Breast Cancer Prediction using Feature Selection and Ensemble Voting
2019 International Conference on System Science and Engineering (ICSSE)(2019)
摘要
Breast cancer is the most common cause of cancer among women worldwide. This paper analyses the performance of supervised and unsupervised models for breast cancer classification. Data from Wisconsin Breast Cancer Dataset is used in this paper. Feature selection is processed through scaling and principal component analysis. Final results indicate that Ensemble Voting approach is ideal as a predictive model for breast cancer. The raw data has 569 cases of breast cancer. The data is split into training and testing sets in the ration 70:30, respectively. The benchmark model is then created using Random Forest method. Various models are trained and tested on the data after Feature Scaling and Principle Component Analysis. Cross-validation is performed which showed that our model is stable. Among all the evaluated models, only four models, i.e., Ensemble - Voting Classifier, Logistics Regression, SVM Tuning and AdaBoost returned with accuracy of at least 98%. Based on results of the precision and recall, ROC-AVC, Fl-measure and computational time of the models, the Ensemble showed the most potential in breast cancer classification of the given dataset.
更多查看译文
关键词
Breast Cancer,Ensemble Voting Classification,SVM,Random Forest,Perception,Logistics Regression,KNN,Stochastic Gradient Descent,XGBoost,Extremely Randomised Trees,AdaBoost
AI 理解论文
溯源树
样例
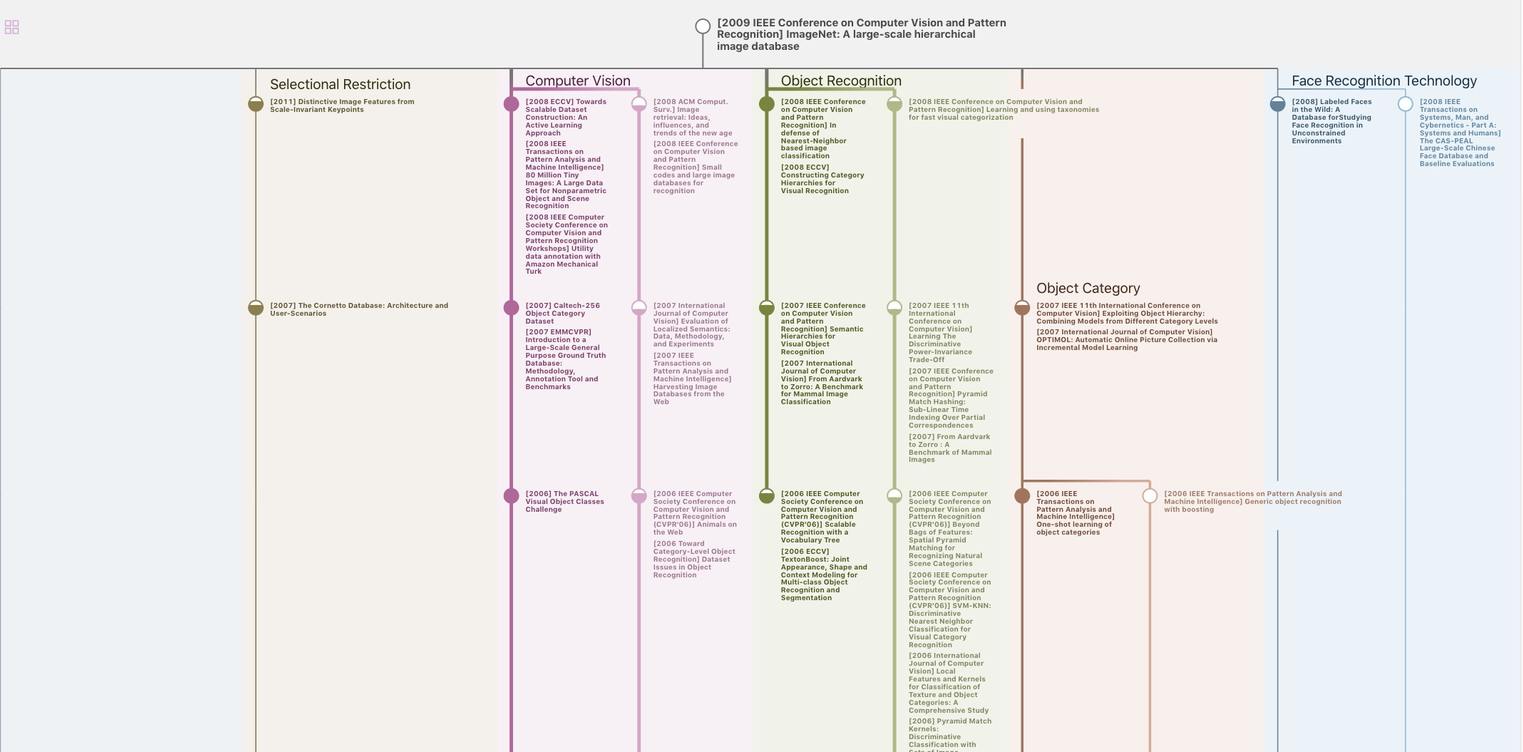
生成溯源树,研究论文发展脉络
Chat Paper
正在生成论文摘要