Sparse Recurrent Mixture Density Networks For Forecasting High Variability Time Series With Confidence Estimates
ARTIFICIAL NEURAL NETWORKS AND MACHINE LEARNING - ICANN 2019: DEEP LEARNING, PT II(2019)
摘要
Accurate forecasting of a high variability time series has relevance in many applications such as supply-chain management, price prediction in stock markets and demand forecasting in the energy segment. Most often forecasts of such time series depend on many factors ranging from weather to socio-economic attributes such as GDP or average income. Dependence on such features can cause the underlying time series to be highly variable in nature and possess non-stationary shifts. Most traditional forecasting methods fail to capture such trend shifts and high variability present in the data. Further, for certain applications, it may be necessary to estimate the confidence of the forecasts. In this work, we propose two variants of recurrent mixture density network (RMDN), for time series forecasting, that have the ability to handle high-dimensional input features, capture trend shifts and high variability present in the data, and provide a confidence estimate of the forecast. To this end, we first pass the high-dimensional time series data through a feedforward layer, which performs dimensionality reduction or feature selection in an unsupervised manner by inducing sparsity on the weights of the feedforward layer. The resultant low-dimensional time series is then fed through recurrent layers to capture temporal patterns. These recurrent layers also aid in learning the latent representation of the input data. Thereafter, a mixture density network (MDN) is used to model the variability and trend shifts present in the input and it also estimates the confidence of the predictions. The models are trained in an end-to-end fashion and the efficacy of the proposed models is demonstrated on three publicly available datasets from energy markets.
更多查看译文
关键词
Recurrent mixture density networks, Sparse neural networks, Highly variable time series, Forecasting, Confidence estimation of prediction, Dimensionality reduction
AI 理解论文
溯源树
样例
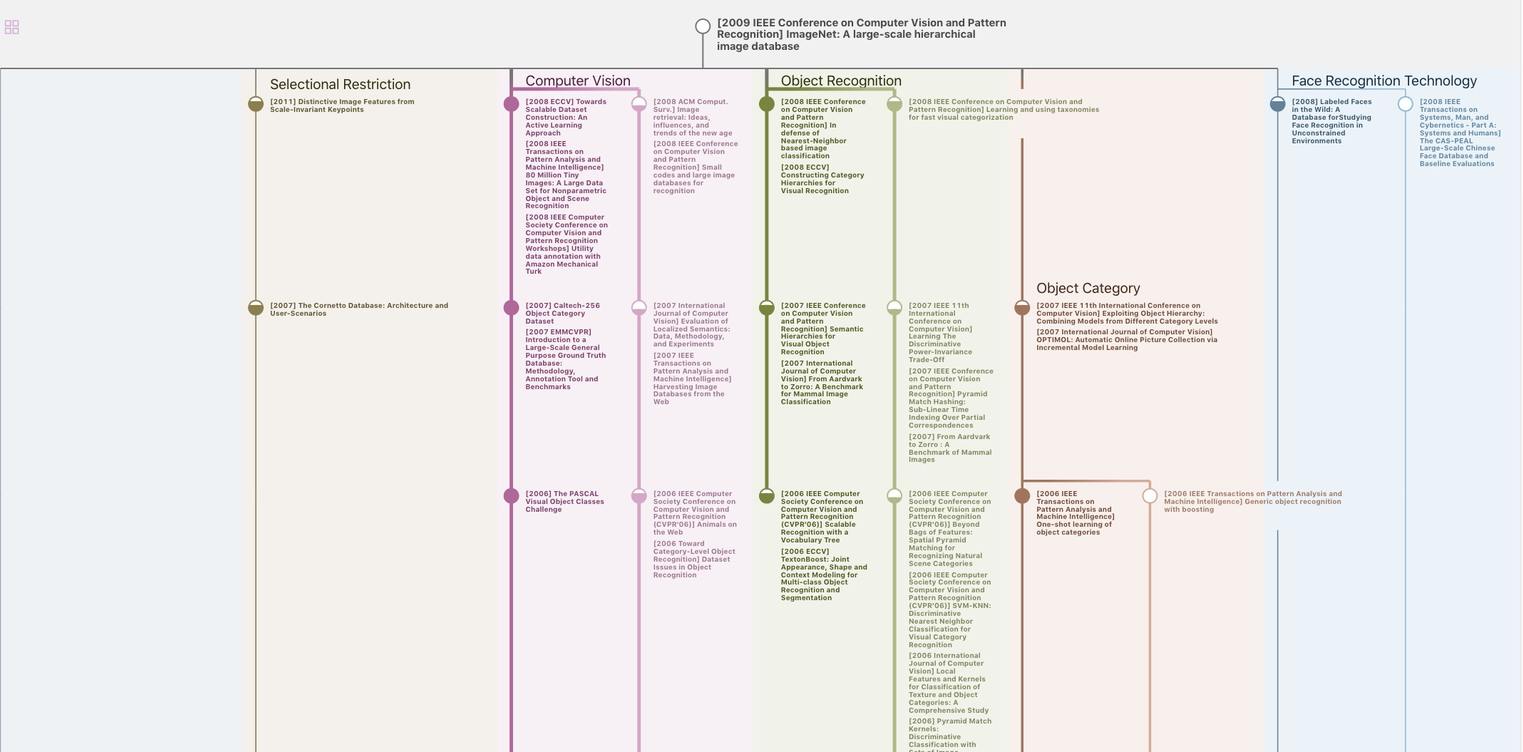
生成溯源树,研究论文发展脉络
Chat Paper
正在生成论文摘要