Person Re-Identification Based On Multi-Parts Of Local Feature Network
IEEE ACCESS(2019)
摘要
Person re-identification has become increasing popular because of its widely application in computer vision. In this paper, we propose a novel, simple and efficient person re-id network called MPLFN. The network combines two tasks : the classification task and the metric learning task. In the classification task, we uniformly partition N feature parts from an image, and compute the person classification loss in each part separately. Computing the part loss separately guides the network to focus on every body part and learn discriminative representations for each of them. And then in the metric learning task, we recalculate the distance of two images by the shortest path between two sets of feature parts. Then the distances are put into a triplet loss to perform a dynamic part alignment during the training. With the joint learning of these two tasks, the performance of the network is significantly enhanced. Compared with existing person re-id works, MPLFN achieves a better performance on three mainstream person re-identification datasets. Extensive experiments have been conducted to validate our proposed method.
更多查看译文
关键词
Person re-identification, part loss, metric learning, alignment
AI 理解论文
溯源树
样例
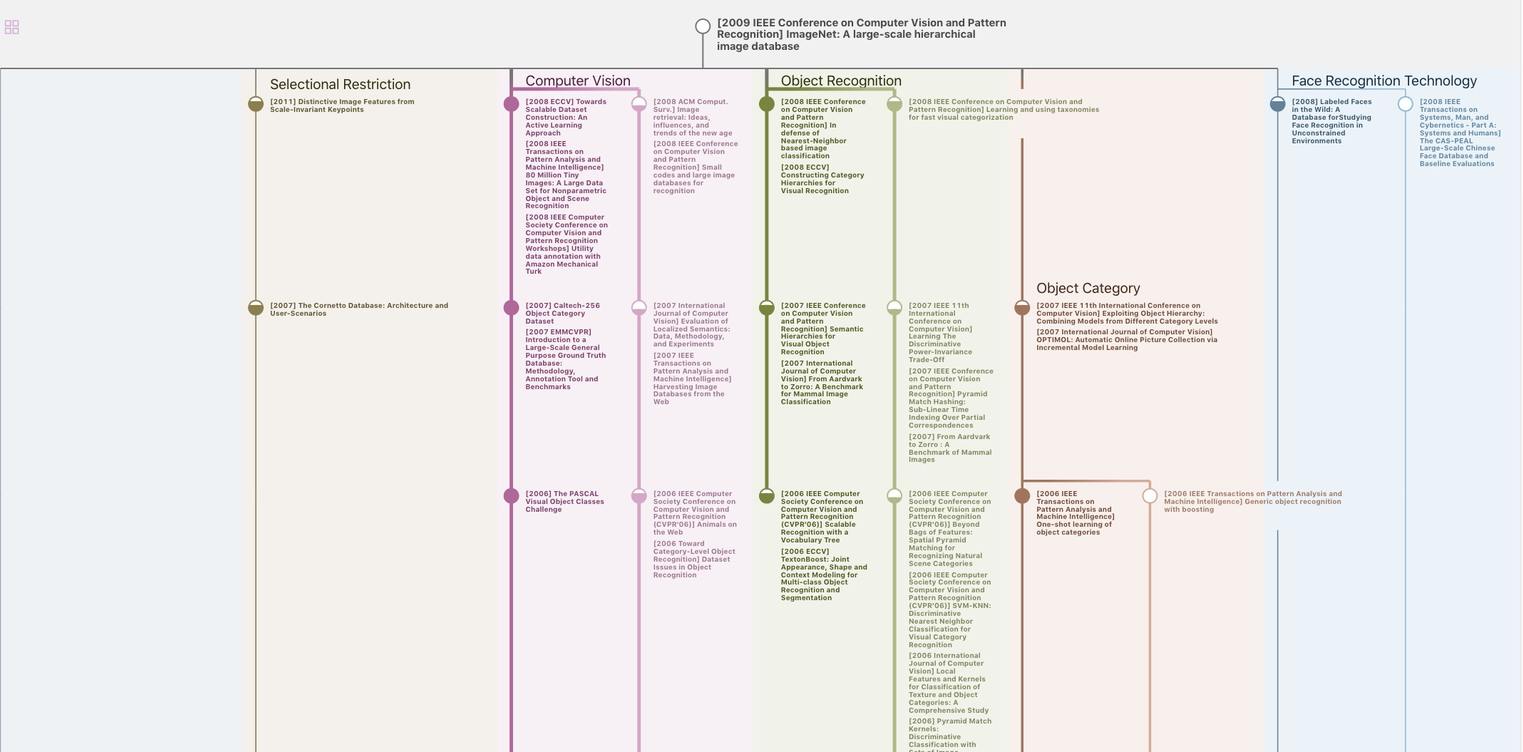
生成溯源树,研究论文发展脉络
Chat Paper
正在生成论文摘要