Evaluation Of Quantitative Imaging Markers From F-18-Fdg Pet/Ct For Treatment Response Evaluation In Pediatric Patients With Hodgkin Lymphoma
JOURNAL OF NUCLEAR MEDICINE(2018)
摘要
23 Objectives: To evaluate textural features from baseline PET/CT as possible predictors of treatment outcomes evaluated by visual criteria such as Deauville scale.
Methods
Twenty one newly diagnosed patients with Hodgkin Lymphoma (HL) were enrolled in this study. Patients underwent 18F-FDG PET/CT scans for initial disease staging (baseline bPET), and at end of two cycles of chemotherapy (interim iPET). In the patient cohort we had 8 male and 13 female, median age was 12 years (range 6-17). PET/CT based stages were I (n=2), II (n=7), III (n=10) and IV (n=2). For histopathology we had: lymphocyte rich (LR, n=3), mixed cellularity (MC, n=10) and nodular sclerosis (NS, n=8). Chemotherapy protocols used were ABVE (n=13) and BEACOP (n=8). Lesions were segmented visually using bPET scans. Patients were assigned, after iPET according to Deauville criteria, to five different scores (1 to 4 and X). We calculated 50 different texture features for each lesion, including first, second and higher order features. Lesions from all patients having an extent of 5 or more voxels were selected for training and evaluating supervised classification models using texture features as classifiers. Lesions were classified following two different criteria: patients Deauville classification and histopathology. For a single patient, all lesions were assigned the same Deauville score and histopathology. Accuracy of the trained classifiers was evaluated using a 5 fold cross-validation scheme. Receiver operating curves (ROC) were used to evaluate discriminator quality using the area under the curve (AUC) value.
Results
For histopathology type classification, we selected 105 (57 MC, 45 NS and 3 LR) lesions and obtained a 76.2% accuracy using a medium Gaussian support vector machine classifier and principal component analysis keeping 12 principal components. True positive rates (TPR) for MC, NS and LR are respectively: 88%, 67%, 0%. Positive predictive values (PPV) for MS and NS are respectively: 74%, 81%. The easiest type to discriminate from the others is MC with an AUC of 0.82 for our model.
For Deauville scores 1 to 4 (D1-D4) we selected 88 lesions (3 D1, 39 D2, 38 D3 and 8 D4). We obtained a 68.2% accuracy using a medium K nearest neighbors classifier and 4 from 50 different textural features. TPR for D1-4 are respectively: 0%, 85%, 71%, 0%. PPV for D2-3 are respectively: 62%, 77%. Using this model the easiest Deauville score to discriminate from the others was score 3, with an AUC of 0.81.
Conclusions
We successfully used textural features calculated from baseline 18F-FDG PET/CT scans to classify patients according to Deauville criteria and to classify lesions according to histopathology with high accuracy. Studies with larger samples are needed to verify these findings.
更多查看译文
AI 理解论文
溯源树
样例
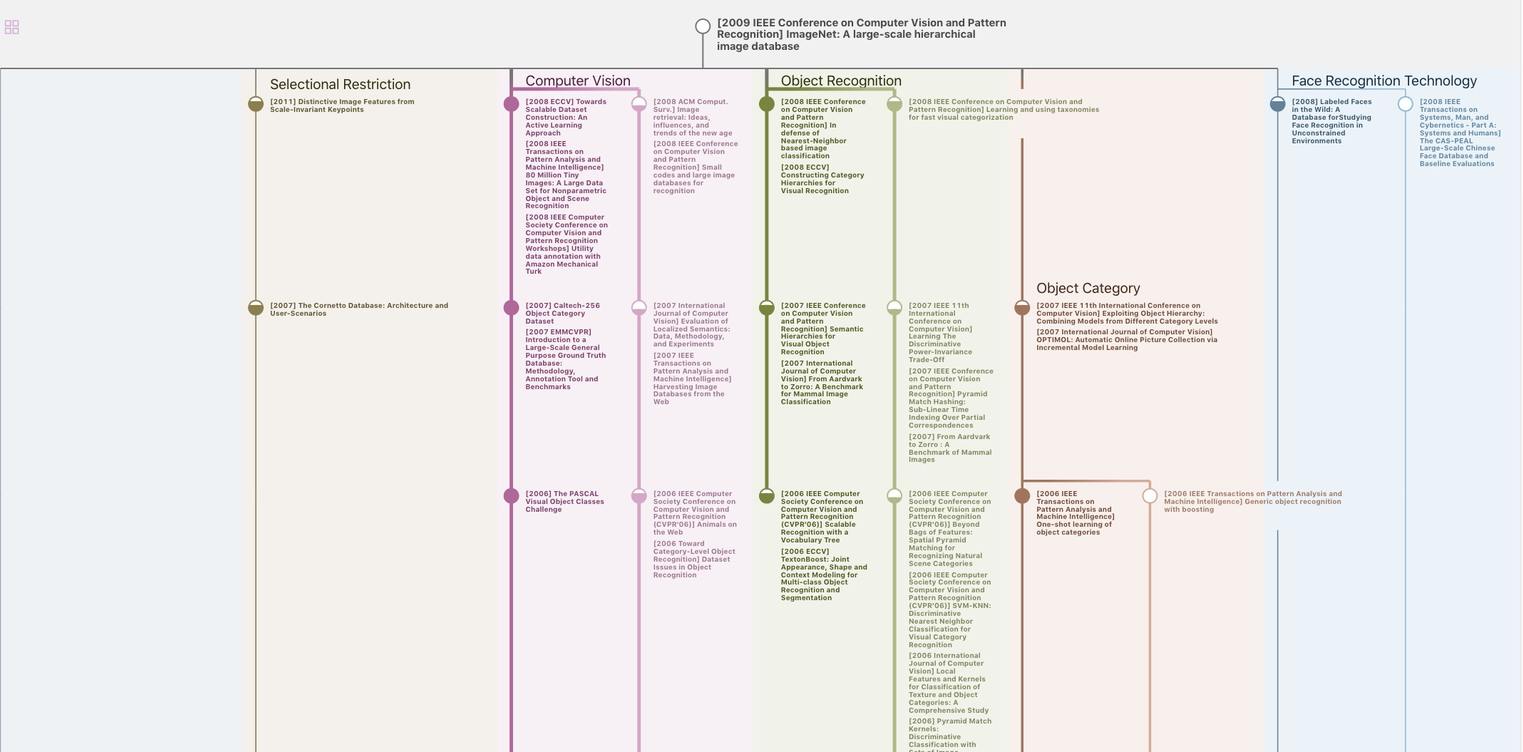
生成溯源树,研究论文发展脉络
Chat Paper
正在生成论文摘要