Effective approximation of parametrized closure systems over transactional data streams
Machine Learning(2019)
摘要
Strongly closed itemsets, defined by a parameterized closure operator, are a generalization of ordinary closed itemsets. Depending on the strength of closedness, the family of strongly closed itemsets typically forms a tiny subfamily of ordinary closed itemsets that is stable against changes in the input. In this paper we consider the problem of mining strongly closed itemsets from transactional data streams. Utilizing their algebraic and algorithmic properties, we propose an algorithm based on reservoir sampling for approximating this type of itemsets in the landmark streaming setting, prove its correctness, and show empirically that it yields a considerable speed-up over a straightforward naive algorithm without any significant loss in precision and recall. We motivate the problem setting considered by two practical applications. In particular, we first experimentally demonstrate that the above properties, i.e., compactness and stability, make strongly closed itemsets an excellent indicator of certain types of concept drifts in transactional data streams. As a second application we consider computer-aided product configuration, a real-world problem raised by an industrial project. For this problem, which is essentially exact concept identification, we propose a learning algorithm based on a certain type of subset queries formed by strongly closed itemsets and show on real-world datasets that it requires significantly less query evaluations than a naive algorithm based on membership queries.
更多查看译文
关键词
Data streams,Itemset mining,Closed itemsets,Concept drift detection,Applications
AI 理解论文
溯源树
样例
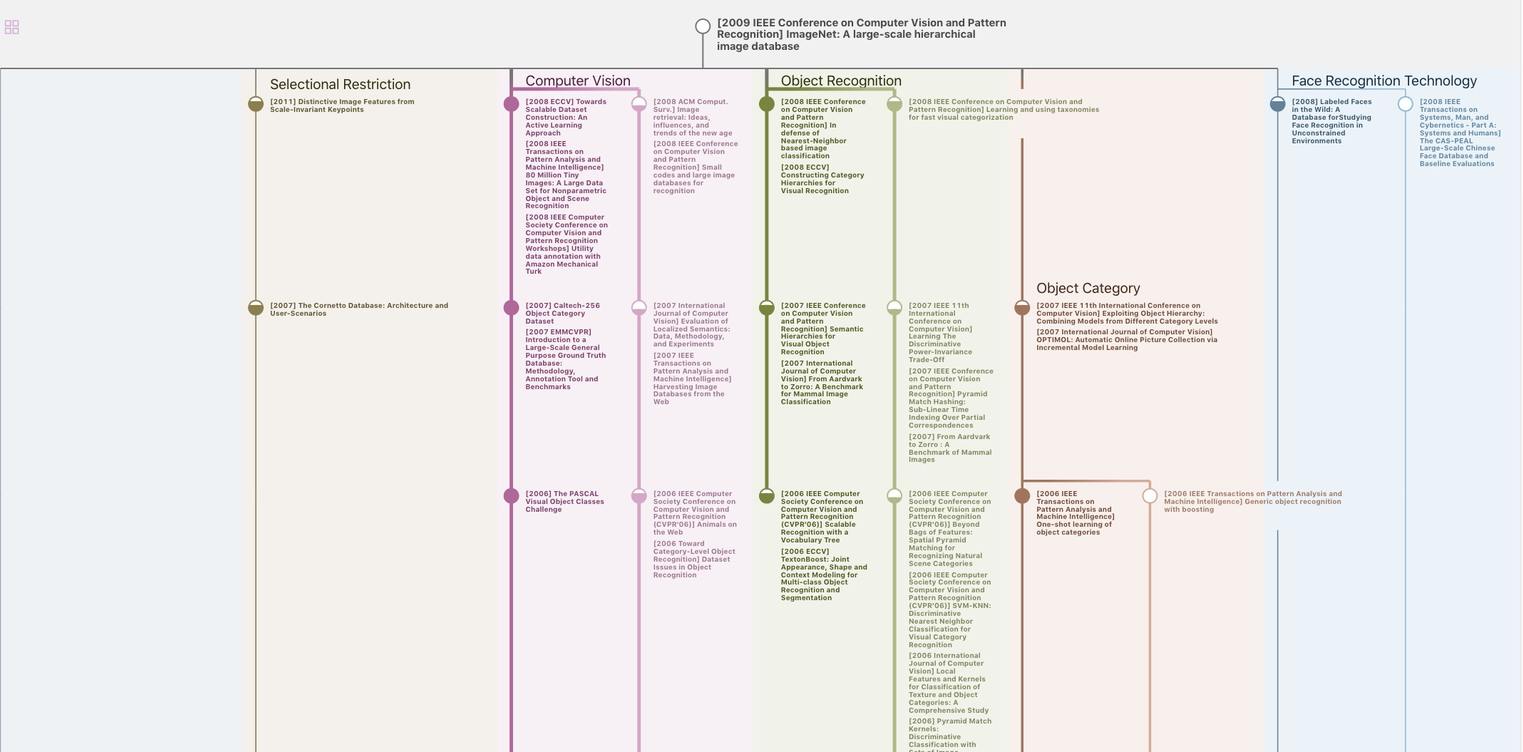
生成溯源树,研究论文发展脉络
Chat Paper
正在生成论文摘要