Catastrophic Forgetting Meets Negative Transfer: Batch Spectral Shrinkage for Safe Transfer Learning
ADVANCES IN NEURAL INFORMATION PROCESSING SYSTEMS 32 (NIPS 2019)(2019)
摘要
Before sufficient training data is available, fine-tuning neural networks pre-trained on large-scale datasets substantially outperforms training from random initialization. However, fine-tuning methods suffer from a dilemma across catastrophic forgetting and negative transfer. While several methods with explicit attempts to overcome catastrophic forgetting have been proposed, negative transfer is rarely delved into. In this paper, we launch an in-depth empirical investigation into negative transfer in fine-tuning and find that, for the weight parameters and feature representations, transferability of their spectral components is diverse. For safe transfer learning, we present Batch Spectral Shrinkage (BSS), a novel regularization approach to penalizing smaller singular values so that untransferable spectral components are suppressed. BSS is orthogonal to existing fine-tuning methods and is readily pluggable into them. Experimental results show that BSS can significantly enhance the performance of state-of-the-art methods, especially in few training data regime.
更多查看译文
关键词
catastrophic forgetting,negative transfer
AI 理解论文
溯源树
样例
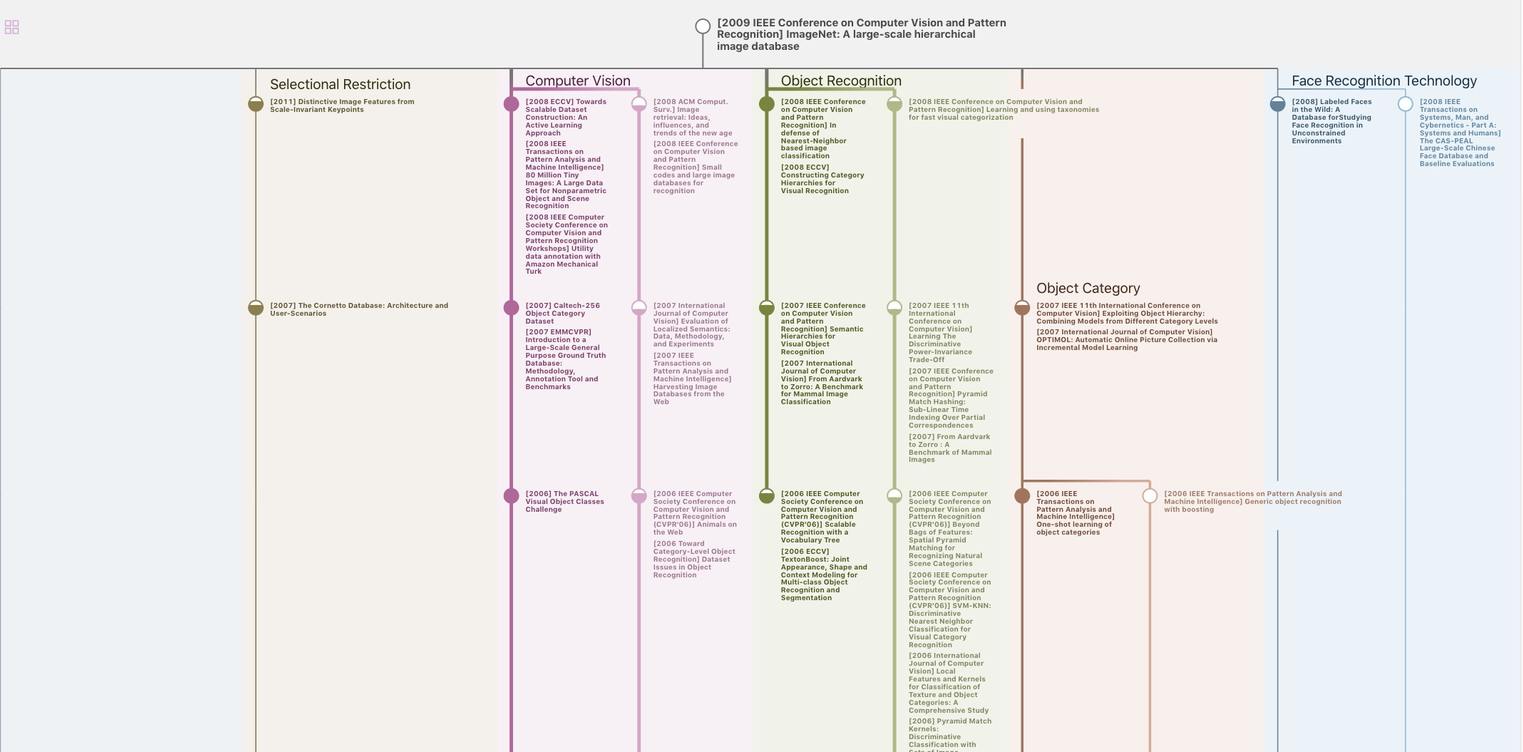
生成溯源树,研究论文发展脉络
Chat Paper
正在生成论文摘要