Gaussian Process Modeling of Finite Element Models with Functional Inputs
SIAM-ASA JOURNAL ON UNCERTAINTY QUANTIFICATION(2019)
摘要
Partial differential equation (PDE) models are often solved numerically. The solution to a PDE model, i.e., the output, and three types of inputs, called the source term, boundary conditions, and initial conditions, are functions of space and/or time. The obvious approach to build an emulator relating the PDE solution to parameters of these functional inputs is to model the relationship with a stationary Gaussian process (GP) model. Many physics-based and statistical models of functional inputs employed in PDE modeling depend nonlinearly on their parameters. Thus, the relationship between functional input parameters and a PDE solution can be highly nonlinear. However, it is known that the solution of many PDE models is approximately a linear function of the source term, boundary conditions, and initial conditions, and the response of the solution to a change in these functional inputs cannot precede the change in time. For linear PDEs, such phenomena are explained by Green's function. The obvious approach ignores this information. This paper proposes a GP emulator that is the sum of a functional linear regression term built from a kernel that takes the role of Green's function and a GP model for the residual. The functional linear regression term transforms the nonlinear effect of the functional input parameters into a functional linear effect that can be more reliably estimated with data. Examples demonstrate large improvements obtained with the proposed model. MATLAB codes for reproducing results in the paper can be found in Appendix A.
更多查看译文
关键词
computer experiment,functional regression,interpolation,random field input
AI 理解论文
溯源树
样例
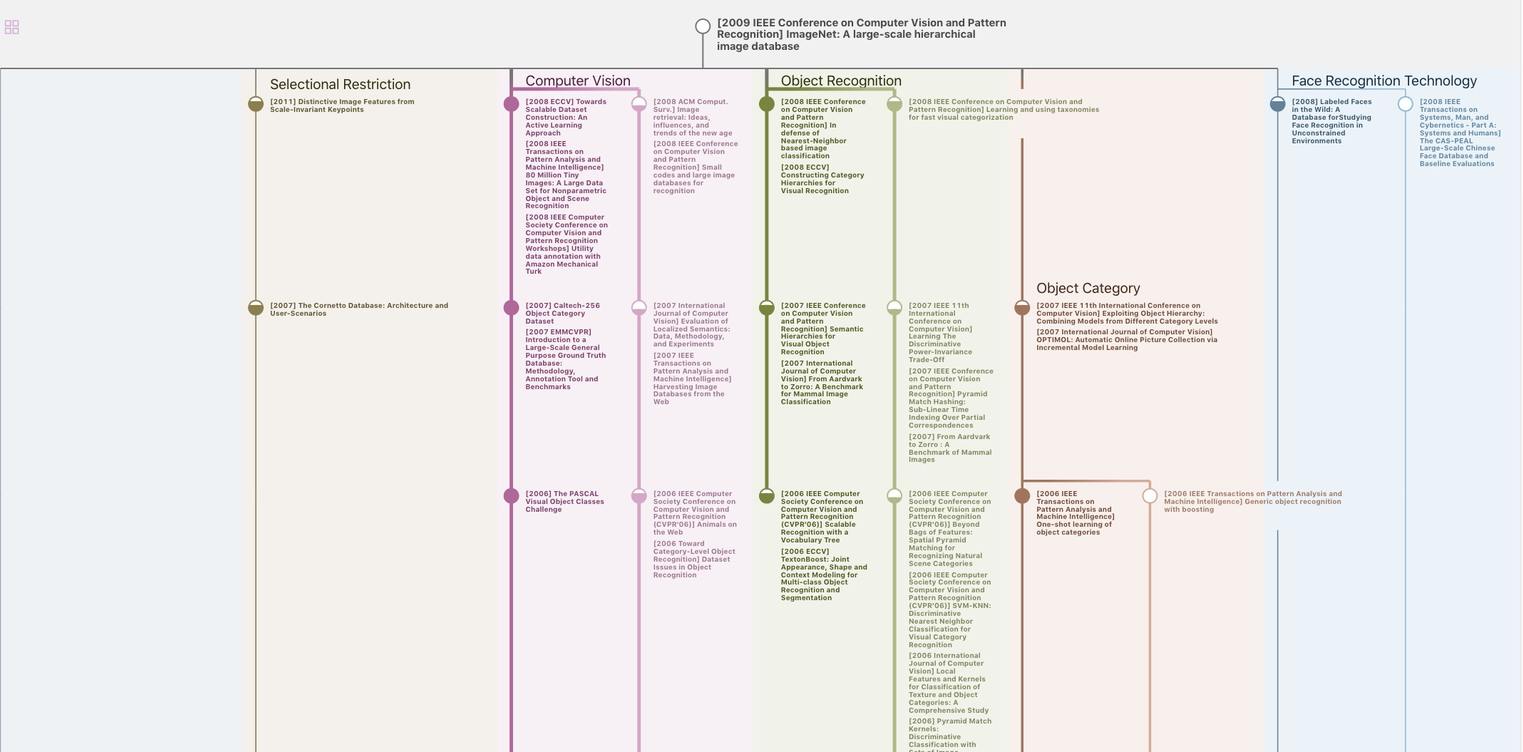
生成溯源树,研究论文发展脉络
Chat Paper
正在生成论文摘要