Neighbors-Based Graph Construction For Dimensionality Reduction
IEEE ACCESS(2019)
摘要
Dimensionality reduction is a fundamental task in the field of data mining and machine learning. In many scenes, examples in high-dimensional space usually lie on low-dimensional manifolds; thus, learning the low-dimensional embedding is important. Some well-known methods, such as LPP and LE, adopt a locality-preserving strategy by constructing an adjacent graph and using the graph Laplacian to project raw examples into subspace in order to obtain the low-dimensional representation. Accordingly, in this paper, we propose a novel neighbors-based distance that measures the distance of two examples through their neighbors. To be more specific, we create a virtual bridge point from the neighbors of each example and use it to link with others. Instead of computing their direct Euclidean distance, we derive the distance of any two examples using their bridge points. We note that the introduced metric shows a high discriminative ability for the examples on the boundary, which known to be infamously hard examples. Extensive experiments on classification and clustering demonstrate that our proposed graph construction method can achieve a large margin improvement in spite of its simple form.
更多查看译文
关键词
Dimensionality reduction, graph, metric, manifold learning
AI 理解论文
溯源树
样例
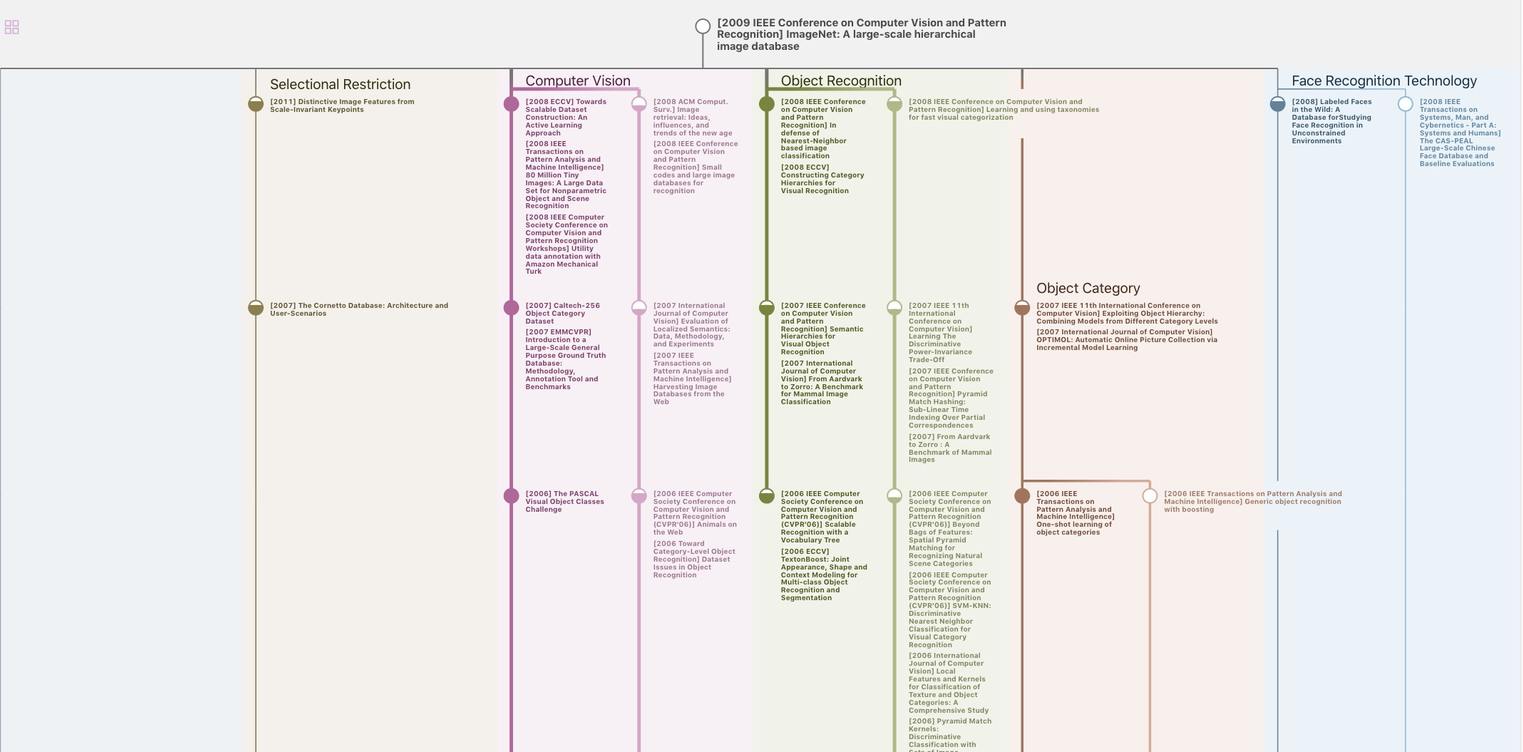
生成溯源树,研究论文发展脉络
Chat Paper
正在生成论文摘要