Identification Of Brain Functional Networks Using A Model-Based Approach
INTERNATIONAL JOURNAL OF PATTERN RECOGNITION AND ARTIFICIAL INTELLIGENCE(2020)
摘要
Functional MRI (fMRI) is a valuable brain imaging technique. A significant problem, when analyzing fMRI time series, is the finding of functional brain networks when the brain is at rest, i.e. no external stimulus is applied to the subject. In this work, we present a probabilistic method to estimate the Resting State Networks (RSNs) using a model-based approach. More specifically, RSNs are assumed to be the result of a clustering procedure. In order to perform the clustering, a mixture of regression models are used. Furthermore, special care has been given in order to incorporate important functionalities, such as spatial and embedded sparsity enforcing properties, through the use of informative priors over the model parameters. Another interesting feature of the proposed scheme is the flexibility to handle all the brain time series at once, allowing more robust solutions. We provide comparative experimental results, using an artificial fMRI dataset and two real resting state fMRI datasets, that empirically illustrate the efficiency of the proposed regression mixture model.
更多查看译文
关键词
FMRI data analysis, Resting State Networks, regression mixture models, MRF prior, sparseness
AI 理解论文
溯源树
样例
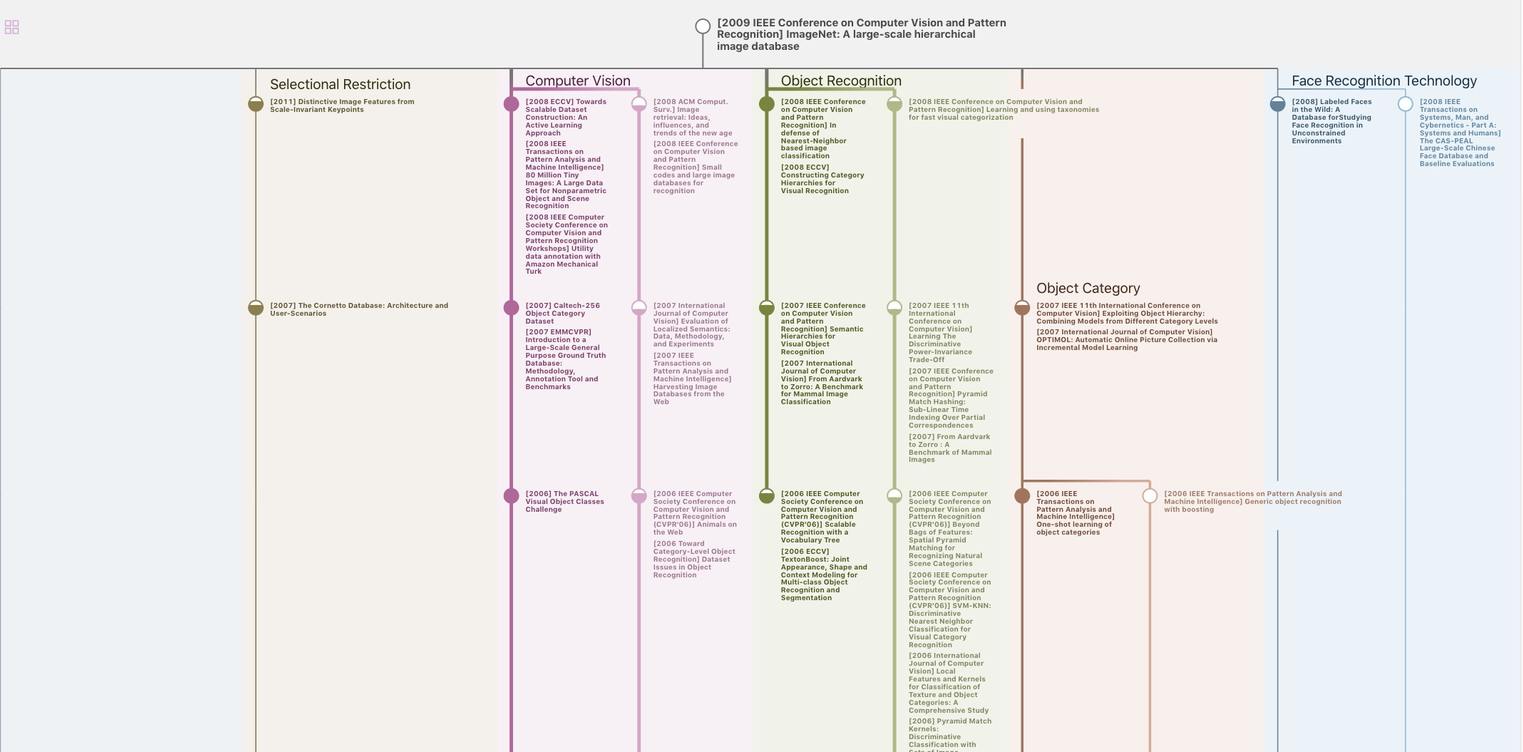
生成溯源树,研究论文发展脉络
Chat Paper
正在生成论文摘要