Pindrop Labs’ Submission to the First Multi-Target Speaker Detection and Identification Challenge
INTERSPEECH(2019)
摘要
This paper summarizes Pindrop Labs' submission to the multi-target speaker detection and identification challenge evaluation (MCE 2018). The MCE challenge is geared towards detecting blacklisted speakers (fraudsters) in the context of call centers. Particularly, it aims to answer the following two questions: Is the speaker of the test utterance on the blacklist? If so, which speaker is it among the blacklisted speakers? While one single system can answer both questions, this work looks at them as two separate tasks: blacklist detection and closed-set identification. The former is addressed using four different systems including probabilistic linear discriminant analysis (PLDA), two deep neural network (DNN) based systems, and a simple system based on cosine similarity and logistic regression. The latter is addressed by combining PLDA and neural network based systems. The proposed system was the best performing system at the challenge on both tasks, reducing the blacklist detection error (Top-S EER) by 31.9% and the identification error (Top-1 EER) by 46.4% over the MCE baseline on the evaluation data.
更多查看译文
关键词
MCE, blacklist detection, multi-speaker identification, speaker recognition
AI 理解论文
溯源树
样例
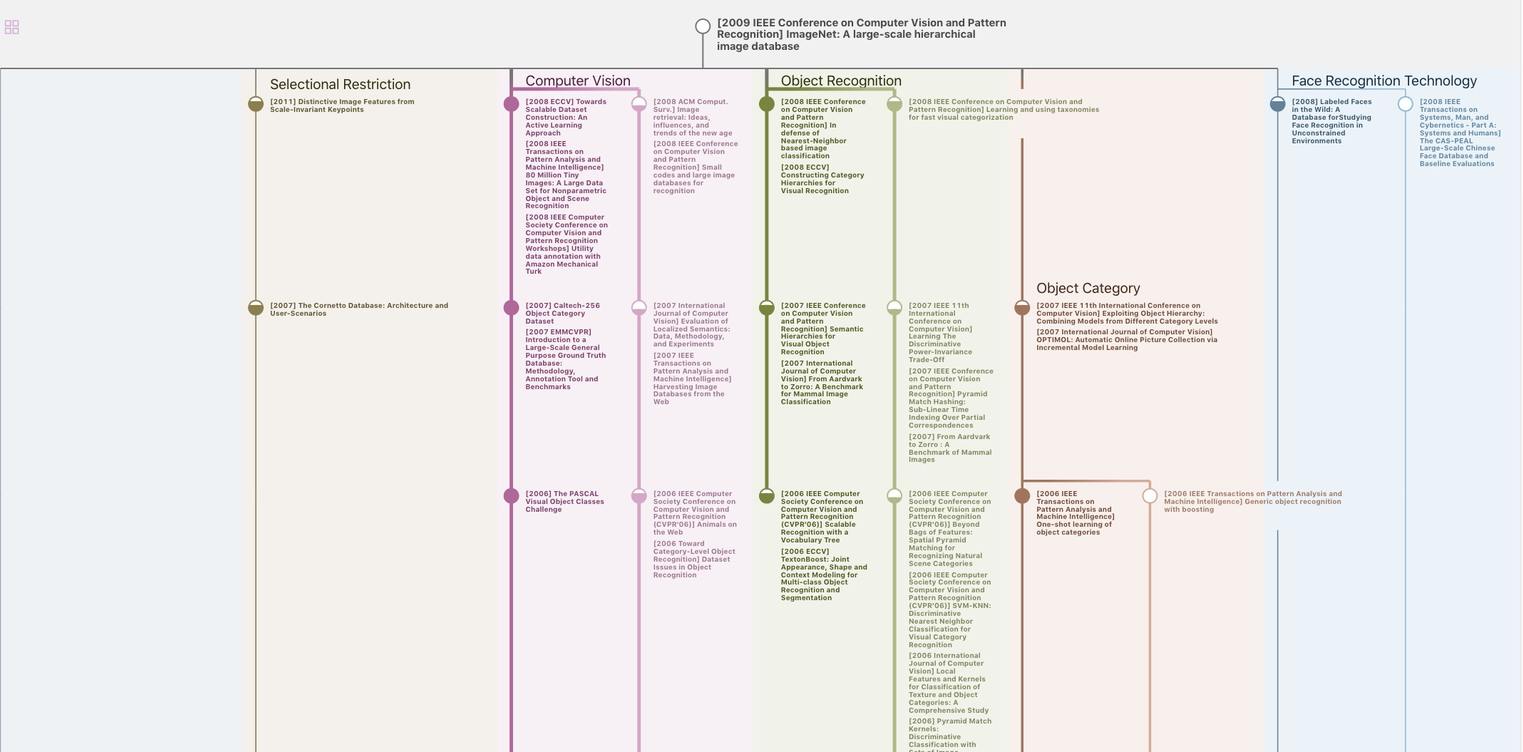
生成溯源树,研究论文发展脉络
Chat Paper
正在生成论文摘要