Doubly sparse regression incorporating graphical structure among predictors
CANADIAN JOURNAL OF STATISTICS-REVUE CANADIENNE DE STATISTIQUE(2019)
摘要
Recent research has demonstrated that information learned from building a graphical model on the predictor set of a regularized linear regression model can be leveraged to improve prediction of a continuous outcome. In this article, we present a new model that encourages sparsity at both the level of the regression coefficients and the level of individual contributions in a decomposed representation. This model provides parameter estimates with a finite sample error bound and exhibits robustness to errors in the input graph structure. Through a simulation study and the analysis of two real data sets, we demonstrate that our model provides a predictive benefit when compared to previously proposed models. Furthermore, it is a highly flexible model that provides a unified framework for the fitting of many commonly used regularized regression models. The Canadian Journal of Statistics 00: 000-000; 2019 (c) 2019 Statistical Society of Canada
更多查看译文
关键词
Prediction,undirected graphs,variable selection
AI 理解论文
溯源树
样例
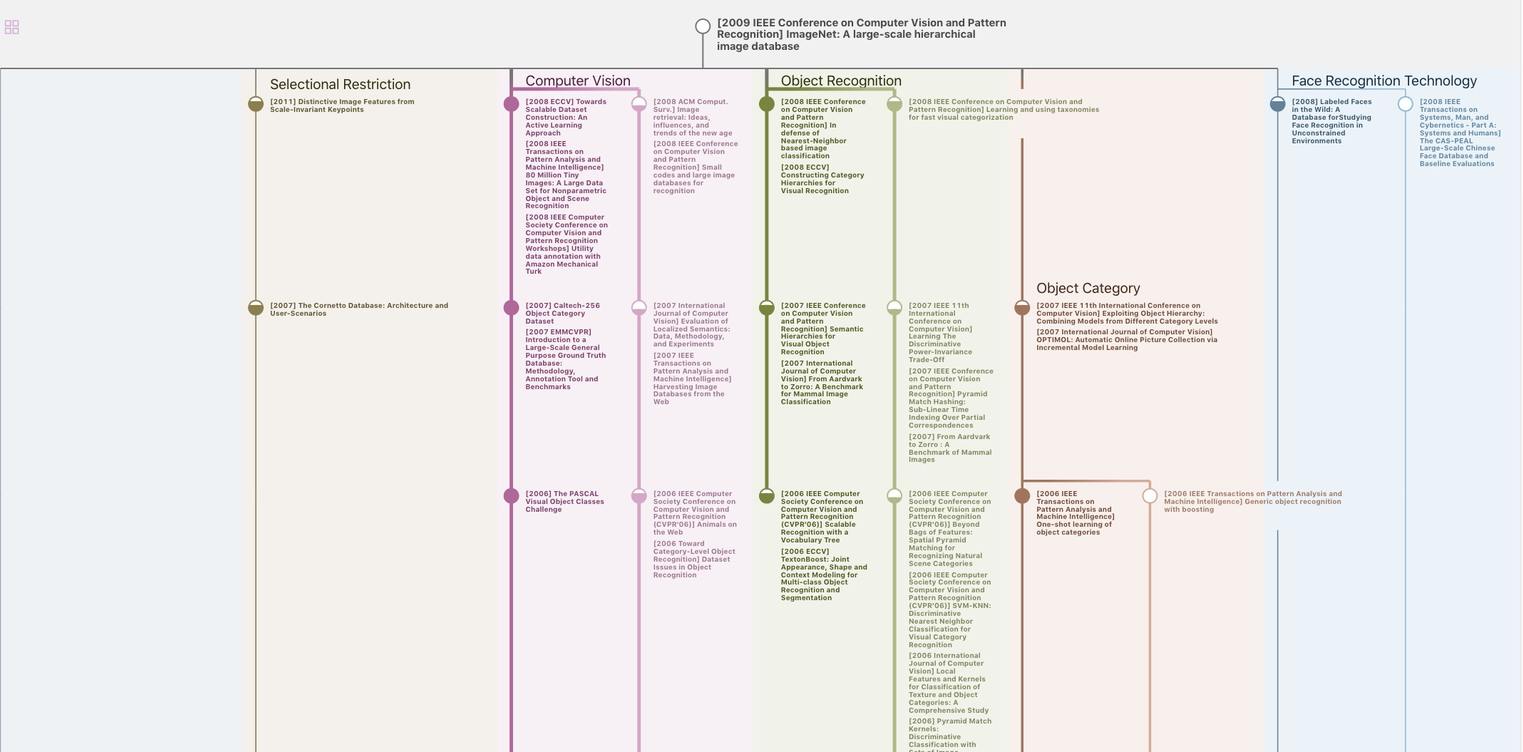
生成溯源树,研究论文发展脉络
Chat Paper
正在生成论文摘要