Multi-subspace supervised descent method for robust face alignment
Multimedia Tools and Applications(2019)
摘要
Supervised Descent Method (SDM) is one of the leading cascaded regression approaches for face alignment with state-of-the-art performance and a solid theoretical basis. However, SDM is prone to local optima and likely averages conflicting descent directions. This makes SDM ineffective in covering a complex facial shape space due to large head poses and rich non-rigid face deformations. In this paper, a novel two-step framework called multi-subspace SDM (MS-SDM) is proposed to equip SDM with a stronger capability for dealing with unconstrained faces. The optimization space is first partitioned with regard to shape variations using k-means. The generated subspaces show semantic significance which highly correlates with head poses. Faces among a certain subspace also show compatible shape-appearance relationships. Then, Naive Bayes is applied to conduct robust subspace prediction by concerning about the relative proximity of each subspace to the sample. This guarantees that each sample can be allocated to the most appropriate subspace-specific regressor. The proposed method is validated on benchmark face datasets with a mobile facial tracking implementation.
更多查看译文
关键词
Unconstrained face alignment, SDM, Subspace learning, Cascaded regression
AI 理解论文
溯源树
样例
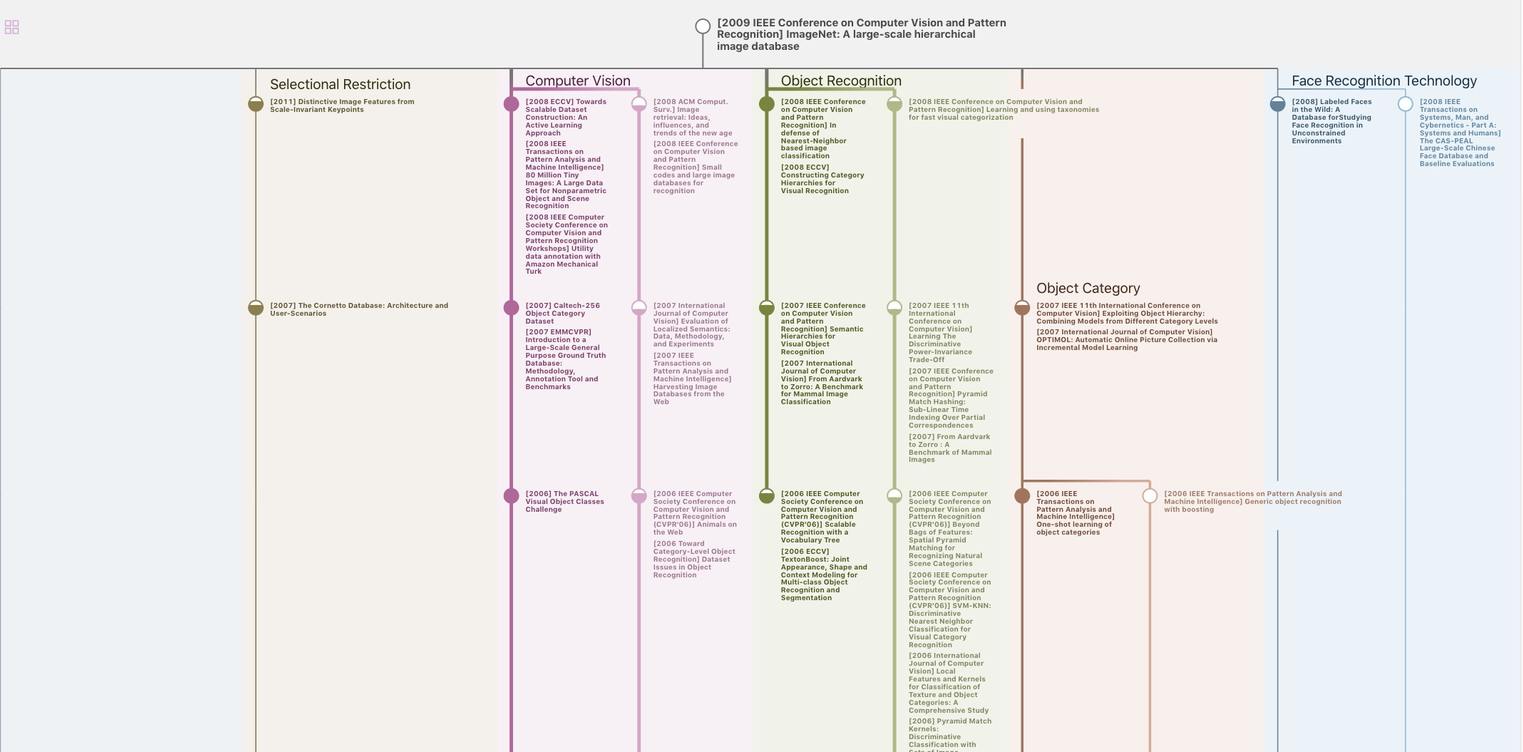
生成溯源树,研究论文发展脉络
Chat Paper
正在生成论文摘要