A Deep Learning Based Method for the Non-Destructive Measuring of Rock Strength through Hammering Sound
APPLIED SCIENCES-BASEL(2019)
摘要
Hammering rocks of different strengths can make different sounds. Geological engineers often use this method to approximate the strengths of rocks in geology surveys. This method is quick and convenient but subjective. Inspired by this problem, we present a new, non-destructive method for measuring the surface strengths of rocks based on deep neural network (DNN) and spectrogram analysis. All the hammering sounds are transformed into spectrograms firstly, and a clustering algorithm is presented to filter out the outliers of the spectrograms automatically. One of the most advanced image classification DNN, the Inception-ResNet-v2, is then re-trained with the spectrograms. The results show that the training accurate is up to 94.5%. Following this, three regression algorithms, including Support Vector Machine (SVM), K-Nearest Neighbor (KNN), and Random Forest (RF) are adopted to fit the relationship between the outputs of the DNN and the strength values. The tests show that KNN has the highest fitting accuracy, and SVM has the strongest generalization ability. The strengths (represented by rebound values) of almost all the samples can be predicted within an error of [ 5, 5]. Overall, the proposed method has great potential in supporting the implementation of efficient rock strength measurement methods in the field.
更多查看译文
关键词
transfer learning,spectrogram analysis,selecting samples,non-destructive testing,hammering sound,regression algorithm
AI 理解论文
溯源树
样例
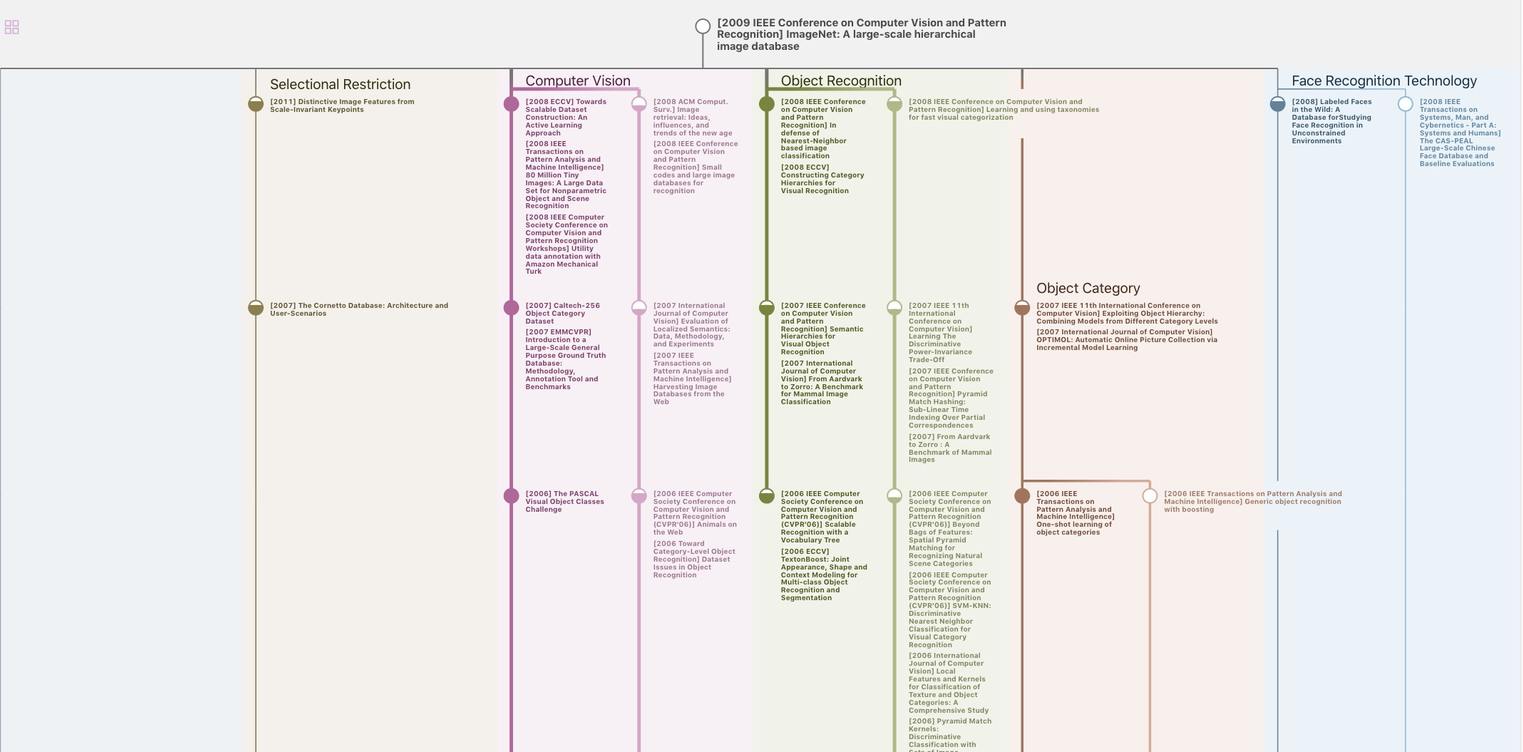
生成溯源树,研究论文发展脉络
Chat Paper
正在生成论文摘要