Semiparametric Garch Via Bayesian Model Averaging
JOURNAL OF BUSINESS & ECONOMIC STATISTICS(2021)
摘要
As the dynamic structure of financial markets is subject to dramatic change, a model capable of providing consistently accurate volatility estimates should not make rigid assumptions on how prices change over time. Most volatility models impose a particular parametric functional form that relates an observed price change to a volatility forecast (news impact function). Here, a new class of functional coefficient semiparametric volatility models is proposed, where the news impact function is allowed to be any smooth function. The ability of the proposed model to estimate volatility is studied and compared to the well-known parametric proposals, in both a simulation study and an empirical study with real financial market data. The news impact function is estimated using a Bayesian model averaging approach, implemented via a carefully developed Markov chain Monte Carlo sampling algorithm. Using simulations it is shown that the proposed flexible semiparametric model is able to learn the shape of the news impact function very effectively, from observed data. When applied to real financial time series, the proposed model suggests that news impact functions have quite different shapes over different asset types, but a consistent shape within the same asset class. for this article are available online.
更多查看译文
关键词
Functional coefficient, Heavy tail, Markov chain Monte Carlo, News impact function, Regression spline, Volatility
AI 理解论文
溯源树
样例
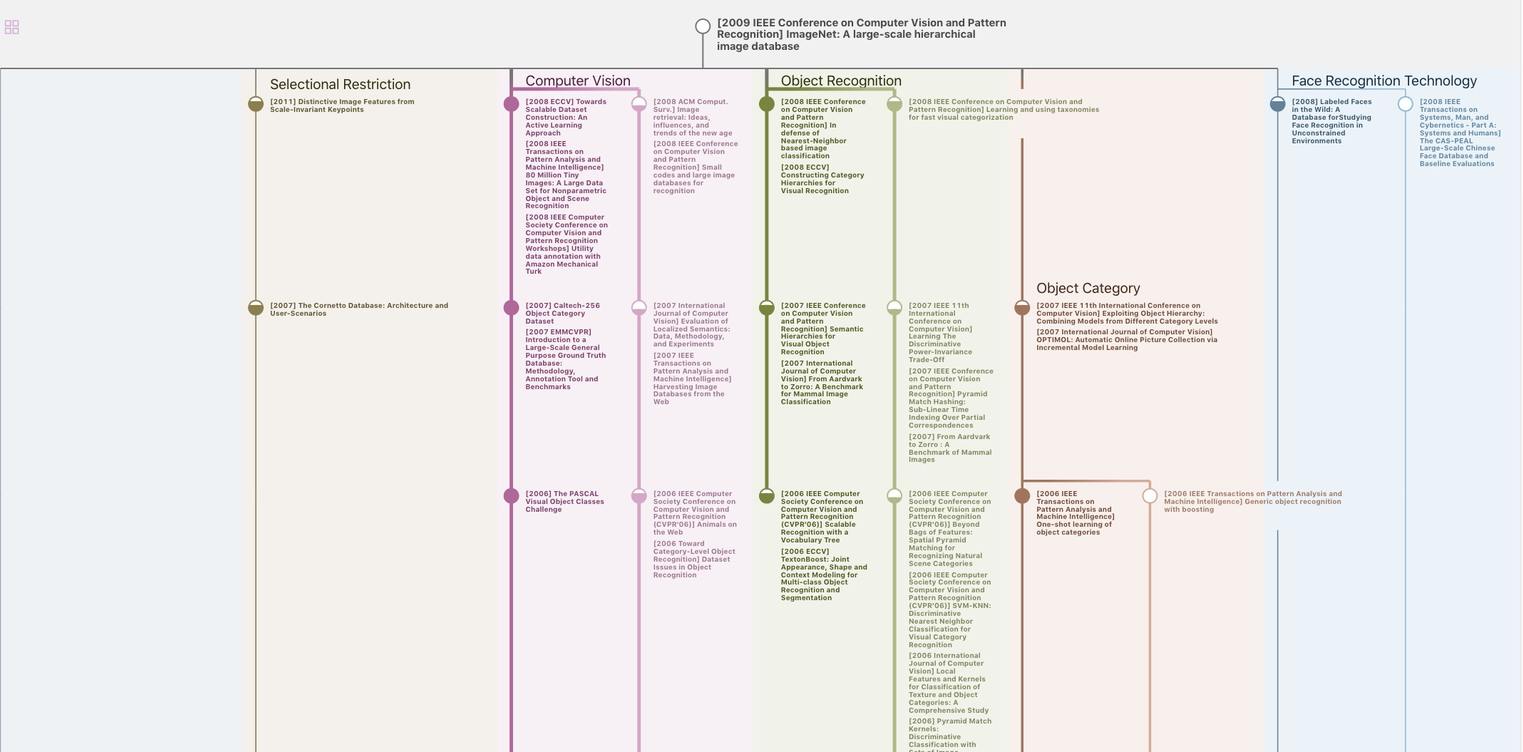
生成溯源树,研究论文发展脉络
Chat Paper
正在生成论文摘要