Semi-Supervised Prosody Modeling Using Deep Gaussian Process Latent Variable Model
INTERSPEECH(2019)
摘要
This paper proposes a semi-supervised speech synthesis framework in which prosodic labels of training data are partially annotated. When we construct a text-to-speech (TTS) system, it is crucial to use appropriately annotated prosodic labels. For this purpose, manually annotated ones would provide a good result, but it generally costs much time and patience. Although recent studies report that end-to-end TTS framework can generate natural-sounding prosody without using prosodic labels, this does not always appear in arbitrary languages such as pitch accent ones. Alternatively, we propose an approach to utilizing a latent variable representation of prosodic information. In the latent variable representation, we employ deep Gaussian process (DGP), a deep Bayesian generative model. In the proposed semi-supervised learning framework, the posterior distributions of latent variables are inferred from linguistic and acoustic features, and the inferred latent variables are utilized to train a DGP-based regression model of acoustic features. Experimental results show that the proposed framework can give a comparable performance with the case using fully-annotated speech data in subjective evaluation even if the prosodic information of pitch accent is limited.
更多查看译文
关键词
deep Gaussian process, statistical speech synthesis, latent variable model, prosody, semi-supervised learning
AI 理解论文
溯源树
样例
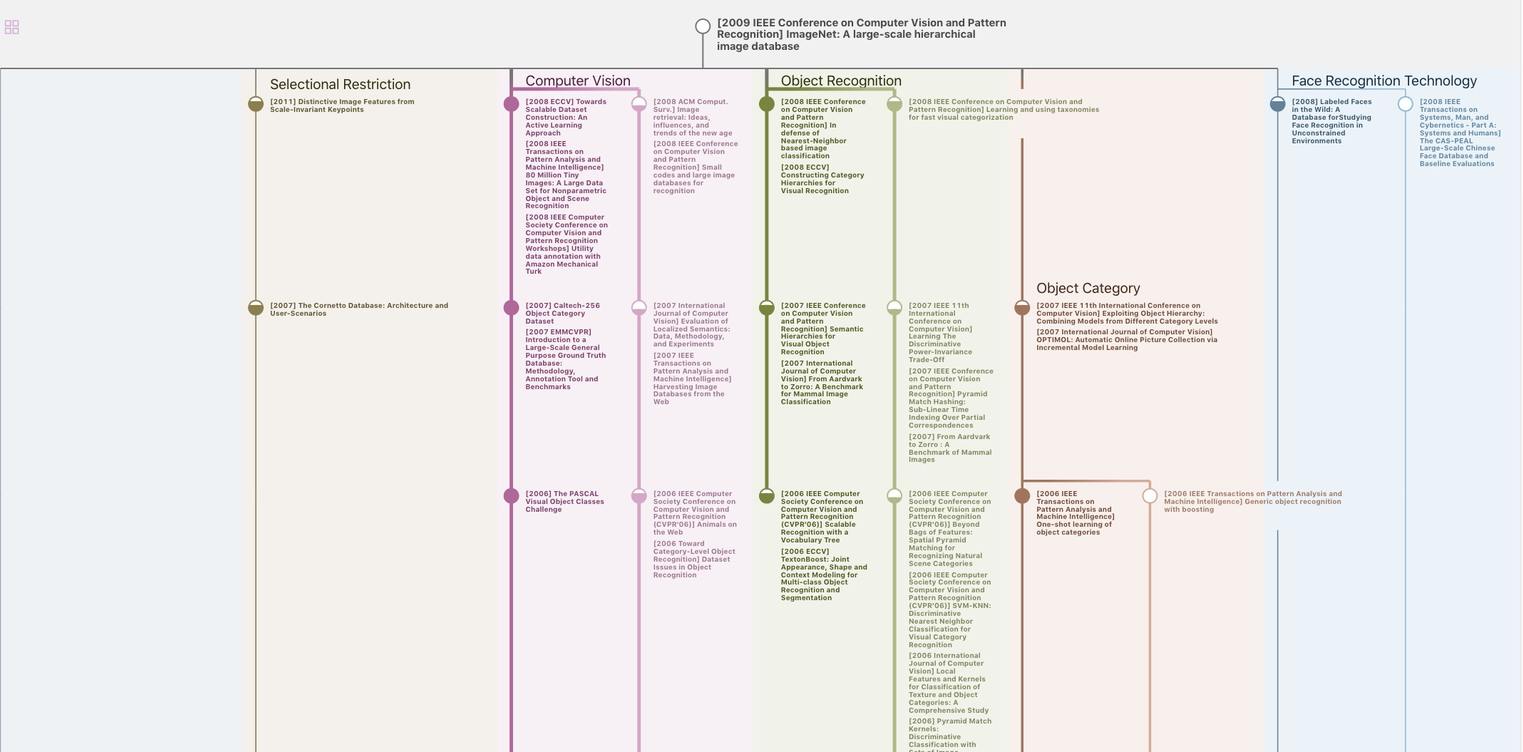
生成溯源树,研究论文发展脉络
Chat Paper
正在生成论文摘要