Local Walsh-average-based estimation and variable selection for single-index models
Science China Mathematics(2019)
摘要
We propose a robust estimation procedure based on local Walsh-average regression (LWR) for single-index models. Our novel method provides a root- n consistent estimate of the single-index parameter under some mild regularity conditions; the estimate of the unknown link function converges at the usual rate for the nonparametric estimation of a univariate covariate. We theoretically demonstrate that the new estimators show significant efficiency gain across a wide spectrum of non-normal error distributions and have almost no loss of efficiency for the normal error. Even in the worst case, the asymptotic relative efficiency (ARE) has a lower bound compared with the least squares (LS) estimates; the lower bounds of the AREs are 0.864 and 0.8896 for the single-index parameter and nonparametric function, respectively. Moreover, the ARE of the proposed LWR-based approach versus the ARE of the LS-based method has an expression that is closely related to the ARE of the signed-rank Wilcoxon test as compared with the t -test. In addition, to obtain a sparse estimate of the single-index parameter, we develop a variable selection procedure by combining the estimation method with smoothly clipped absolute deviation penalty; this procedure is shown to possess the oracle property. We also propose a Bayes information criterion (BIC)-type criterion for selecting the tuning parameter and further prove its ability to consistently identify the true model. We conduct some Monte Carlo simulations and a real data analysis to illustrate the finite sample performance of the proposed methods.
更多查看译文
关键词
single-index models, local Walsh-average regression, asymptotic relative efficiency, variable selection, oracle property, 62G20, 62H12
AI 理解论文
溯源树
样例
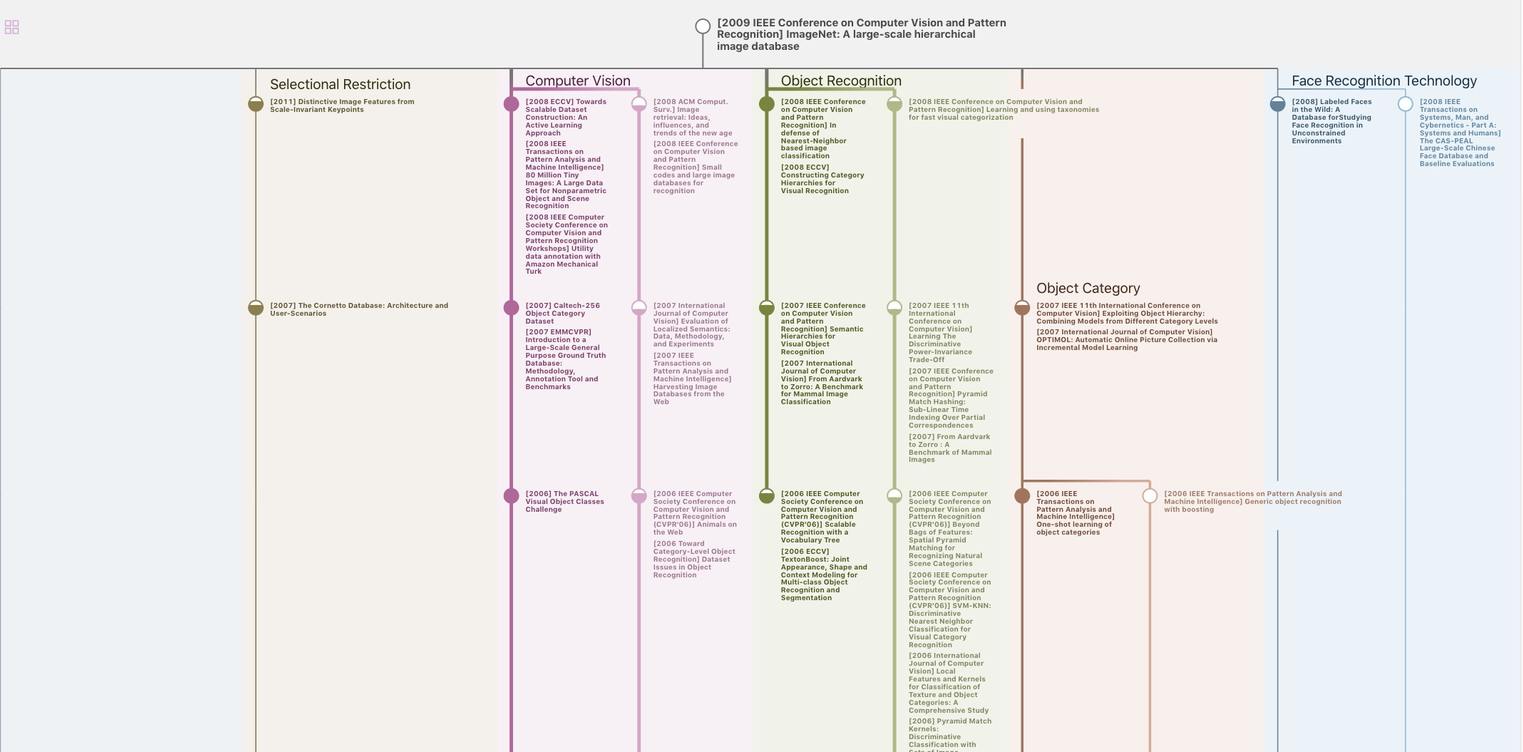
生成溯源树,研究论文发展脉络
Chat Paper
正在生成论文摘要