On Making Stochastic Classifiers Deterministic
NeurIPS(2019)
摘要
Stochastic classifiers arise in a number of machine learning problems, and have become especially prominent of late, as they often result from constrained optimization problems, e.g. for fairness, churn, or custom losses. Despite their utility, the inherent randomness of stochastic classifiers may cause them to be problematic to use in practice for a variety of practical reasons. In this paper, we attempt to answer the theoretical question of how well a stochastic classifier can be approximated by a deterministic one, and compare several different approaches, proving lower and upper bounds. We also experimentally investigate the pros and cons of these methods, not only in regard to how successfully each deterministic classifier approximates the original stochastic classifier, but also in terms of how well each addresses the other issues that can make stochastic classifiers undesirable.
更多查看译文
关键词
lower and upper bounds
AI 理解论文
溯源树
样例
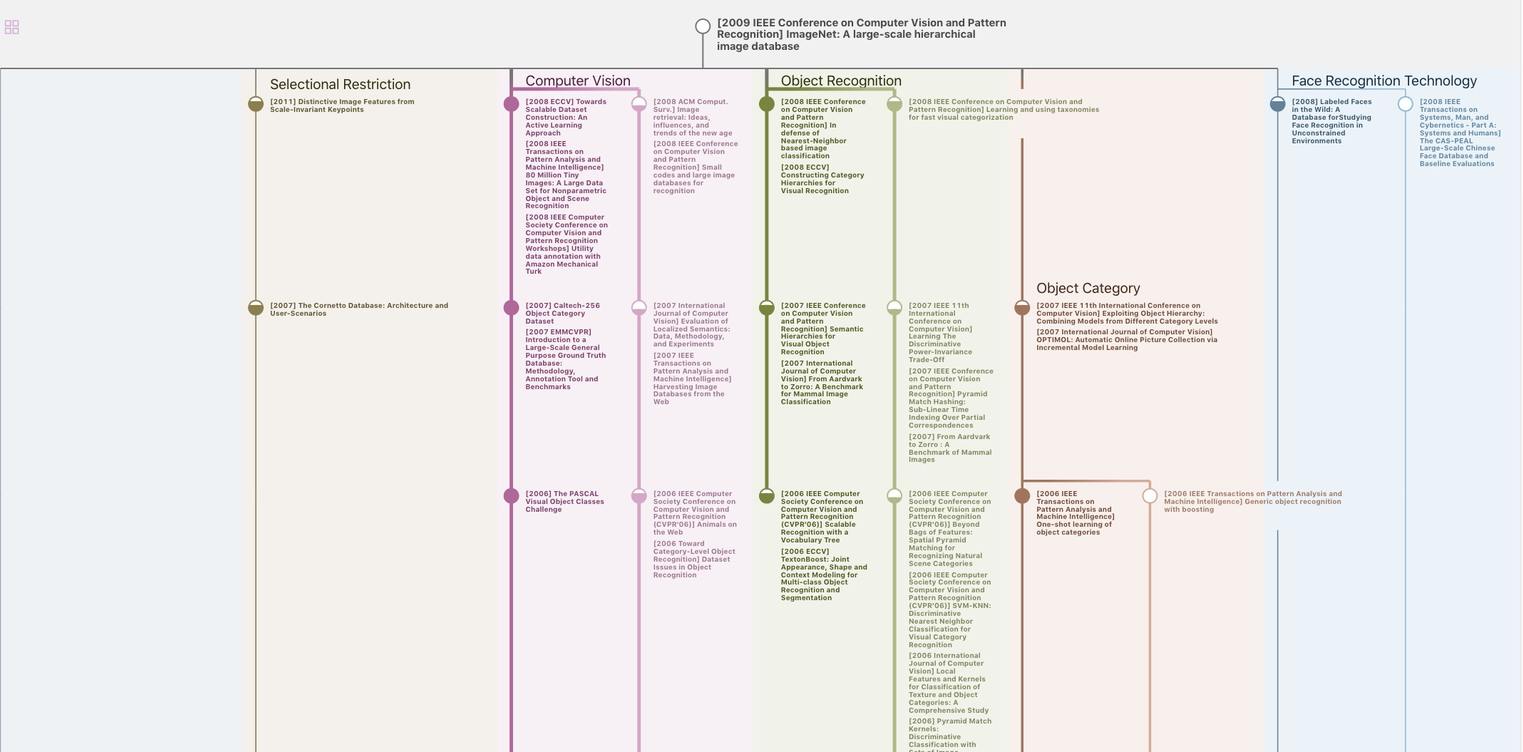
生成溯源树,研究论文发展脉络
Chat Paper
正在生成论文摘要