Reinforcement Learning For Cyber-Physical Security Assessment Of Power Systems
2019 IEEE MILAN POWERTECH(2019)
摘要
The protection of power systems is of paramount significance for the supply of electricity. Contingency analysis allows to access the impact of power grid components failures. Typically, power systems are designed to handle N - 2 contingencies. Existing algorithms mainly focus on performance and computational efficiency. There has been little effort in designing contingency methods from a cybersecurity perspective. To address this limitation, we study contingency analysis in the context of power system planning and operation towards cyber-physical security assessment. The proposed methodology considers attackers transitions in the network based on the N- 2 critical contingency pairs. We develop an online reinforcement Q-learning scheme to solve a Markov decision process that models adversarial actions. In this model, the adversary aims to maximize the cumulative reward before making any action and learns adaptively how to optimize the attack strategies. We validate and test the algorithm on eleven literature-based and synthetic power grid test cases.
更多查看译文
关键词
Contingency analysis, cybersecurity, power systems security, reinforcement learning
AI 理解论文
溯源树
样例
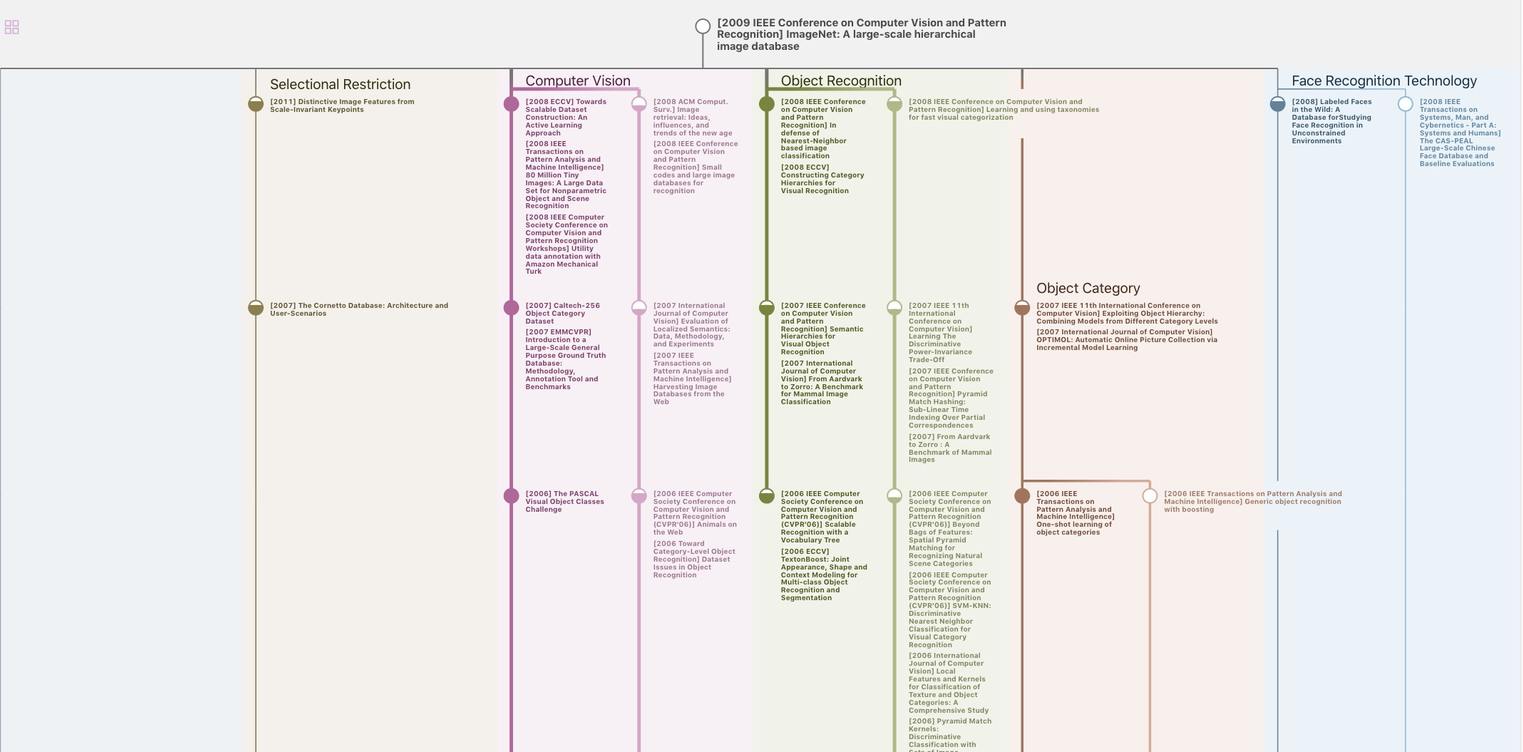
生成溯源树,研究论文发展脉络
Chat Paper
正在生成论文摘要