Self-Attention for Speech Emotion Recognition
INTERSPEECH(2019)
摘要
Speech Emotion Recognition (SER) has been shown to benefit from many of the recent advances in deep learning, including recurrent based and attention based neural network architectures as well. Nevertheless, performance still falls short of that of humans. In this work, we investigate whether SER could benefit from the self-attention and global windowing of the transformer model. We show on the IEMOCAP database that this is indeed the case. Finally, we investigate whether using the distribution of, possibly conflicting, annotations in the training data, as soft targets could outperform a majority voting. We prove that this performance increases with the agreement level of the annotators.
更多查看译文
关键词
speech emotion recognition, self-attention, global windowing
AI 理解论文
溯源树
样例
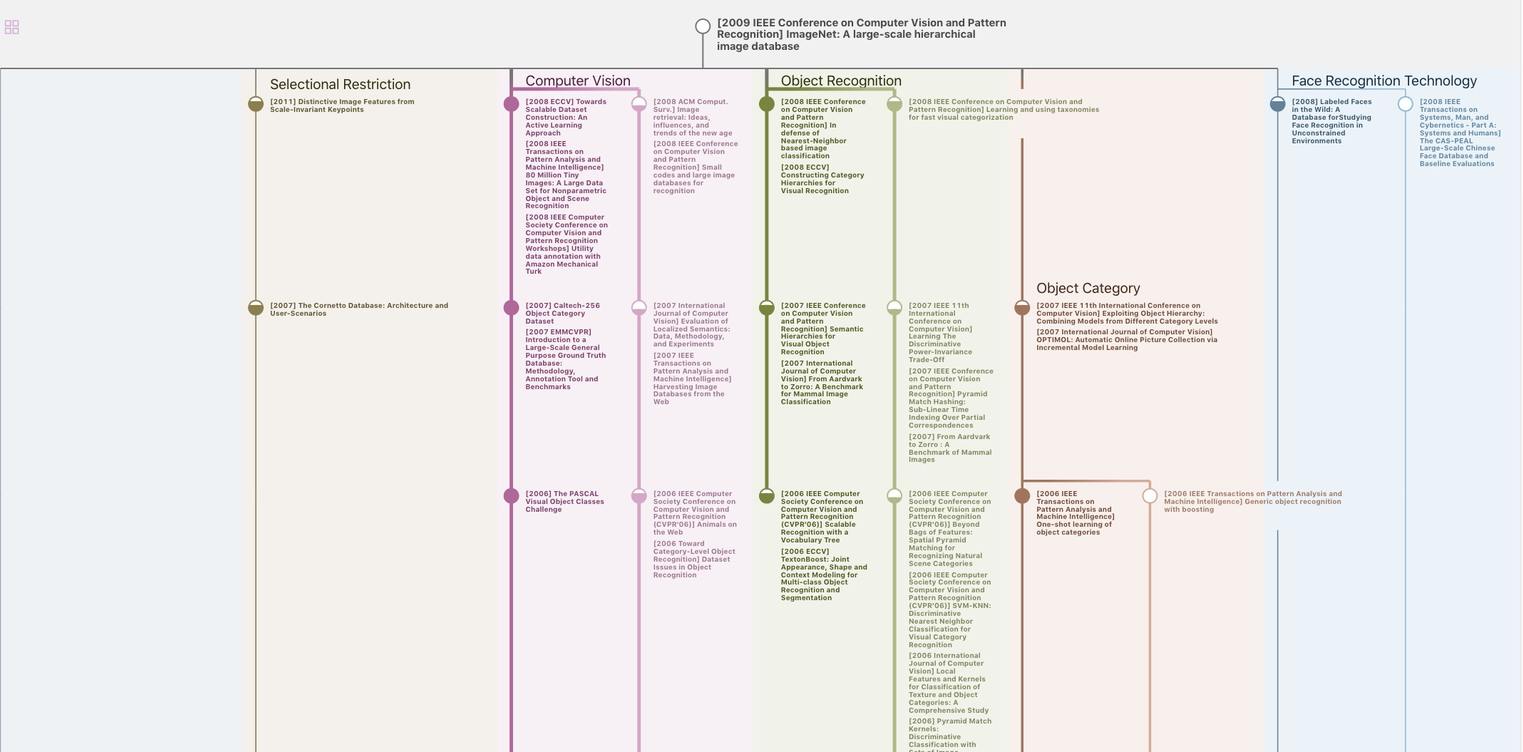
生成溯源树,研究论文发展脉络
Chat Paper
正在生成论文摘要