A Personalized Model for Driver Lane-Changing Behavior Prediction Using Deep Neural Network
2019 2nd International Conference on Artificial Intelligence and Big Data (ICAIBD)(2019)
摘要
Lane changes are stressful maneuvers for drivers, particularly during high-speed traffic flows. Modeling driver's lane-changing decision and implementation process is challenging due to the complexity and uncertainty of driving behaviors. To address this issue, this paper presents a personalized Model, LCPM, for Lane-Changing Behavior Prediction based on the Root Residual Network (Root-ResNet) backbone from vehicle dynamics data and driver physiological data. Firstly, with abundant inputs of Root-ResNet, LCPM is able to exploit more local information from two differing modality data. Secondly, LCPM has an ability of using global information to selectively emphasise informative features and suppress less useful ones with a special regularization method, Weighted Channel Dropout (WCD). The experimental results show that the LCPM is capable of learning the latent features of lane-changing behaviors and achieving significantly better performance than other popular networks on a real-world driving dataset.
更多查看译文
关键词
lane-changing behavior prediction,deep learning,weighted channel dropout,Root-ResNet
AI 理解论文
溯源树
样例
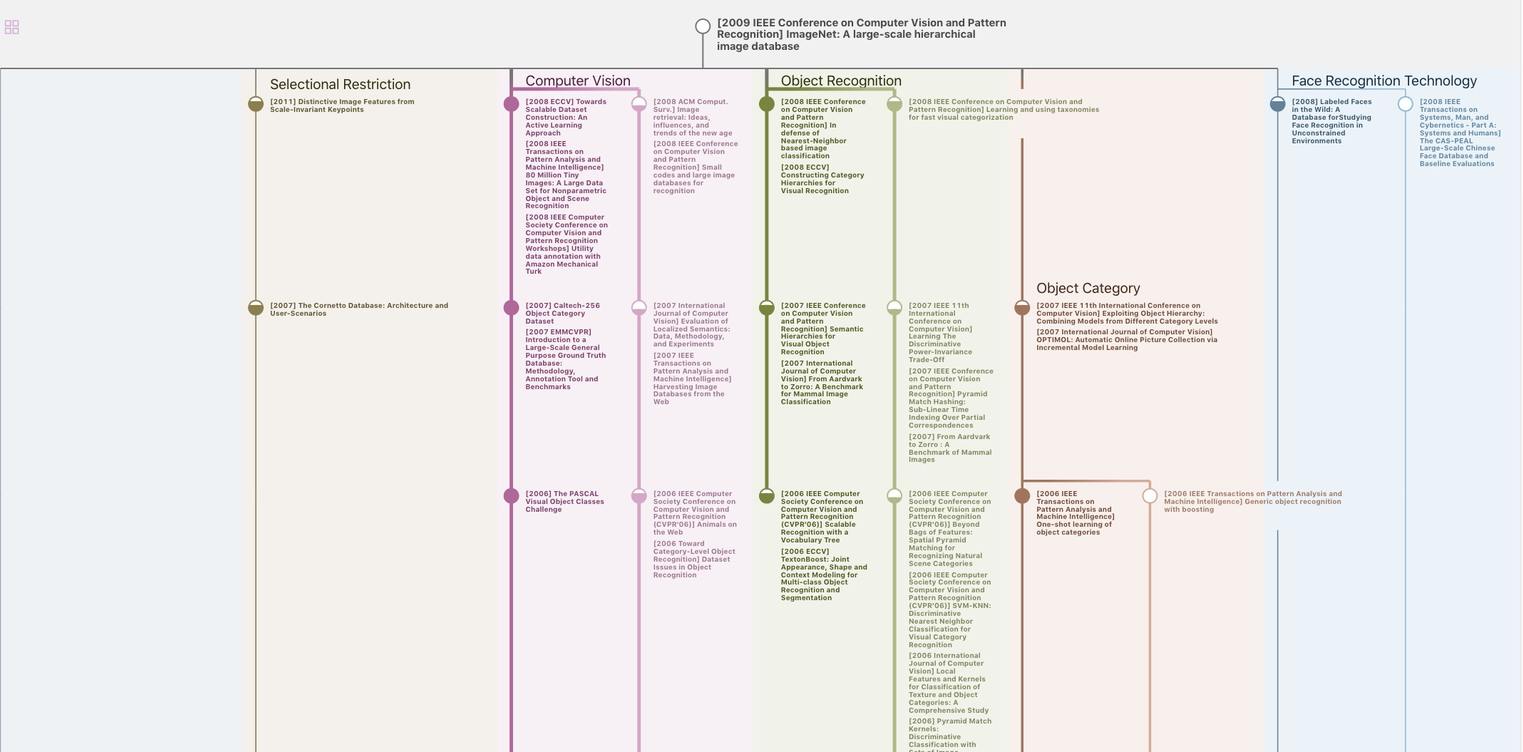
生成溯源树,研究论文发展脉络
Chat Paper
正在生成论文摘要