One-Shot Voice Conversion with Disentangled Representations by Leveraging Phonetic Posteriorgrams
INTERSPEECH(2019)
摘要
We propose voice conversion model from arbitrary source speaker to arbitrary target speaker with disentangled representations. Voice conversion is a task to convert the voice of spoken utterance of source speaker to that of target speaker. Most prior work require to know either source speaker or target speaker or both in training, with either parallel or non-parallel corpus. Instead, we study the problem of voice conversion in nonparallel speech corpora and one-shot learning setting. We convert an arbitrary sentences of an arbitrary source speaker to target speakers given only one or few target speaker training utterances. To achieve this, we propose to use disentangled representations of speaker identity and linguistic context. We use a recurrent neural network (RNN) encoder for speaker embedding and phonetic posteriorgram as linguistic context encoding, along with a RNN decoder to generate converted utterances. Ours is a simpler model without adversarial training or hierarchical model design and thus more efficient. In the subjective tests, our approach achieved significantly better results compared to baseline regarding similarity.
更多查看译文
关键词
voice conversion, disentangled representations, phonetic posteriorgrams
AI 理解论文
溯源树
样例
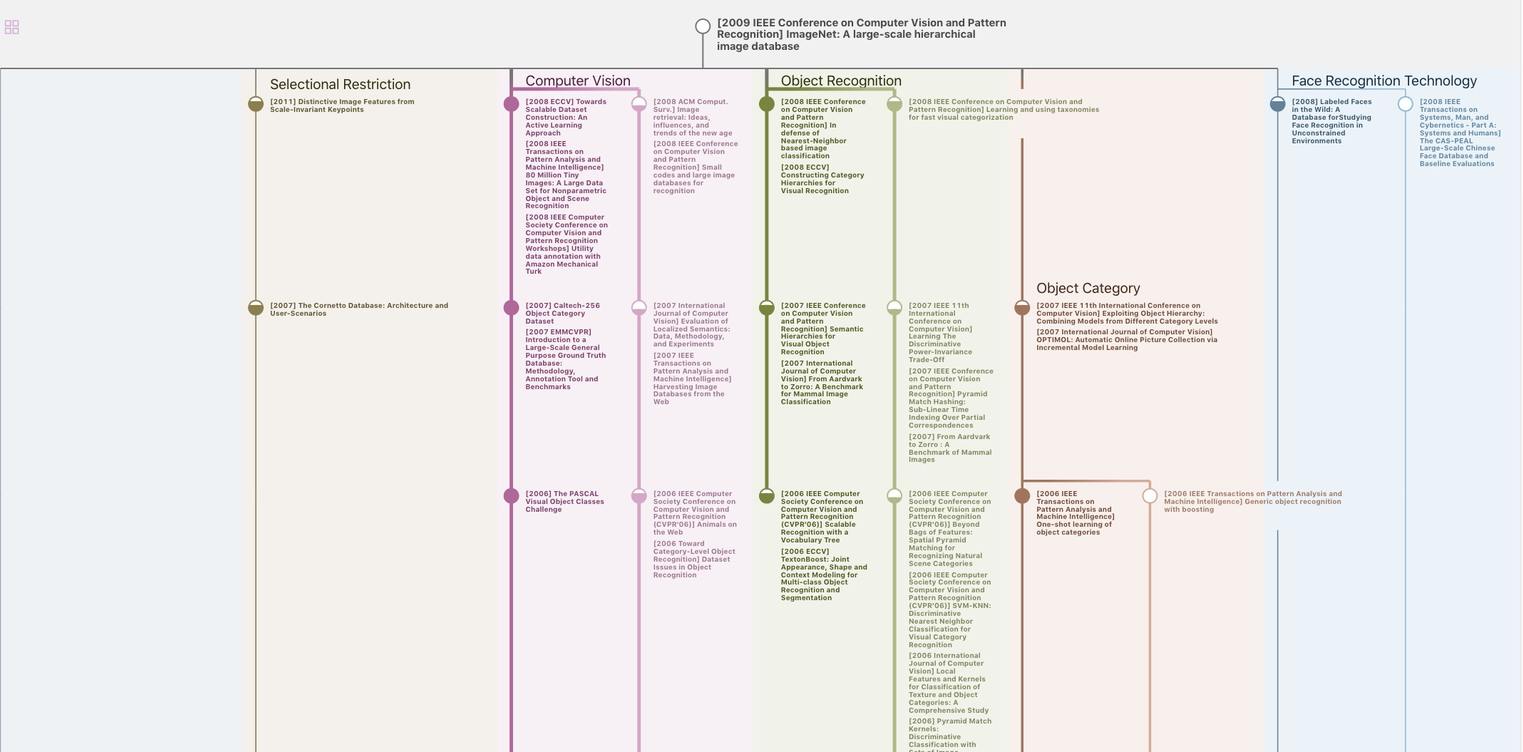
生成溯源树,研究论文发展脉络
Chat Paper
正在生成论文摘要