Classification-By-Components: Probabilistic Modeling Of Reasoning Over A Set Of Components
ADVANCES IN NEURAL INFORMATION PROCESSING SYSTEMS 32 (NIPS 2019)(2019)
摘要
Neural networks are state-of-the-art classification approaches but are generally difficult to interpret. This issue can be partly alleviated by constructing a precise decision process within the neural network. In this work, a network architecture, denoted as Classification-By-Components network (CBC), is proposed. It is restricted to follow an intuitive reasoning based decision process inspired by BIEDERMAN's recognition-by-components theory from cognitive psychology. The network is trained to learn and detect generic components that characterize objects. In parallel, a class-wise reasoning strategy based on these components is learned to solve the classification problem. In contrast to other work on reasoning, we propose three different types of reasoning: positive, negative, and indefinite. These three types together form a probability space to provide a probabilistic classifier. The decomposition of objects into generic components combined with the probabilistic reasoning provides by design a clear interpretation of the classification decision process. The evaluation of the approach on MNIST shows that CBCs are viable classifiers. Additionally, we demonstrate that the inherent interpretability offers a profound understanding of the classification behavior such that we can explain the success of an adversarial attack. The method's scalability is successfully tested using the IMAGENET dataset.
更多查看译文
关键词
neural networks,probabilistic reasoning,probability space,cognitive psychology
AI 理解论文
溯源树
样例
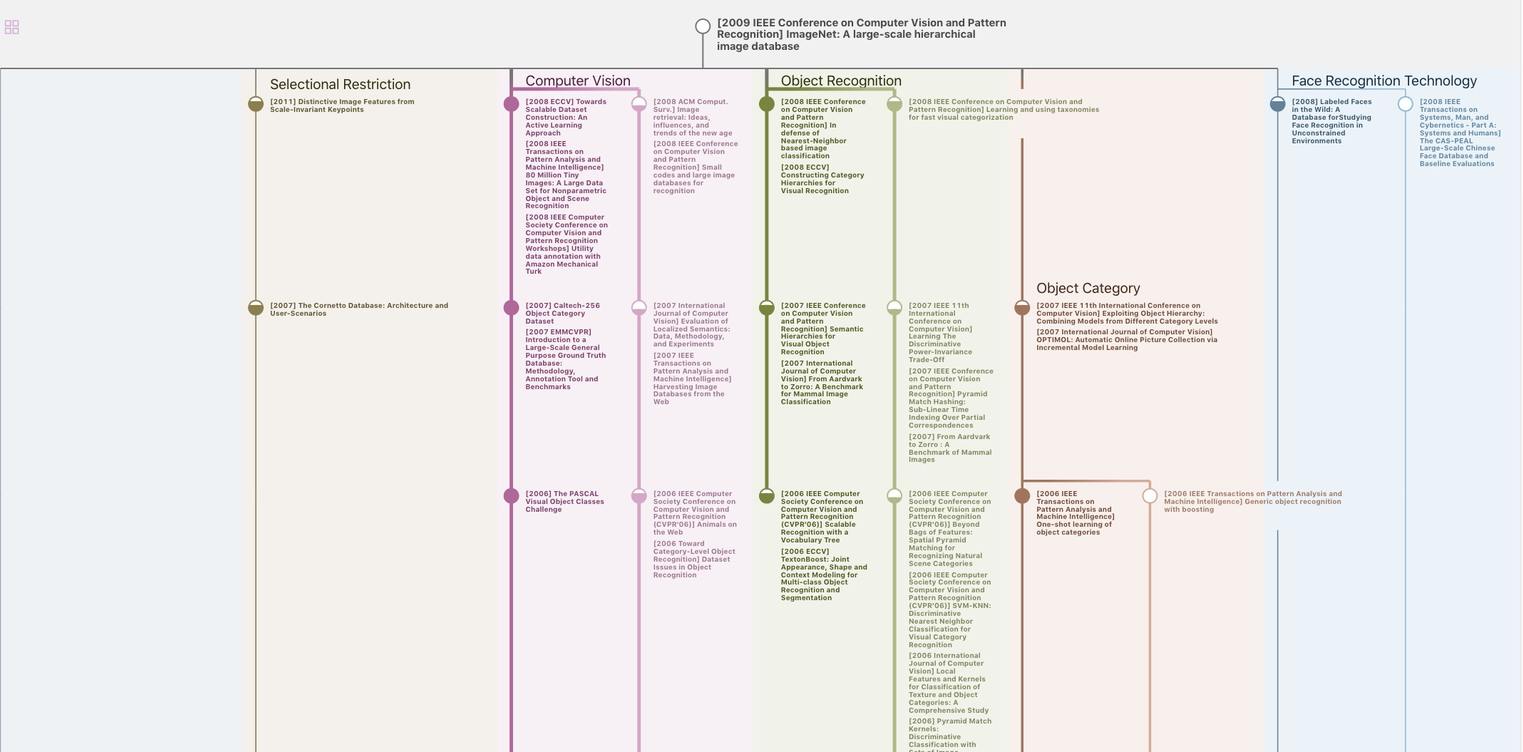
生成溯源树,研究论文发展脉络
Chat Paper
正在生成论文摘要