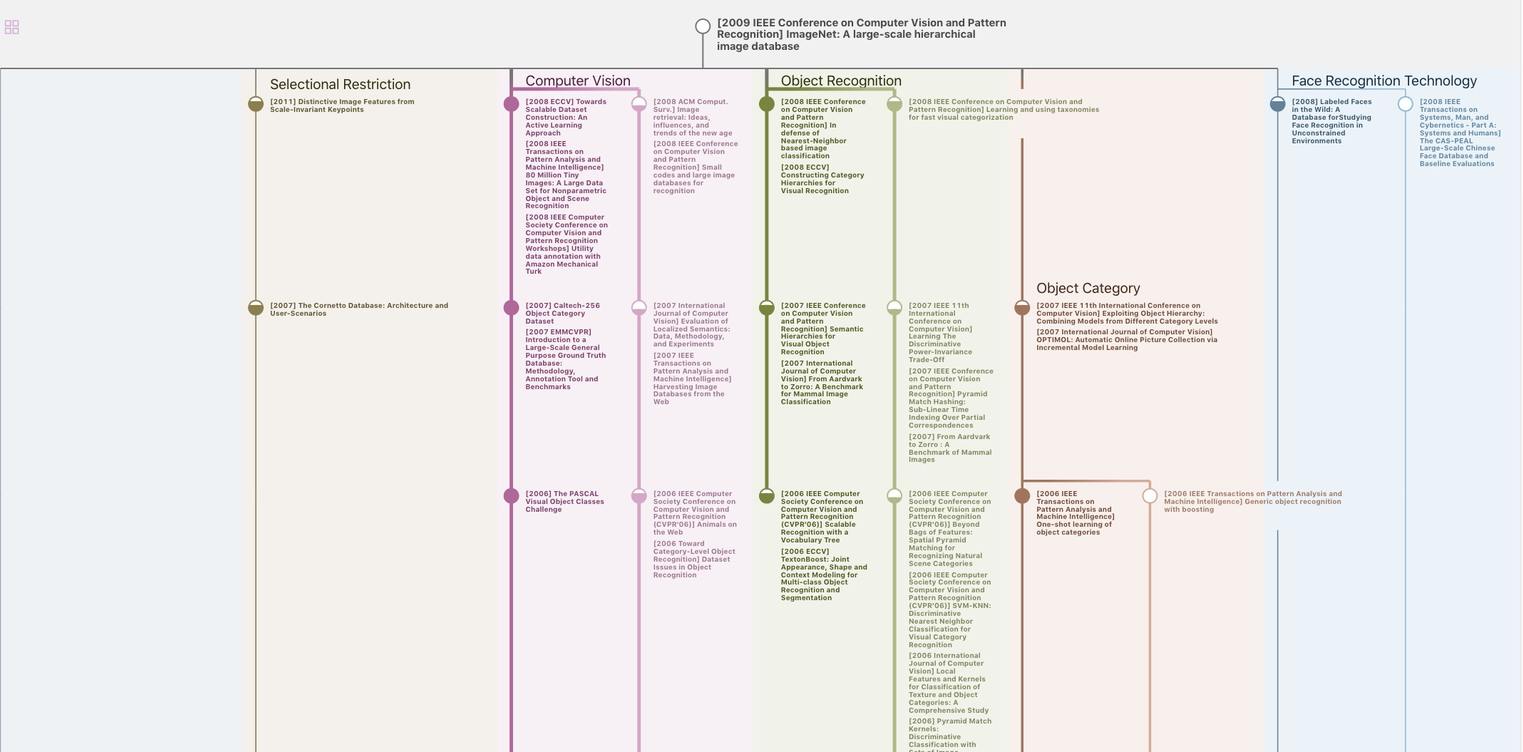
生成溯源树,研究论文发展脉络
Chat Paper
正在生成论文摘要