Approximate lumpability for Markovian agent-based models using local symmetries
JOURNAL OF APPLIED PROBABILITY(2019)
摘要
We study a Markovian agent-based model (MABM) in this paper. Each agent is endowed with a local state that changes over time as the agent interacts with its neighbours. The neighbourhood structure is given by a graph. Recently. Simon, Taylor, and Kiss [40] used the automorphisms of the underlying graph to generate a lumpable partition of the joint state space, ensuring Markovianness of the lumped process for binary dynamics. However, many large random graphs tend to become asymmetric, rendering the automorphism-based lumping approach ineffective as a tool of model reduction. In order to mitigate this problem, we propose a lumping method based on a notion of local symmetry, which compares only local neighbourhoods of vertices. Since local symmetry only ensures approximate lumpability, we quantify the approximation error by means of the Kullback-Leibler divergence rate between the original Markov chain and a lifted Markov chain. We prove the approximation error decreases monotonically. The connections to fibrations of graphs are also discussed.
更多查看译文
关键词
Lumpability,aggregation,agent-based models,local symmetry,fibration
AI 理解论文
溯源树
样例
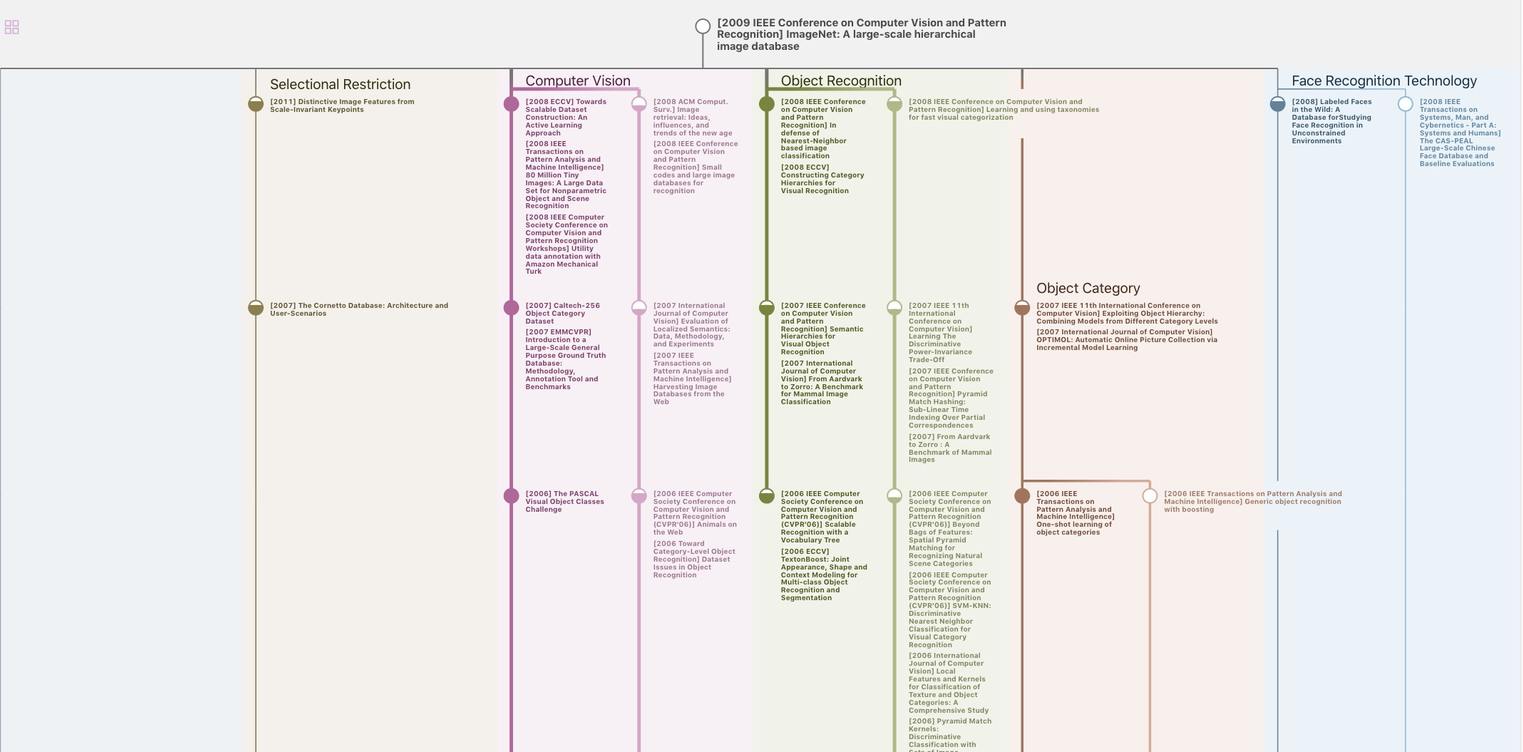
生成溯源树,研究论文发展脉络
Chat Paper
正在生成论文摘要