Tensor-Factorization-Based 3d Single Image Super-Resolution With Semi-Blind Point Spread Function Estimation
2019 IEEE INTERNATIONAL CONFERENCE ON IMAGE PROCESSING (ICIP)(2019)
摘要
A volumetric non-blind single image super-resolution technique using tensor factorization has been recently introduced by our group. That method allowed a 2-order-of-magnitude faster high-resolution image reconstruction with equivalent image quality compared to state-of-the-art algorithms. In this work a joint alternating recovery of the high-resolution image and of the unknown point spread function parameters is proposed. The method is evaluated on dental computed to-mography images. The algorithm was compared to an existing 3D super-resolution method using low-rank and total variation regularization, combined with the same alternating PSF-optimization. The two algorithms have shown similar improvement in PSNR, but our method converged roughly 40 times faster, under 6 minutes both in simulation and on experimental dental computed tomography data.
更多查看译文
关键词
image enhancement, 3D super-resolution, semi-blind deconvolution, 3D computed tomography
AI 理解论文
溯源树
样例
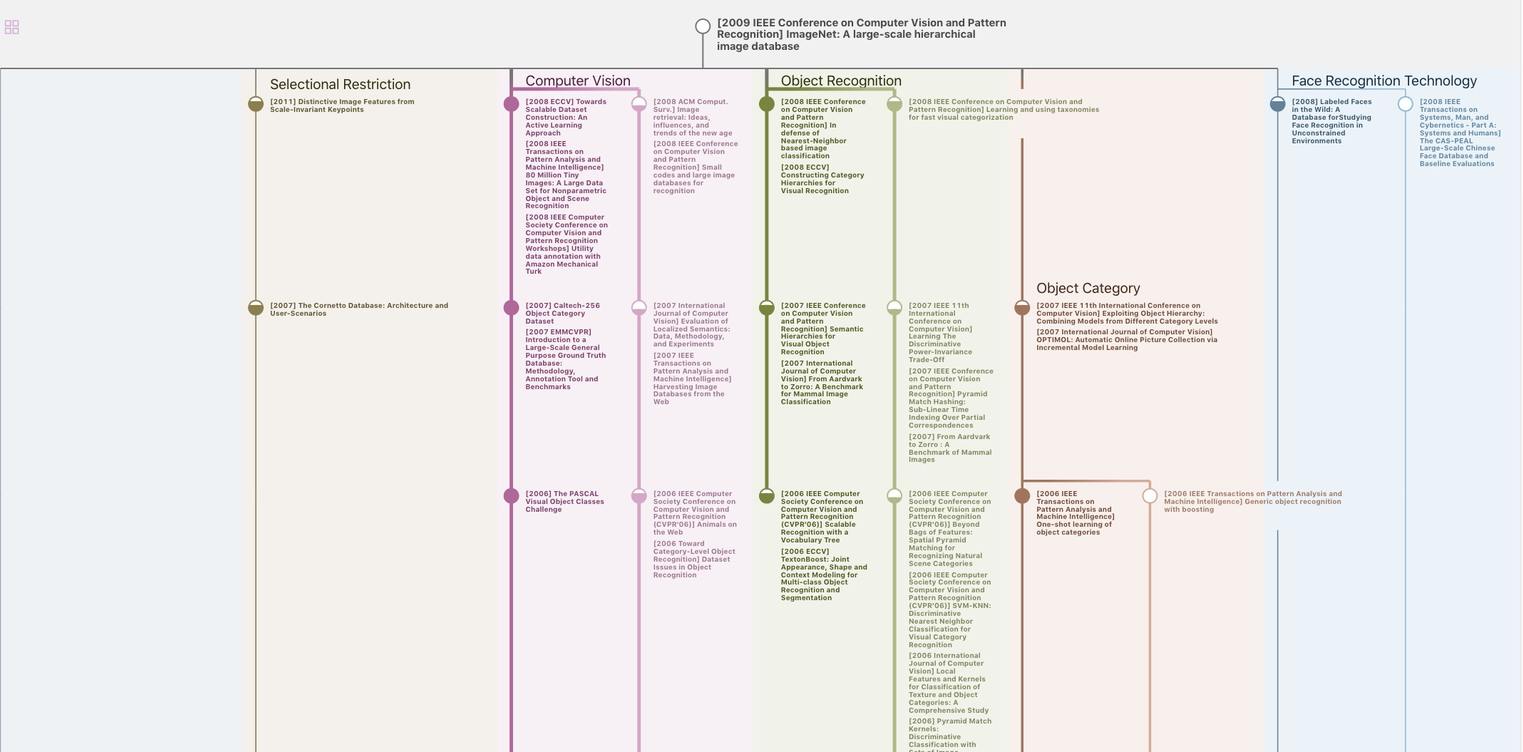
生成溯源树,研究论文发展脉络
Chat Paper
正在生成论文摘要