A Saliency-Based Attention LSTM Model for Cognitive Load Classification from Speech
INTERSPEECH(2019)
摘要
Cognitive Load (CL) refers to the amount of mental demand that a given task imposes on an individual's cognitive system and it can affect his/her productivity in very high load situations. In this paper, we propose an automatic system capable of classifying the CL level of a speaker by analyzing his/her voice. Our research on this topic goes into two main directions. In the first one, we focus on the use of Long Short-Term Memory (LSTM) networks with different weighted pooling strategies for CL level classification. In the second contribution, for overcoming the need of a large amount of training data, we propose a novel attention mechanism that uses the Kalinli's auditory saliency model. Experiments show that our proposal outperforms significantly both, a baseline system based on Support Vector Machines (SVM) and a LSTM-based system with logistic regression attention model.
更多查看译文
关键词
cognitive load, speech, LSTM, weigthed pooling, auditory saliency, attention model
AI 理解论文
溯源树
样例
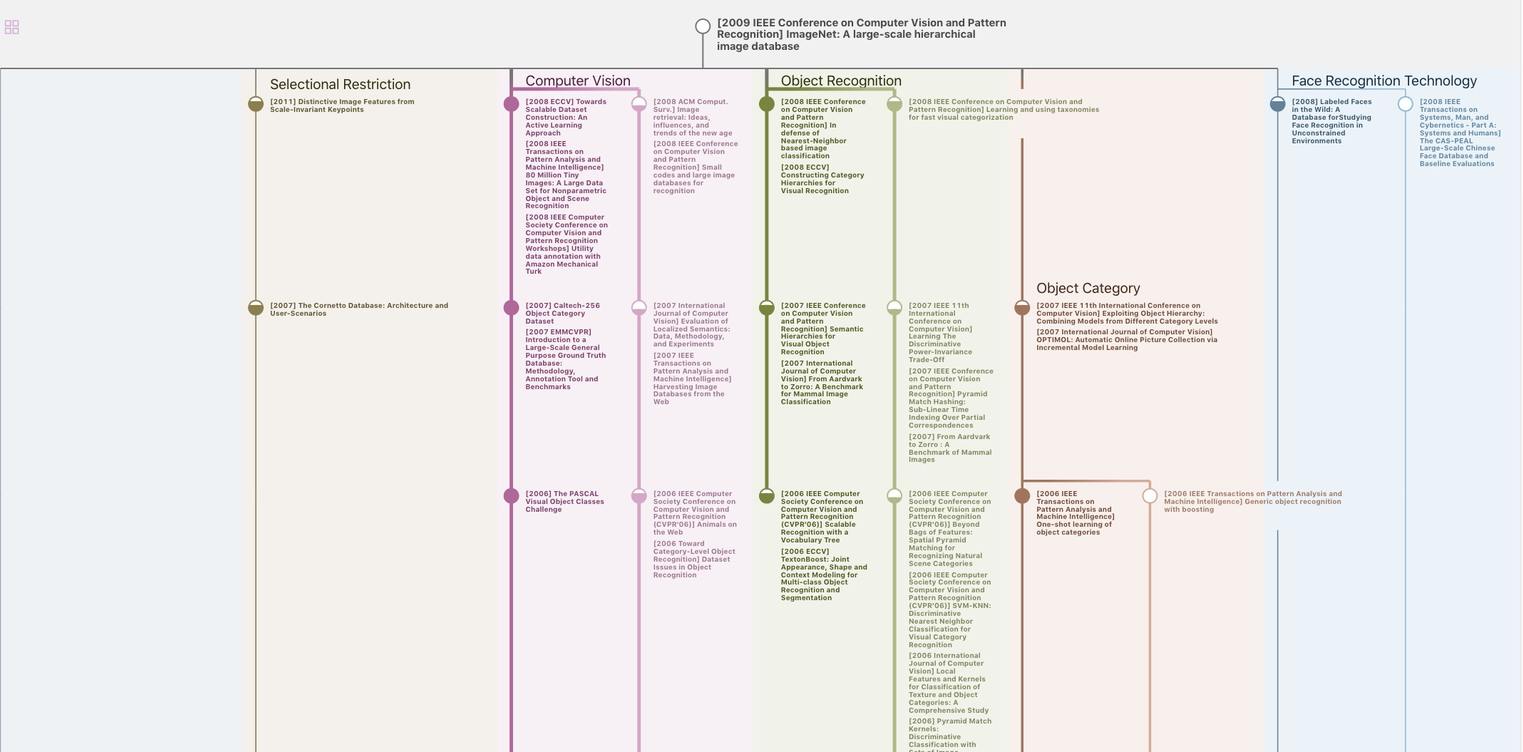
生成溯源树,研究论文发展脉络
Chat Paper
正在生成论文摘要