Sparse-View CT Reconstruction via Generative Adversarial Networks
2018 IEEE Nuclear Science Symposium and Medical Imaging Conference Proceedings (NSS/MIC)(2018)
摘要
Low dose and sparse view CT are effective approaches to reduce the radiation dose and accelerate scan speed. Images reconstructed from insufficient data acquired from low dose and sparse view CT are associated with severe streaking artifacts. Therefore, reducing the radiation dose will further degrade the imaging quality. Several attempts have been made to remove these artifacts using deep learning methods such as CNN. Although the deep learning methods for low dose and sparse view CT reconstruction have gained impressive successes, the reconstruction results are still over-smooth. In this work, we propose an artifacts reduction method for low dose and sparse-view CT via a single model trained by generative adversarial networks (GAN). Several numerical simulation experiments are implemented to test the performance of our network. The results show that our GAN can significantly reduce the streaking atrifacts campared with FBP method, and carries more detailed information than CNN.
更多查看译文
关键词
low dose,sparse view,GAN
AI 理解论文
溯源树
样例
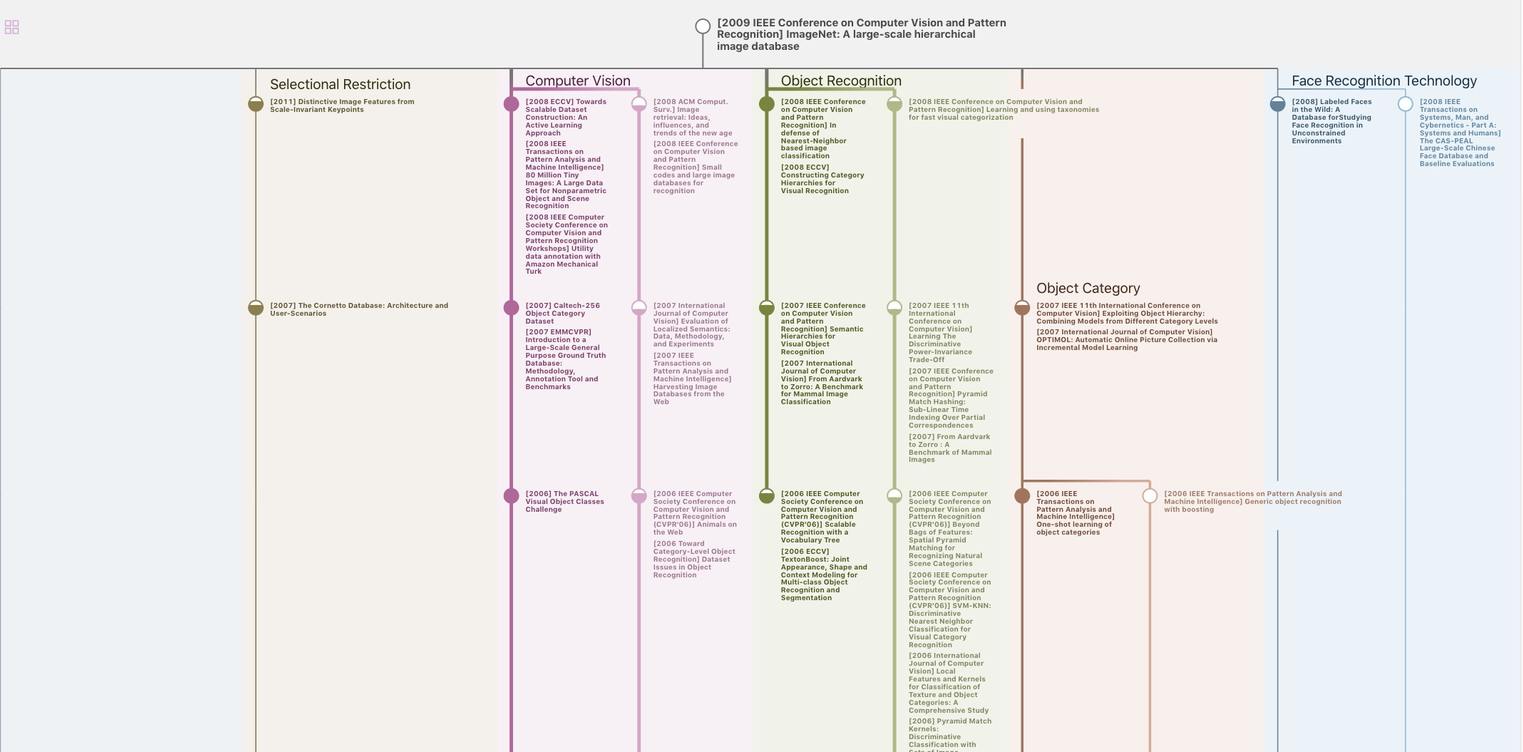
生成溯源树,研究论文发展脉络
Chat Paper
正在生成论文摘要