Person Re-Identification By Ranking Ensemble Representations
2019 IEEE INTERNATIONAL CONFERENCE ON IMAGE PROCESSING (ICIP)(2019)
摘要
Existing deep learning algorithms for person re-identification (re-id) typically rely on single-sample classification or pair-wise matching constraints. This indicates a breach of deployment due to ignoring the probe-specific matching information against the gallery set encoded in ranking lists. In this work, we address this problem by exploring the idea of RANkinG Ensembles (RANGE) that learns such information from the ranking lists. Specifically, given an off-the-self deep re-id feature representation model, we construct per-probe ranking lists and exploit them to learn inter ranking ensemble representation. To mitigate the harm of inevitable false gallery positives, we further introduce a complementary intra ranking ensemble representation. Extensive experiments show that both supervised and unsupervised re-id benefit from the proposed RANGE method on four challenging benchmarks: MSMT17, Market-1501, DukeMTMC-ReID, and CUHK03.
更多查看译文
关键词
Person re-identification, ranking list
AI 理解论文
溯源树
样例
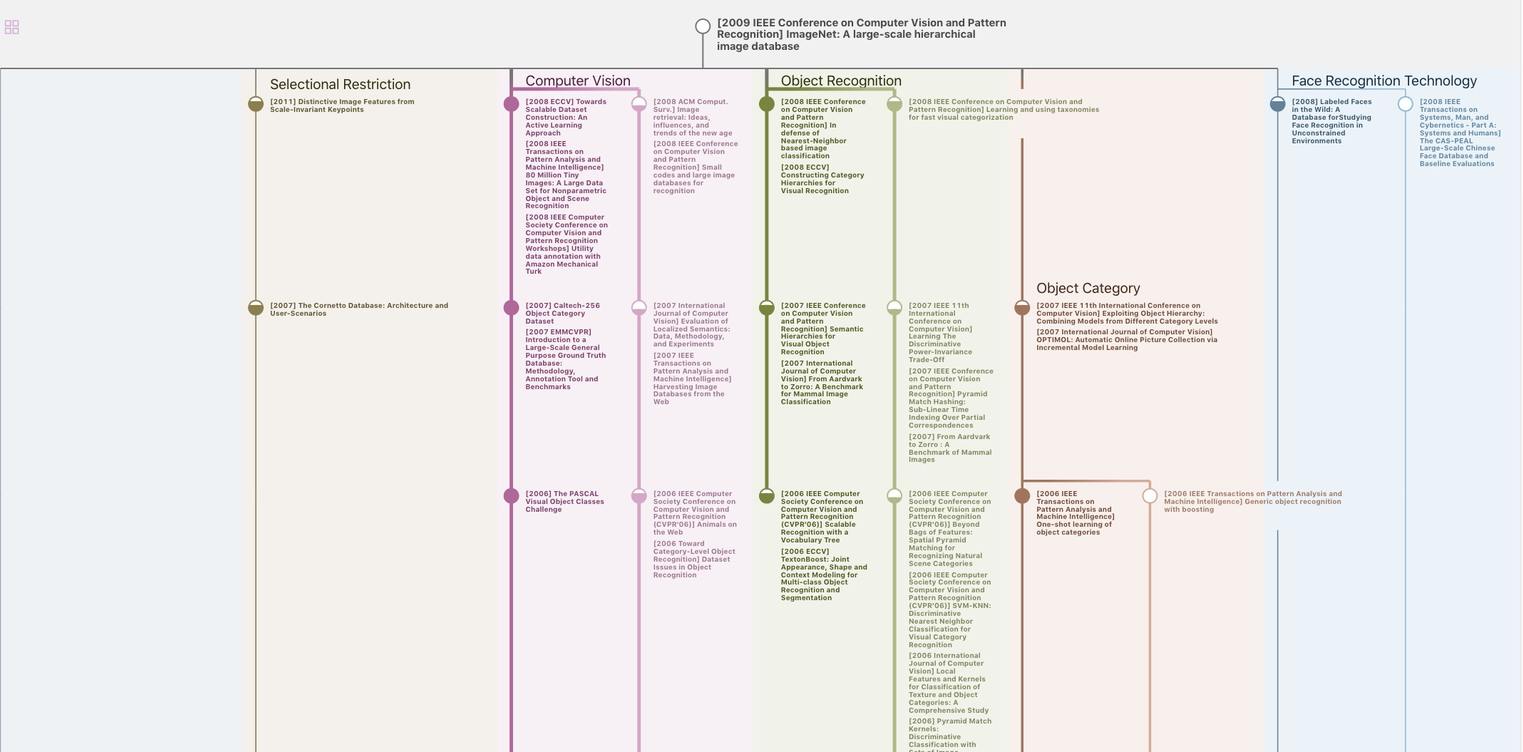
生成溯源树,研究论文发展脉络
Chat Paper
正在生成论文摘要