Automated Learning Of User Preferences For Selection Of High Quality 3d Designs
29TH CIRP DESIGN CONFERENCE 2019(2019)
摘要
3D object design is one of the most sought-after topics due to its application in many domains including Medical imaging, Virtual Reality, Computer Graphics, Animation etc. Manual 3D object design is inherently an expensive and labour intensive task. Several techniques such as GAN (Generative Adversarial Nets), SFM (Structure from Motion) and MVS (Multi-View Stereo) are employed to automate 3D design. 3D-GAN is one such method that allows the fully automated design of 3D objects using deep artificial neural networks. However, such generated 3D objects often suffer from distortions, obscure/missing parts, and other artefacts. For a large number of generated models, it becomes time-consuming for the human expert to select models of high quality among the set of generated ones. In this paper, we tackle this problem through machine learning powered 3D model quality assessment algorithm, that mimics the users preferences. For our experiments, we generate 3D objects using 3D-GAN and investigate several machine learning algorithms including Support Vector Machines, Gradient Boosting, Deep Neural Networks to classify generated models based on their visual appealability. We find that our model successfully mimics user choices with 90% accuracy, thus eliminating the need for human intervention in the subjective quality analysis, once user preferences have been learned. The proposed system can be seamlessly integrated into existing automated 3D design systems further augmenting the capabilities of human designers. (C) 2019 The Authors. Published by Elsevier B.V. Peer-review under responsibility of the scientific committee of the CIRP Design Conference 2019.
更多查看译文
关键词
Automated 3D Design, Artificial Intelligence, Quality Estimation, chine Learning
AI 理解论文
溯源树
样例
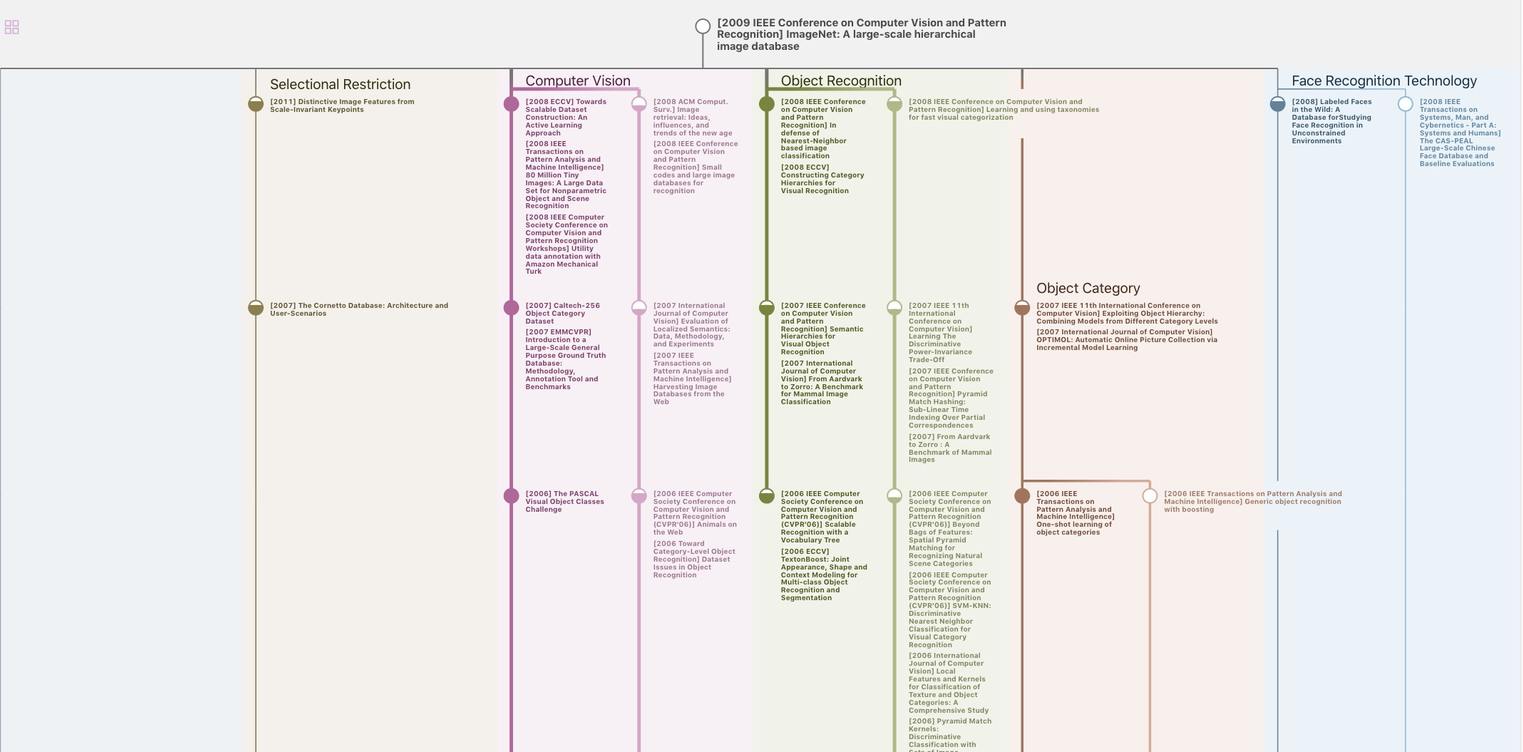
生成溯源树,研究论文发展脉络
Chat Paper
正在生成论文摘要