Single Image Colorization Via Modified Cyclegan
2019 IEEE INTERNATIONAL CONFERENCE ON IMAGE PROCESSING (ICIP)(2019)
摘要
In this paper, we focus on automatically colorizing single grayscale image without manual interventions. Most of existing methods tried to accurately restore unknown ground-truth colors and require paired training data for model optimization. However, the ideal restoration objective and strict training constraints limited their performance. Inspired by CycleGAN, we formulate the process of colorization as image-to-image translation and propose an effective color-CycleGAN solution. High-level semantic identity loss and low-level color loss are additionally suggested for model optimization. Our method allows using unpaired images for training and direct prediction in rgb color space, which makes training data collection much easier and more general. We train our model on randomly selected PASCAL VOC 2007 images. All ablation study on loss function and comparisons with state-of-the-art methods are performed on grayscale SUN data. The experiment results show that our improvements on training loss could achieve better content consistence and generate better reasonable colors with less artifacts. Moreover, due to the bidirectional nature of our model, our proposed method provides a by-product that gives an excellent alternative way on color image graying.
更多查看译文
关键词
Image colorization, Image translation, CycleGAN, Image processing, Unpaired training
AI 理解论文
溯源树
样例
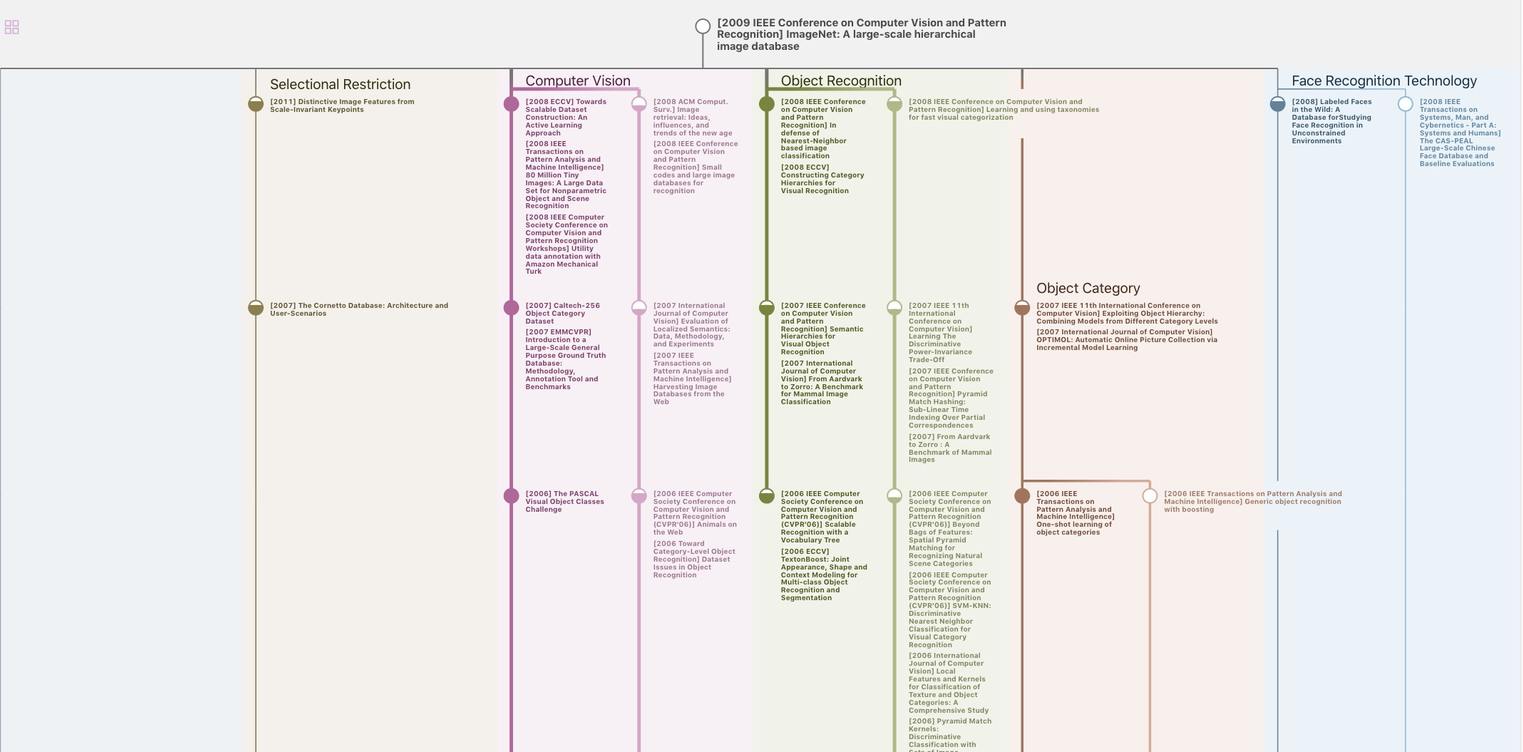
生成溯源树,研究论文发展脉络
Chat Paper
正在生成论文摘要