On Simulation Knowledge Acquisition Using Gaussian Processes For The Design Of Electric Motors
29TH CIRP DESIGN CONFERENCE 2019(2019)
摘要
In the automotive industry, high efforts are made to design high-efficiency, low-cost, requirement-optimized electric drives. Traditionally, time-consuming and expensive 3D finite element analyses are used to assess the effects of different design parameters from various engineering disciplines such as the electromagnetics, heat transfer, and mechanics. Often, to decrease the calculation efforts, scalable, physics-based reduced order models are used. For setting up and interpreting such reduced order models, simulation experts employ implicit knowledge by applying heuristic correction factors. This paper proposes a novel method to formalizing such implicit simulation knowledge using Gaussian Processes. Furthermore, the successful application of the method to a case study of electric motors is reported by validating the aforementioned correction factors for different motor designs. (C) 2019 The Authors. Published by Elsevier B.V.
更多查看译文
关键词
Knowledge Acquisition, Meta-Modelling, Gaussian Process, Electric Motor, Case Study
AI 理解论文
溯源树
样例
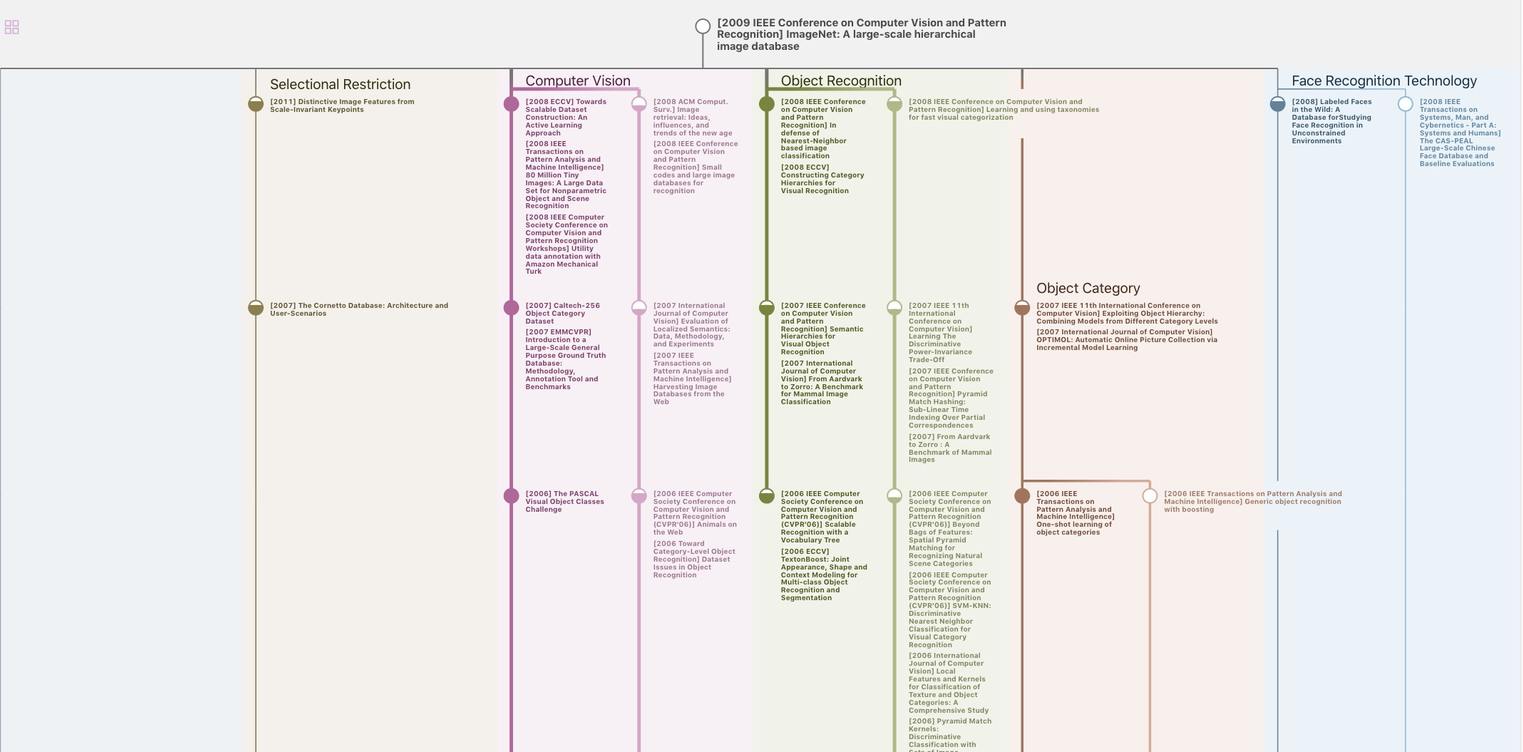
生成溯源树,研究论文发展脉络
Chat Paper
正在生成论文摘要