When QoE Meets Learning: A Distributed Traffic-Processing Framework for Elastic Resource Provisioning in HetNets
Computer Networks(2020)
摘要
In heterogeneous networks (HetNets), dynamics of user requirements across the temporal domain lead to the order of magnitude traffic to be processed by macro cells. To achieve high quality of experience (QoE) for users and to perform resource allocation for cells intelligently, we first propose a distributed traffic-processing framework (SDVTS) for elastic resource partitioning, to accommodate dynamics from the user-centric and resource-oriented perspectives respectively. Assisted by a software defined infrastructure, SDVTS fulfills the responsibilities of the request-based and push-based services in an interactive loop. Second, we formulate a traffic-processing time model that computes the delay of handling traffic. The non-convex model is decomposed and a dual evolution algorithm is explored to approximate the optimal solution. Furthermore, we introduce a low-complexity reinforcement learning algorithm with the personalized QoE profiling. A distributed algorithm in coalition between user and cell is designed for seamless connection of an advanced reinforcement learning system (ARLS) components and engines embedded in SDVTS. Extensive simulation results with thorough analysis demonstrate that our framework SDVTS dominates in terms of QoE and cell′s system performance when compared with competing approaches.
更多查看译文
关键词
Reinforcement learning,Resource provisioning,Software defined infrastructure,QoE,Traffic-processing framework
AI 理解论文
溯源树
样例
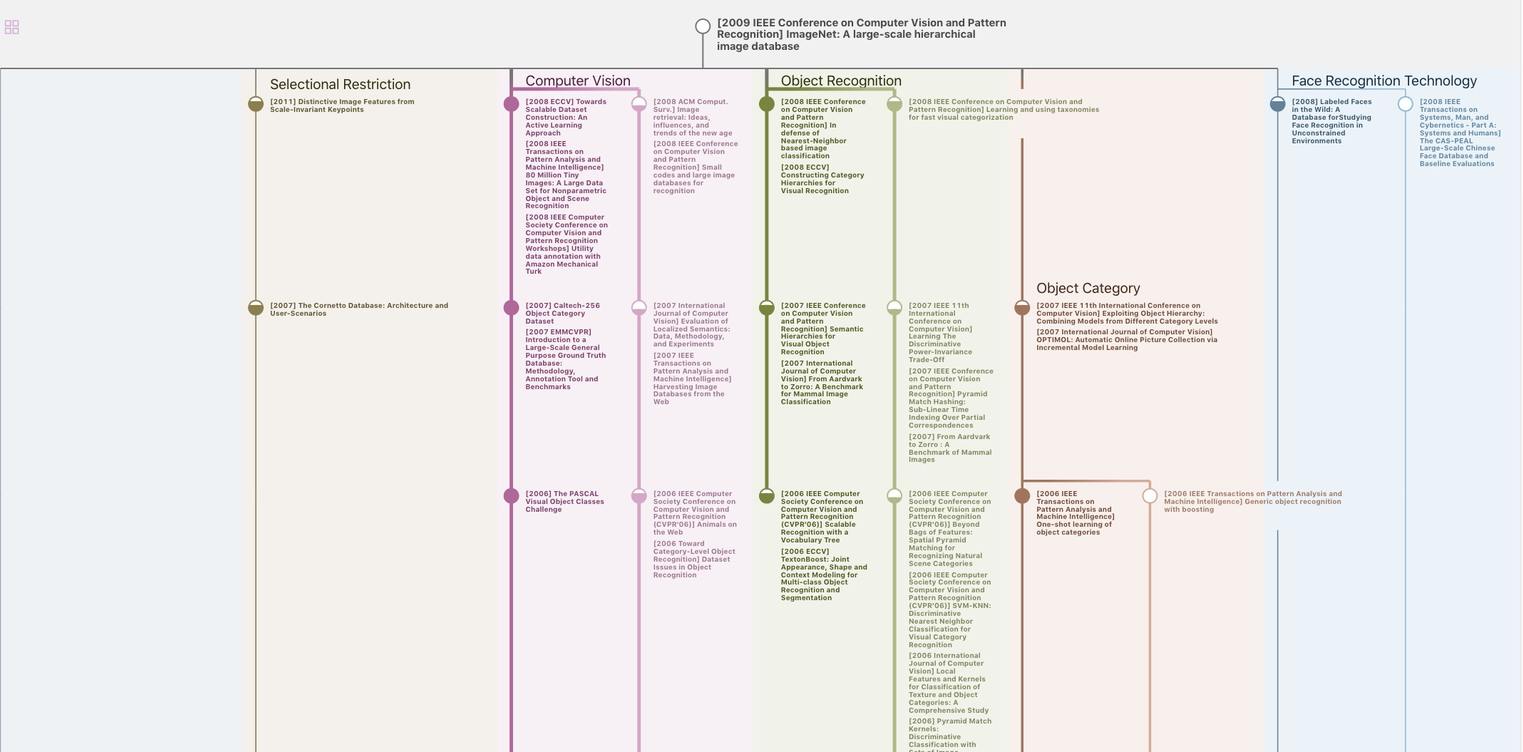
生成溯源树,研究论文发展脉络
Chat Paper
正在生成论文摘要