Differentially Private Covariance Estimation
ADVANCES IN NEURAL INFORMATION PROCESSING SYSTEMS 32 (NIPS 2019)(2019)
摘要
The task of privately estimating a covariance matrix is a popular one due to its applications to regression and PCA. While there are known methods for releasing private covariance matrices, these algorithms either achive only (epsilon,delta)-differential privacy or require very complicated sampling schemes, ultimately performing poorly in real data. In this work we propose a new E-differentially private algorithm for computing the covariance matrix of a dataset that addresses both of these limitations. We show that it has lower error than existing state-of-the-art approaches, both analytically and empirically. In addition, the algorithm is significantly less complicated than other methods and can be efficiently implemented with rejection sampling.
更多查看译文
关键词
rejection sampling
AI 理解论文
溯源树
样例
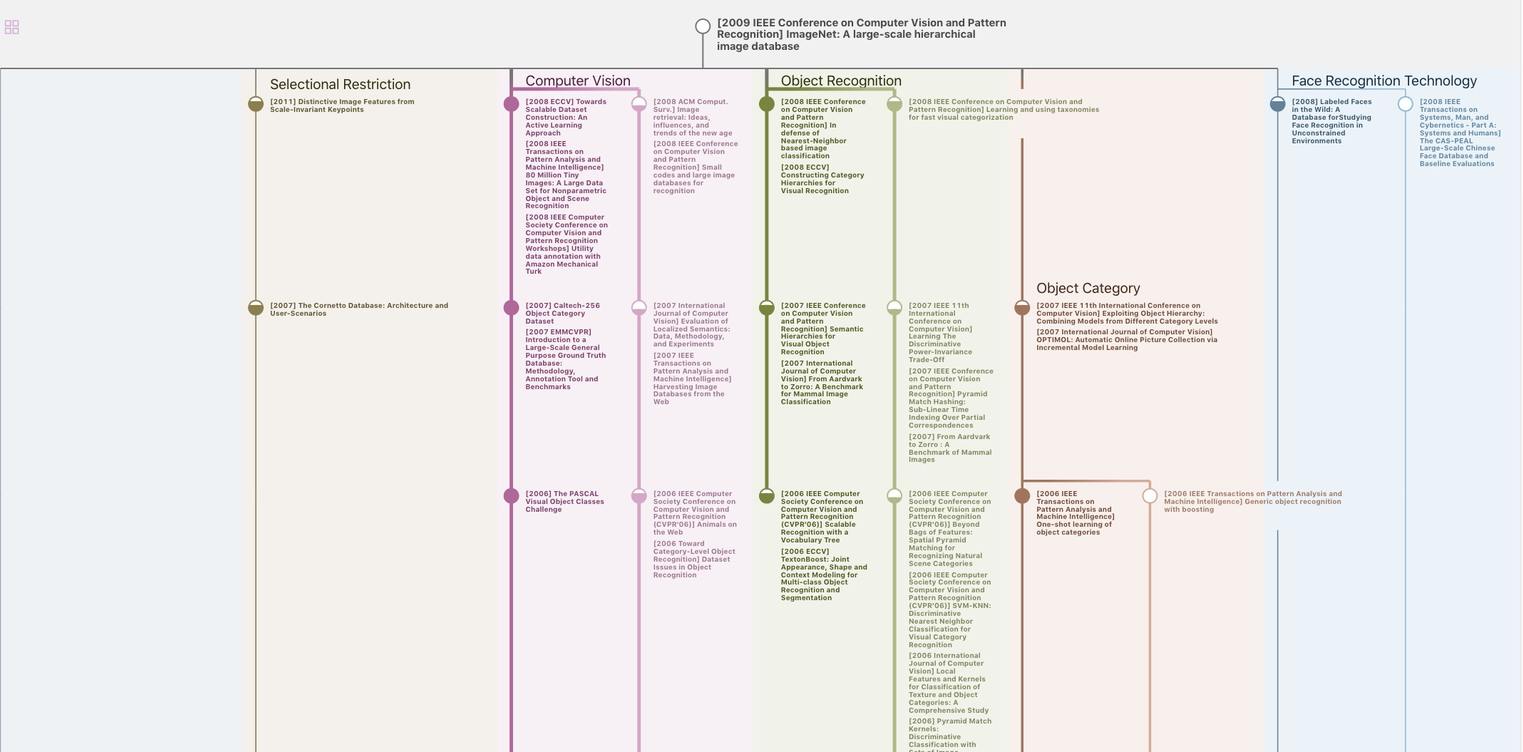
生成溯源树,研究论文发展脉络
Chat Paper
正在生成论文摘要